Adaptive-SpikeNet: Event-based Optical Flow Estimation using Spiking Neural Networks with Learnable Neuronal Dynamics
2023 IEEE INTERNATIONAL CONFERENCE ON ROBOTICS AND AUTOMATION, ICRA(2023)
摘要
Event-based cameras have recently shown great potential for high-speed motion estimation owing to their ability to capture temporally rich information asynchronously. Spiking Neural Networks (SNNs), with their neuro-inspired event-driven processing can efficiently handle such asynchronous data, while neuron models such as the leaky-integrate and fire (LIF) can keep track of the quintessential timing information contained in the inputs. SNNs achieve this by maintaining a dynamic state in the neuron memory, retaining important information while forgetting redundant data over time. Thus, we posit that SNNs would allow for better performance on sequential regression tasks compared to similarly sized Analog Neural Networks (ANNs). However, deep SNNs are difficult to train due to vanishing spikes at later layers. To that effect, we propose an adaptive fully-spiking framework with learnable neuronal dynamics to alleviate the spike vanishing problem. We utilize surrogate gradient-based backpropagation through time (BPTT) to train our deep SNNs from scratch. We validate our approach for the task of optical flow estimation on the Multi-Vehicle Stereo Event-Camera (MVSEC) dataset and the DSEC-Flow dataset. Our experiments on these datasets show an average reduction of similar to 13% in average endpoint error (AEE) compared to state-of-the-art ANNs. We also explore several down-scaled models and observe that our SNN models consistently outperform similarly sized ANNs offering similar to 10%-16% lower AEE. These results demonstrate the importance of SNNs for smaller models and their suitability at the edge. In terms of efficiency, our SNNs offer substantial savings in network parameters (similar to 48.3x) and computational energy (similar to 10.2x) while attaining similar to 10% lower EPE compared to the state-of-the-art ANN implementations.
更多查看译文
AI 理解论文
溯源树
样例
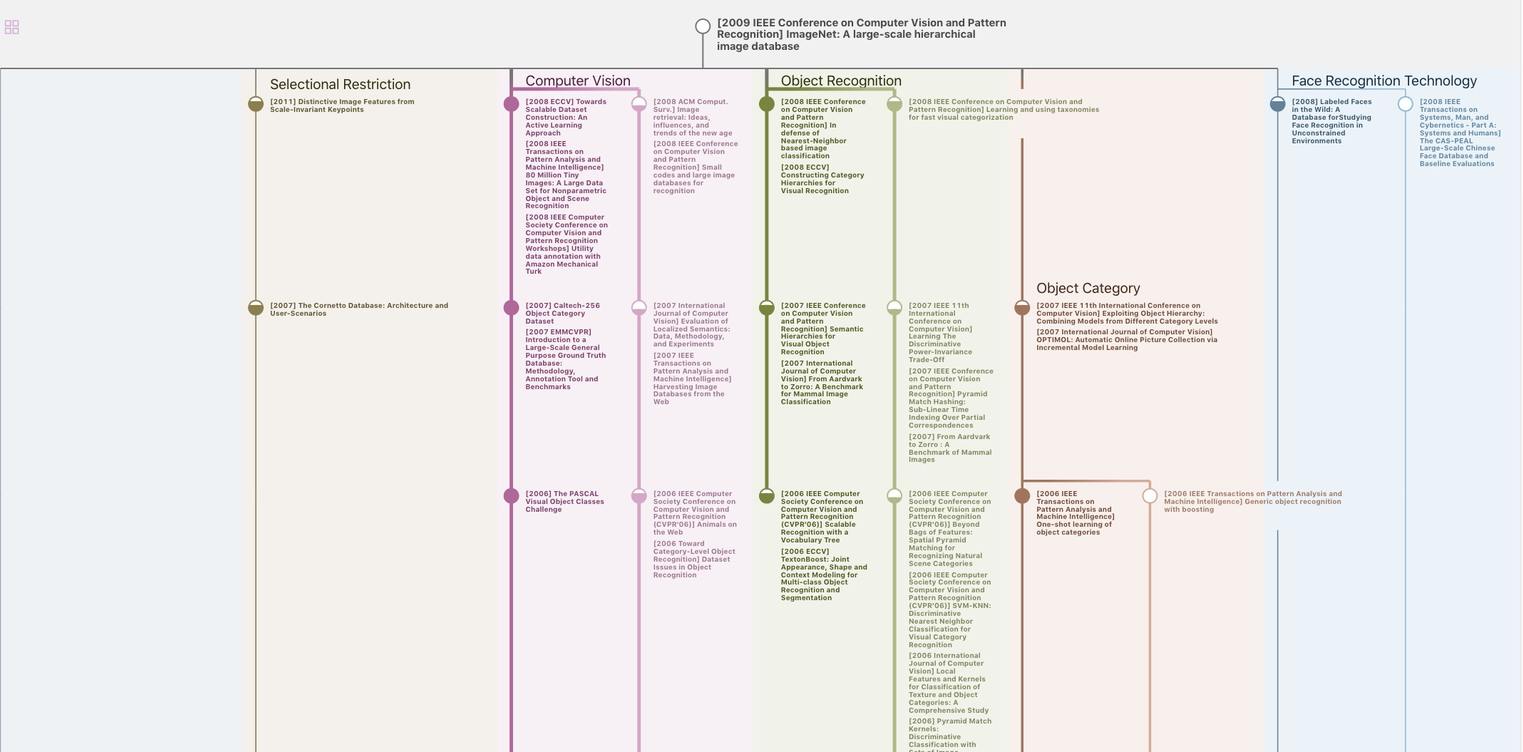
生成溯源树,研究论文发展脉络
Chat Paper
正在生成论文摘要