Multimodal Fake-News Recognition Using Ensemble of Deep Learners
ENTROPY(2022)
摘要
Social networks have drastically changed how people obtain information. News in social networks is accompanied by images and videos and thus receives more attention from readers as opposed to traditional sources. Unfortunately, fake-news publishers often misuse these advantages to spread false information rapidly. Therefore, the early detection of fake news is crucial. The best way to address this issue is to design an automatic detector based on fake-news content. Thus far, many fake-news recognition systems, including both traditional machine learning and deep learning models, have been proposed. Given that manual feature-extraction methods are very time-consuming, deep learning methods are the preferred tools. This study aimed to enhance the performance of existing approaches by utilizing an ensemble of deep learners based on attention mechanisms. To a great extent, the success of an ensemble model depends on the variety of its learners. To this end, we propose a novel loss function that enforces each learner to attend to different parts of news content on the one hand and obtain good classification accuracy on the other hand. Also, the learners are built on a common deep-feature extractor and only differ in their attention modules. As a result, the number of parameters is reduced efficiently and the overfitting problem is addressed. We conducted several experiments on some widely used fake-news detection datasets. The results confirm that the proposed method consistently surpasses the existing peer methods.
更多查看译文
关键词
fake-news recognition, ensemble of deep learners, attention mechanism, multimodal data
AI 理解论文
溯源树
样例
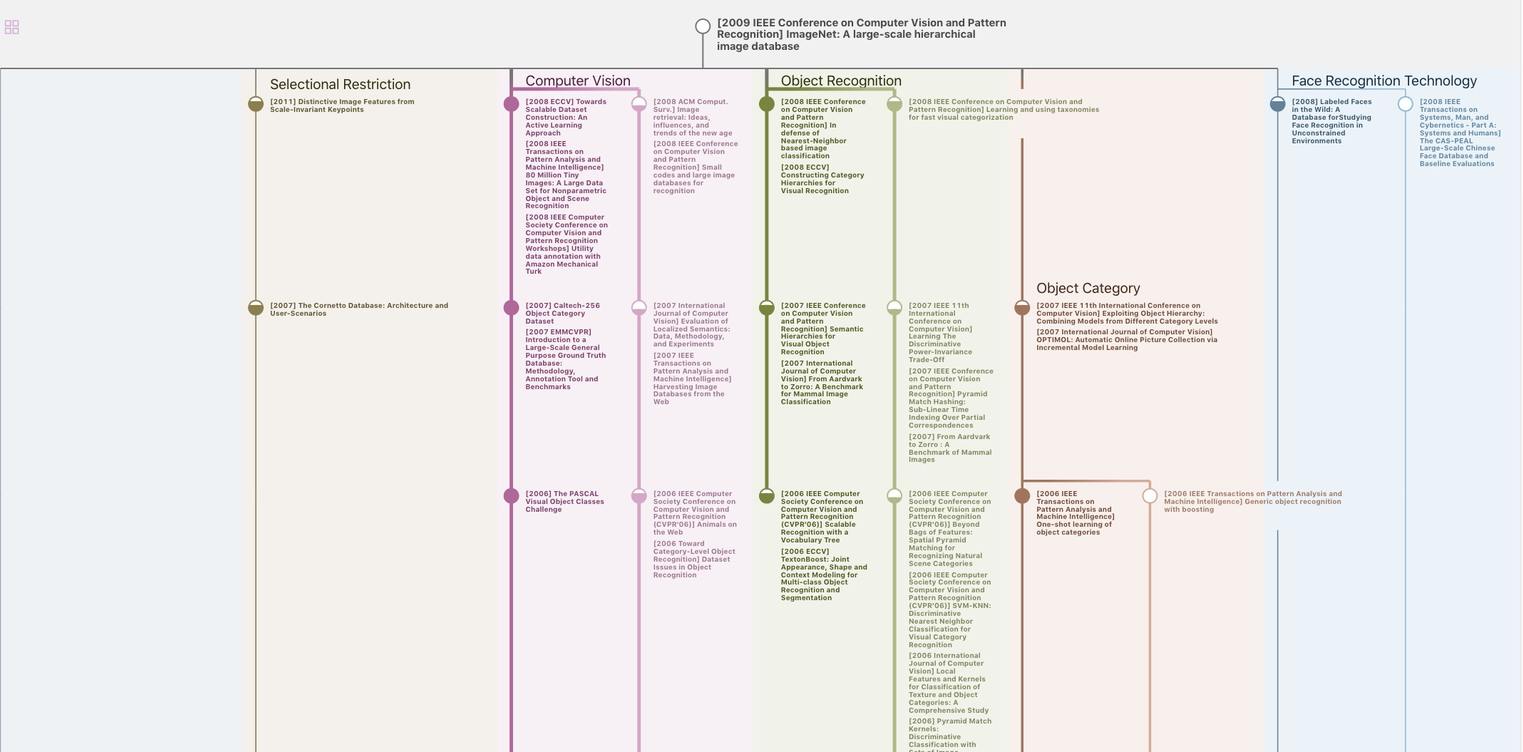
生成溯源树,研究论文发展脉络
Chat Paper
正在生成论文摘要