FIAT: Fine-grained Information Audit for Trustless Transborder Data Flow
arxiv(2023)
摘要
Auditing the information leakage of latent sensitive features during the transborder data flow has attracted sufficient attention from global digital regulators. However, there is missing a technical approach for the audit practice due to two technical challenges. Firstly, there is a lack of theory and tools for measuring the information of sensitive latent features in a dataset. Secondly, the transborder data flow involves multi-stakeholders with diverse interests, which means the audit must be trustless. Despite the tremendous efforts in protecting data privacy, an important issue that has long been neglected is that the transmitted data in data flows can leak other regulated information that is not explicitly contained in the data, leading to unaware information leakage risks. To unveil such risks trustfully before the actual data transfer, we propose FIAT, a Fine-grained Information Audit system for Trustless transborder data flow. In FIAT, we use a learning approach to quantify the amount of information leakage, while the technologies of zero-knowledge proof and smart contracts are applied to provide trustworthy and privacy-preserving auditing results. Experiments show that large information leakage can boost the predictability of uninvolved information using simple machine-learning models, revealing the importance of information auditing. Further performance benchmarking also validates the efficiency and scalability of the FIAT auditing system.
更多查看译文
关键词
information audit,data,flow,fine-grained
AI 理解论文
溯源树
样例
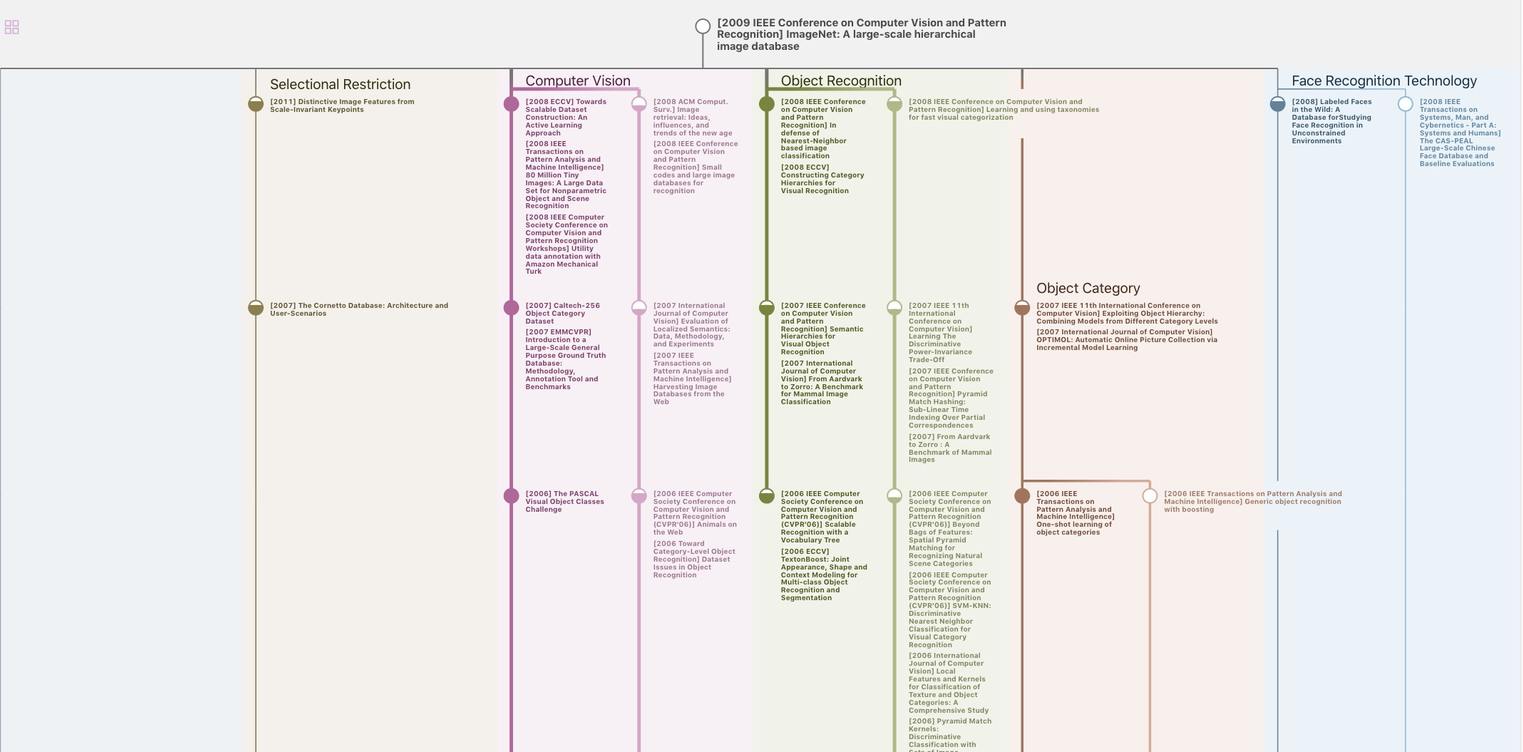
生成溯源树,研究论文发展脉络
Chat Paper
正在生成论文摘要