Deep Ensemble Learning for the Automatic Detection of Pneumoconiosis in Coal Worker's Chest X-ray Radiography
JOURNAL OF CLINICAL MEDICINE(2022)
摘要
Globally, coal remains one of the natural resources that provide power to the world. Thousands of people are involved in coal collection, processing, and transportation. Particulate coal dust is produced during these processes, which can crush the lung structure of workers and cause pneumoconiosis. There is no automated system for detecting and monitoring diseases in coal miners, except for specialist radiologists. This paper proposes ensemble learning techniques for detecting pneumoconiosis disease in chest X-ray radiographs (CXRs) using multiple deep learning models. Three ensemble learning techniques (simple averaging, multi-weighted averaging, and majority voting (MVOT)) were proposed to investigate performances using randomised cross-folds and leave-one-out cross-validations datasets. Five statistical measurements were used to compare the outcomes of the three investigations on the proposed integrated approach with state-of-the-art approaches from the literature for the same dataset. In the second investigation, the statistical combination was marginally enhanced in the ensemble of multi-weighted averaging on a robust model, CheXNet. However, in the third investigation, the same model elevated accuracies from 87.80 to 90.2%. The investigated results helped us identify a robust deep learning model and ensemble framework that outperformed others, achieving an accuracy of 91.50% in the automated detection of pneumoconiosis.
更多查看译文
关键词
ensemble learning, pneumoconiosis, transfer learning, deep learning, chest X-ray radiographs, cross validation, weighted-averaging, majority voting, LOOCV, CheXNet
AI 理解论文
溯源树
样例
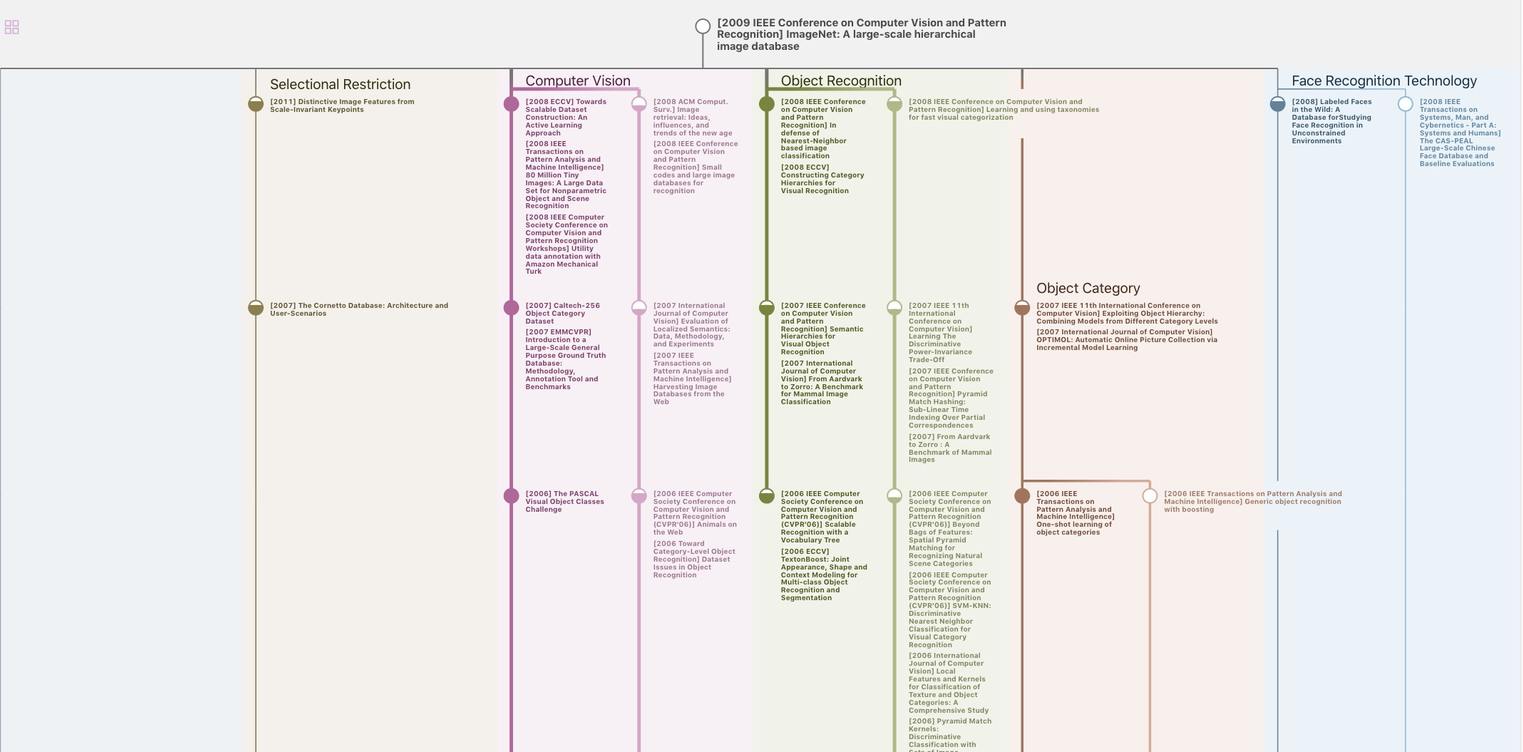
生成溯源树,研究论文发展脉络
Chat Paper
正在生成论文摘要