A Robust and Explainable Data-Driven Anomaly Detection Approach For Power Electronics
2022 IEEE International Conference on Communications, Control, and Computing Technologies for Smart Grids (SmartGridComm)(2022)
摘要
Timely and accurate detection of anomalies in power electronics is becoming increasingly critical for maintaining complex production systems. Robust and explainable strategies help decrease system downtime and preempt or mitigate infrastructure cyberattacks. This work begins by explaining the types of uncertainty present in current datasets and machine learning algorithm outputs. Three techniques for combating these uncertainties are then introduced and analyzed. We further present two anomaly detection and classification approaches, namely the Matrix Profile algorithm and anomaly transformer, which are applied in the context of a power electronic converter dataset. Specifically, the Matrix Profile algorithm is shown to be well suited as a generalizable approach for detecting real-time anomalies in streaming time-series data. The STUMPY python library implementation of the iterative Matrix Profile is used for the creation of the detector. A series of custom filters is created and added to the detector to tune its sensitivity, recall, and detection accuracy. Our numerical results show that, with simple parameter tuning, the detector provides high accuracy and performance in a variety of fault scenarios.
更多查看译文
关键词
Industrial Internet of Things,anomaly detection,fault classification,cyber-physical system,visualization
AI 理解论文
溯源树
样例
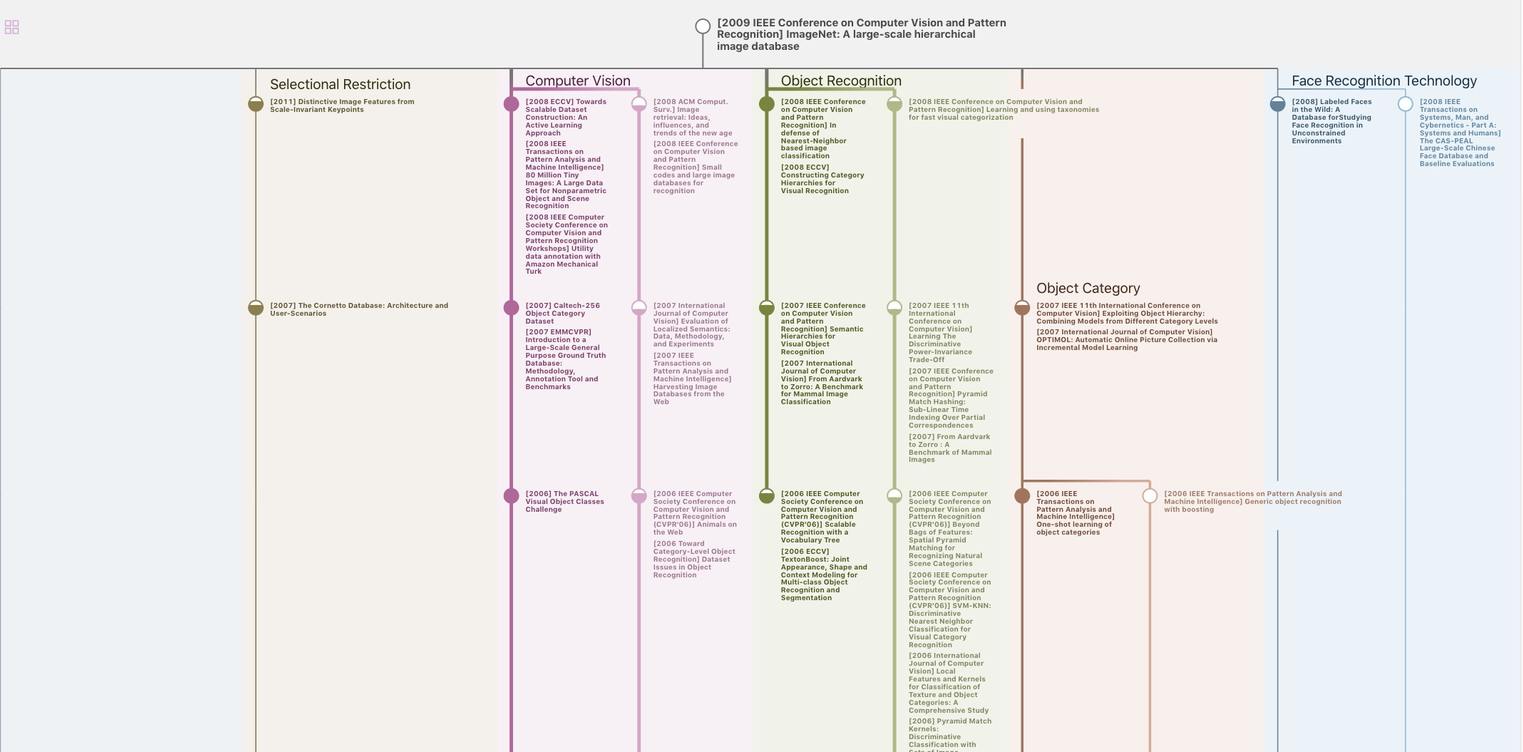
生成溯源树,研究论文发展脉络
Chat Paper
正在生成论文摘要