Effectiveness of Machine Learning in Assessing the Diagnostic Quality of Bitewing Radiographs
Applied Sciences(2022)
摘要
Background: Identifying the diagnostic value of bitewing radiographs (BW) is highly dependent on the operator’s knowledge and experience. The aim of this study is to assess the effectiveness of machine learning (ML) to classify the BW according to their diagnostic quality. Methods: 864 BW radiographs from records of 100 patients presented at King Abdulaziz University Dental Hospital, Jeddah, Saudi Arabia were assessed. The radiographic errors in representing proximal contact areas (n = 1951) were categorized into diagnostic and non-diagnostic. Labeling and training of the BW were done using Roboflow. Data were divided into validation, training, and testing sets to train the pre-trained model Efficientdet-d0 using TensorFlow. The model’s performance was assessed by calculating recall, precision, F1 score, and log loss value. Results: The model excelled at detecting “overlap within enamel” and “overlap within restoration (clear margins) with F1 score of 0.89 and 0.76, respectively. The overall system errors made by the built model showed a log loss value of 0.15 indicating high accuracy of the model. Conclusion: The model is a “proof of concept” for the effectiveness of ML in diagnosing the quality of the BW radiographs based on the contact areas. More dataset specification and optimization are needed to overcome the class imbalance.
更多查看译文
关键词
artificial intelligence, bitewing radiographs, contact areas, machine learning, radiographic errors
AI 理解论文
溯源树
样例
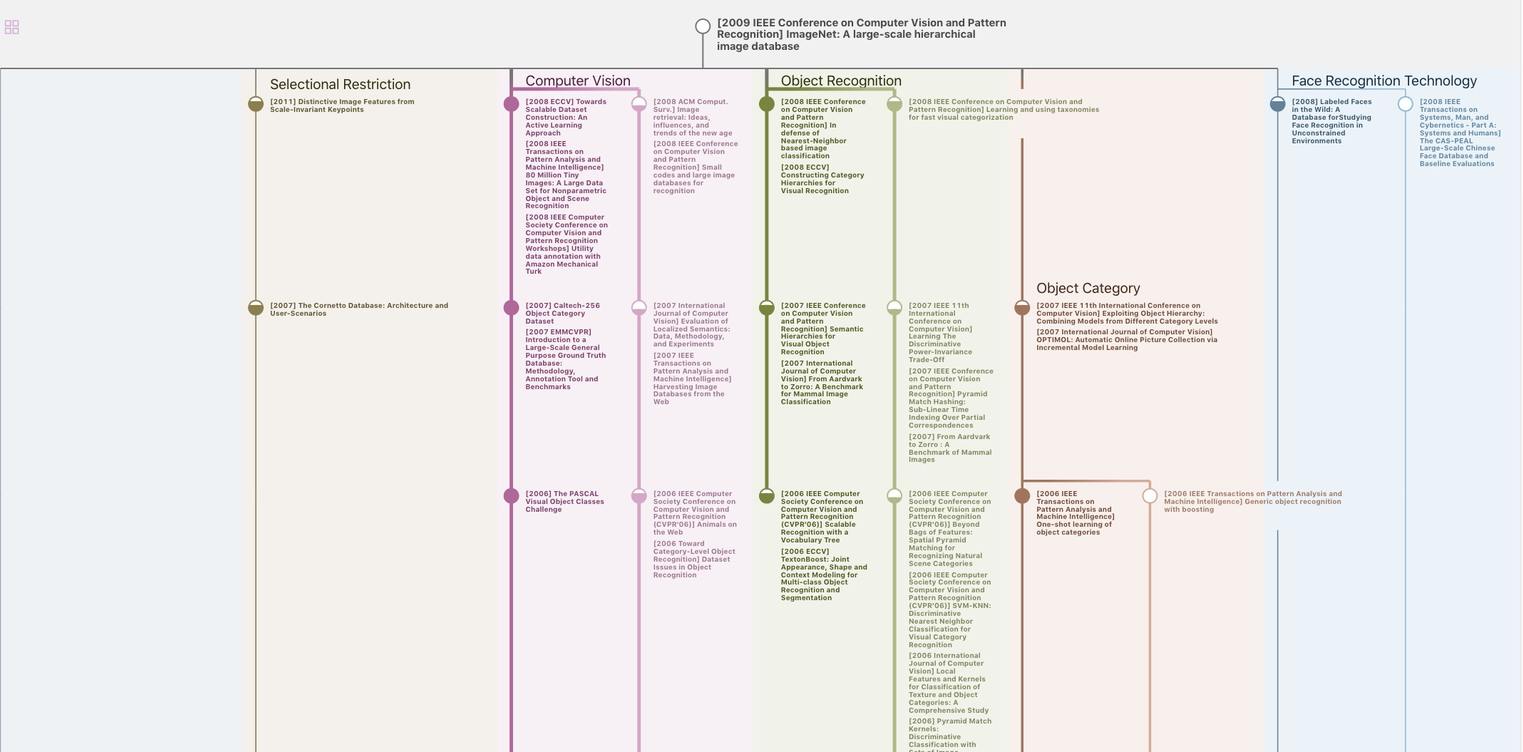
生成溯源树,研究论文发展脉络
Chat Paper
正在生成论文摘要