Reactive Anticipatory Robot Skills with Memory
arxiv(2022)
摘要
Optimal control in robotics has been increasingly popular in recent years and has been applied in many applications involving complex dynamical systems. Closed-loop optimal control strategies include model predictive control (MPC) and time-varying linear controllers optimized through iLQR. However, such feedback controllers rely on the information of the current state, limiting the range of robotic applications where the robot needs to remember what it has done before to act and plan accordingly. The recently proposed system level synthesis (SLS) framework circumvents this limitation via a richer controller structure with memory. In this work, we propose to optimally design reactive anticipatory robot skills with memory by extending SLS to tracking problems involving nonlinear systems and nonquadratic cost functions. We showcase our method with two scenarios exploiting task precisions and object affordances in pick-and-place tasks in a simulated and a real environment with a 7-axis Franka Emika robot.
更多查看译文
关键词
Optimal control, Feedback control with memory, Robot control
AI 理解论文
溯源树
样例
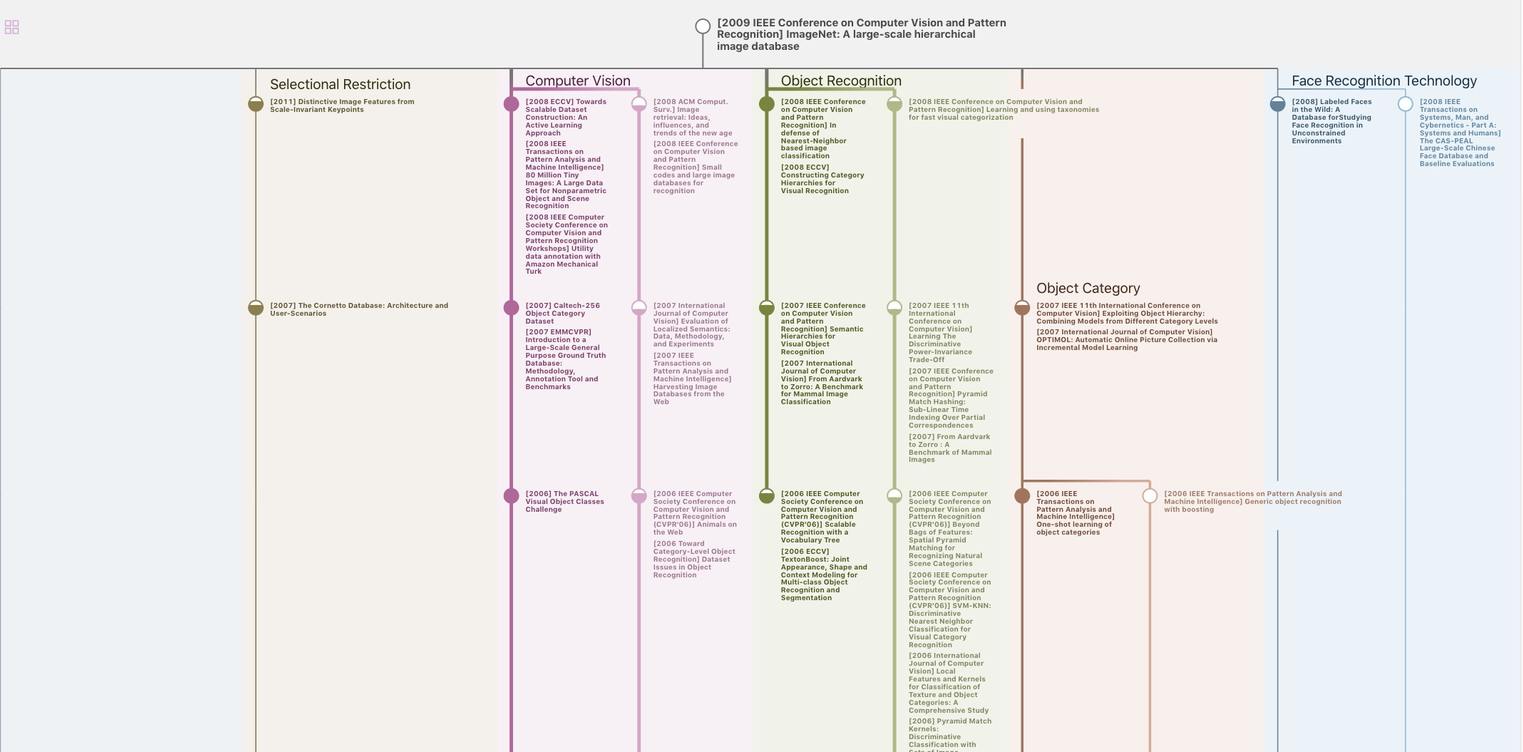
生成溯源树,研究论文发展脉络
Chat Paper
正在生成论文摘要