Image-to-Image Translation for Autonomous Driving from Coarsely-Aligned Image Pairs
arxiv(2023)
摘要
A self-driving car must be able to reliably handle adverse weather conditions (e.g., snowy) to operate safely. In this paper, we investigate the idea of turning sensor inputs (i.e., images) captured in an adverse condition into a benign one (i.e., sunny), upon which the downstream tasks (e.g., semantic segmentation) can attain high accuracy. Prior work primarily formulates this as an unpaired image-to-image translation problem due to the lack of paired images captured under the exact same camera poses and semantic layouts. While perfectly-aligned images are not available, one can easily obtain coarsely-paired images. For instance, many people drive the same routes daily in both good and adverse weather; thus, images captured at close-by GPS locations can form a pair. Though data from repeated traversals are unlikely to capture the same foreground objects, we posit that they provide rich contextual information to supervise the image translation model. To this end, we propose a novel training objective leveraging coarsely-aligned image pairs. We show that our coarsely-aligned training scheme leads to a better image translation quality and improved downstream tasks, such as semantic segmentation, monocular depth estimation, and visual localization.
更多查看译文
关键词
adverse condition,autonomous driving,coarsely-paired images,downstream tasks,good weather,handle adverse weather conditions,image translation model,image translation quality,perfectly-aligned images,self-driving car,semantic layouts,semantic segmentation,training objective leveraging coarsely-aligned image pairs,unpaired image-to-image translation problem
AI 理解论文
溯源树
样例
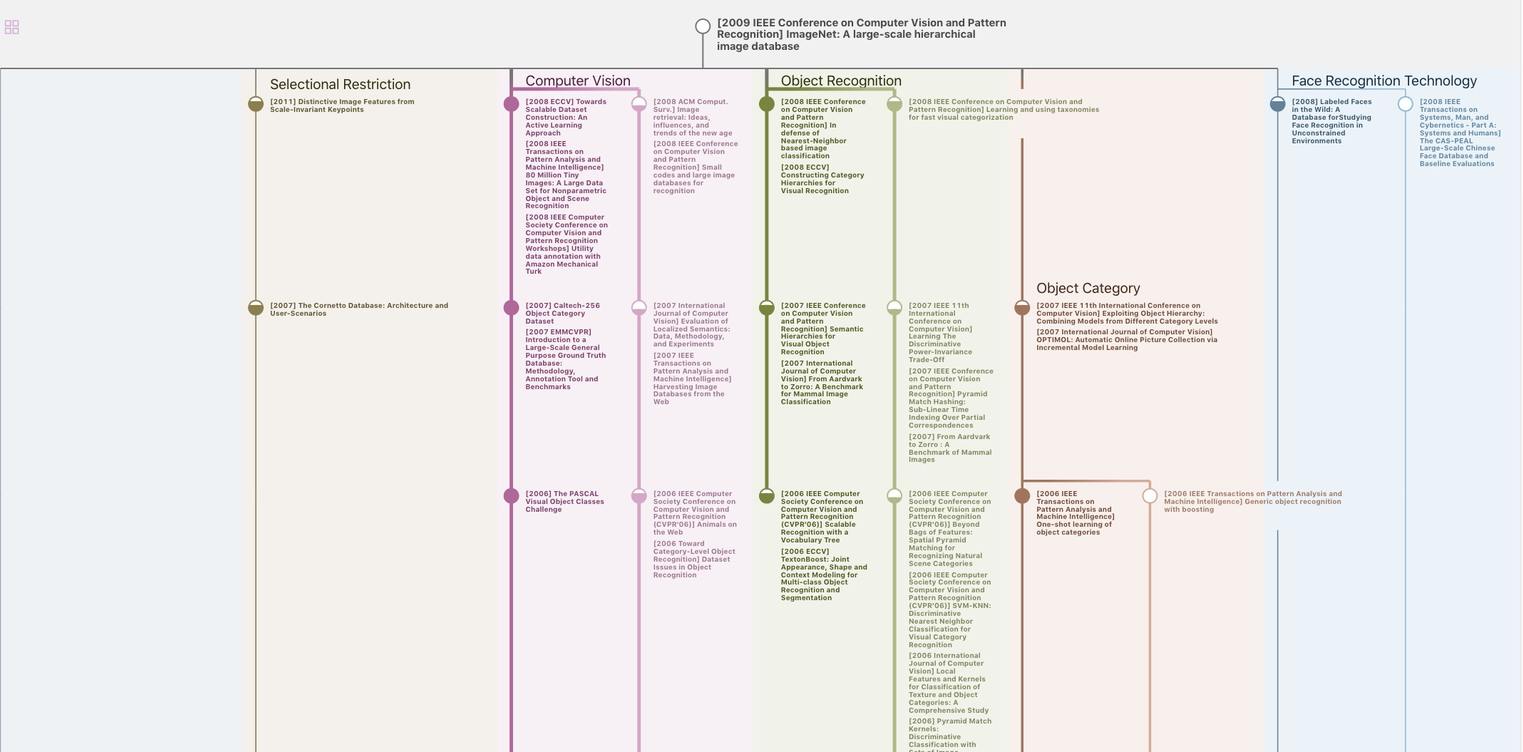
生成溯源树,研究论文发展脉络
Chat Paper
正在生成论文摘要