Toward Explainable 3D Grounded Visual Question Answering: A New Benchmark and Strong Baseline
arxiv(2023)
摘要
Recently, 3D vision-and-language tasks have attracted increasing research interest. Compared to other vision-and-language tasks, the 3D visual question answering (VQA) task is less exploited and is more susceptible to language priors and co-reference ambiguity. Meanwhile, a couple of recently proposed 3D VQA datasets do not well support 3D VQA task due to their limited scale and annotation methods. In this work, we formally define and address a 3D grounded question answering (GQA) task by collecting a new 3D VQA dataset, referred to as flexible and explainable 3D GQA (FE-3DGQA), with diverse and relatively free-form question-answer pairs, as well as dense and completely grounded bounding box annotations. To achieve more explainable answers, we label the objects appeared in the complex QA pairs with different semantic types, including answer-grounded objects (both appeared and not appeared in the questions), and contextual objects for answer-grounded objects. We also propose a new 3D VQA framework to effectively predict the completely visually grounded and explainable answer. Extensive experiments verify that our newly collected benchmark datasets can be effectively used to evaluate various 3D VQA methods from different aspects and our newly proposed framework also achieves the state-of-the-art performance on the new benchmark dataset. The datasets and the source code are available via https://github.com/zlccccc/3DVL_Codebase.
更多查看译文
关键词
Three-dimensional displays,Task analysis,Visualization,Annotations,Point cloud compression,Solid modeling,Question answering (information retrieval),Grounded visual question answering,vision and language on 3D scenes
AI 理解论文
溯源树
样例
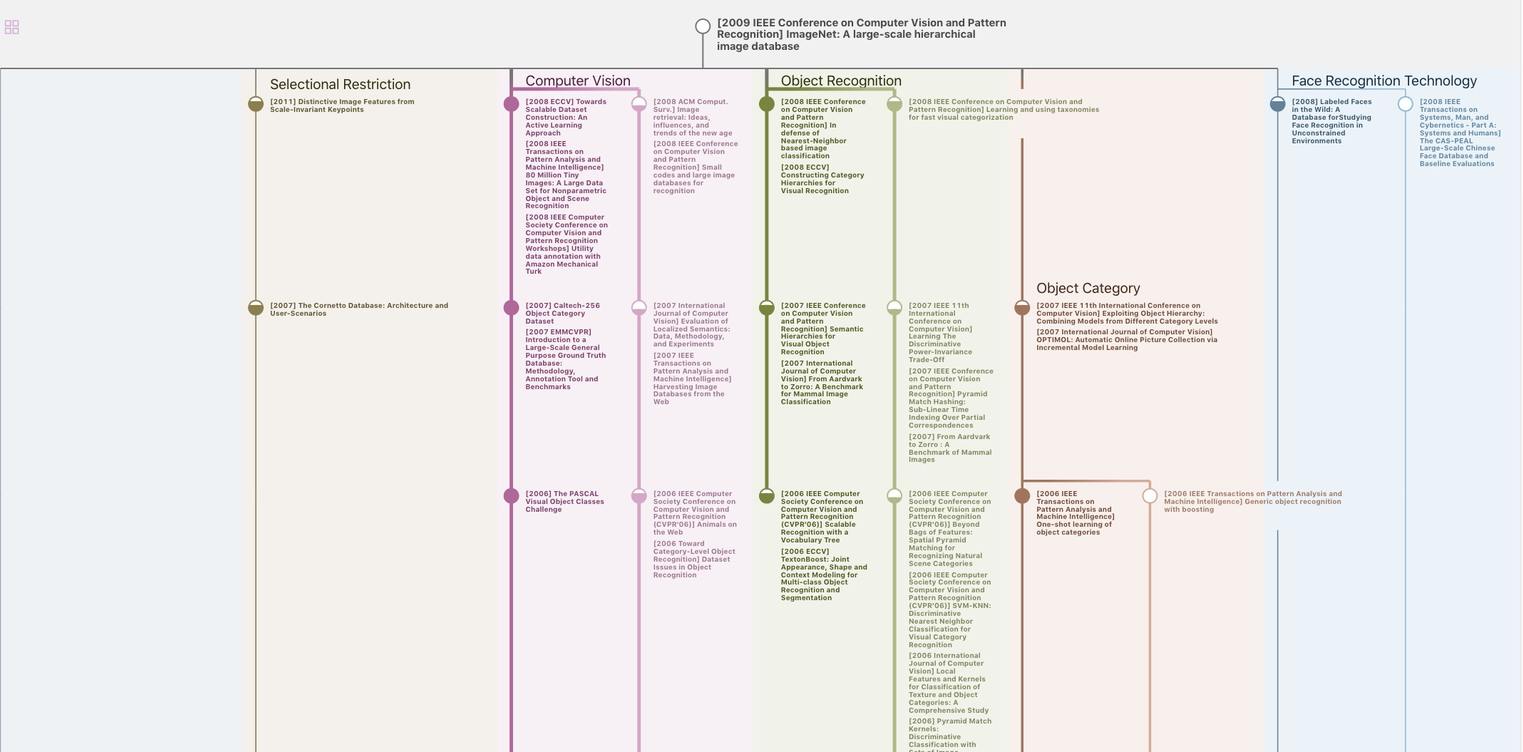
生成溯源树,研究论文发展脉络
Chat Paper
正在生成论文摘要