Large-Scale LiDAR Consistent Mapping Using Hierarchical LiDAR Bundle Adjustment
IEEE Robotics and Automation Letters(2023)
摘要
Reconstructing an accurate and consistent large-scale LiDAR point cloud map is crucial for robotics applications. The existing solution, pose graph optimization, though it is time-efficient, does not directly optimize the mapping consistency. LiDAR bundle adjustment (BA) has been recently proposed to resolve this issue; however, it is too time-consuming on large-scale maps. To mitigate this problem, this paper presents a globally consistent and efficient mapping method suitable for large-scale maps. Our proposed work consists of a bottom-up hierarchical BA and a top-down pose graph optimization, which combines the advantages of both methods. With the hierarchical design, we solve multiple BA problems with a much smaller Hessian matrix size than the original BA; with the pose graph optimization, we smoothly and efficiently update the LiDAR poses. The effectiveness and robustness of our proposed approach have been validated on multiple spatially and timely large-scale public spinning LiDAR datasets, i.e., KITTI, MulRan and Newer College, and self-collected solid-state LiDAR datasets under structured and unstructured scenes. With proper setups, we demonstrate our work could generate a globally consistent map with around 12
$\%$
of the sequence time.
更多查看译文
关键词
Mapping,SLAM,localization
AI 理解论文
溯源树
样例
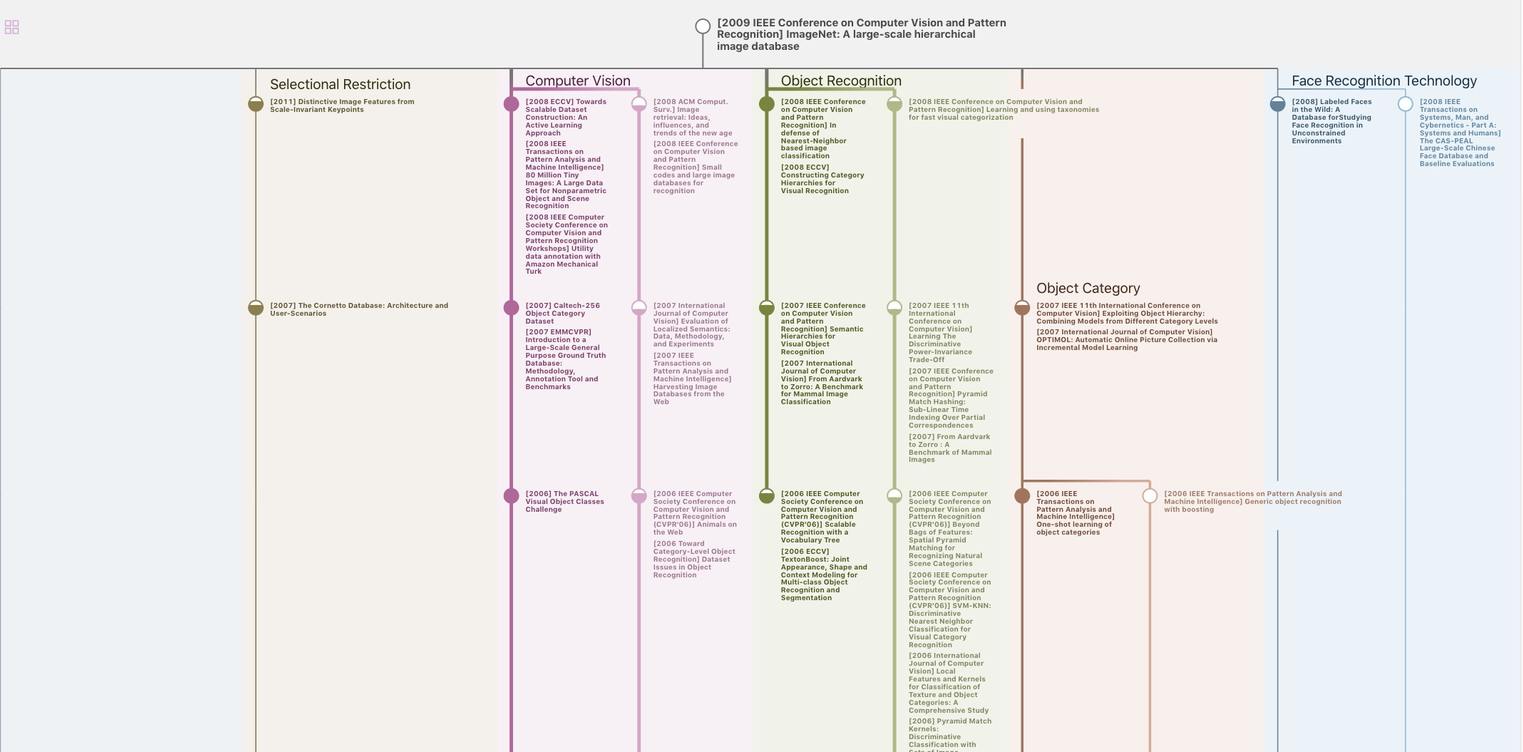
生成溯源树,研究论文发展脉络
Chat Paper
正在生成论文摘要