Clinical Decision Support for Axillary Lymph Node Staging in Newly Diagnosed Breast Cancer Patients Based on 18F-FDG PET/MRI and Machine Learning
Journal of nuclear medicine : official publication, Society of Nuclear Medicine(2023)
摘要
In addition to its high prognostic value, the involvement of axillary lymph nodes in breast cancer patients also plays an important role in therapy planning. Therefore, an imaging modality that can determine nodal status with high accuracy in patients with primary breast cancer is desirable. Our purpose was to investigate whether, in newly diag-nosed breast cancer patients, machine-learning prediction models based on simple assessable imaging features on MRI or PET/MRI are able to determine nodal status with performance comparable to that of experienced radiologists; whether such models can be adjusted to achieve low rates of false-negatives such that invasive procedures might potentially be omitted; and whether a clinical framework for decision support based on simple imaging features can be derived from these models. Methods: Between August 2017 and September 2020, 303 participants from 3 centers prospectively underwent dedi-cated whole-body 18F-FDG PET/MRI. Imaging datasets were evalu-ated for axillary lymph node metastases based on morphologic and metabolic features. Predictive models were developed for MRI and PET/MRI separately using random forest classifiers on data from 2 centers and were tested on data from the third center. Results: The diagnostic accuracy for MRI features was 87.5% both for radiologists and for the machine-learning algorithm. For PET/MRI, the diagnostic accuracy was 89.3% for the radiologists and 91.2% for the machine -learning algorithm, with no significant differences in diagnostic perfor-mance between radiologists and the machine-learning algorithm for MRI (P = 0.671) or PET/MRI (P = 0.683). The most important lymph node feature was tracer uptake, followed by lymph node size. With an adjusted threshold, a sensitivity of 96.2% was achieved by the ran-dom forest classifier, whereas specificity, positive predictive value, negative predictive value, and accuracy were 68.2%, 78.1%, 93.8%, and 83.3%, respectively. A decision tree based on 3 simple imaging features could be established for MRI and PET/MRI. Conclusion: Applying a high-sensitivity threshold to the random forest results might potentially avoid invasive procedures such as sentinel lymph node biopsy in 68.2% of the patients.
更多查看译文
关键词
MRI,Oncology: Breast,Other,PET/MRI,breast cancer,lymph node metastases,machine learning
AI 理解论文
溯源树
样例
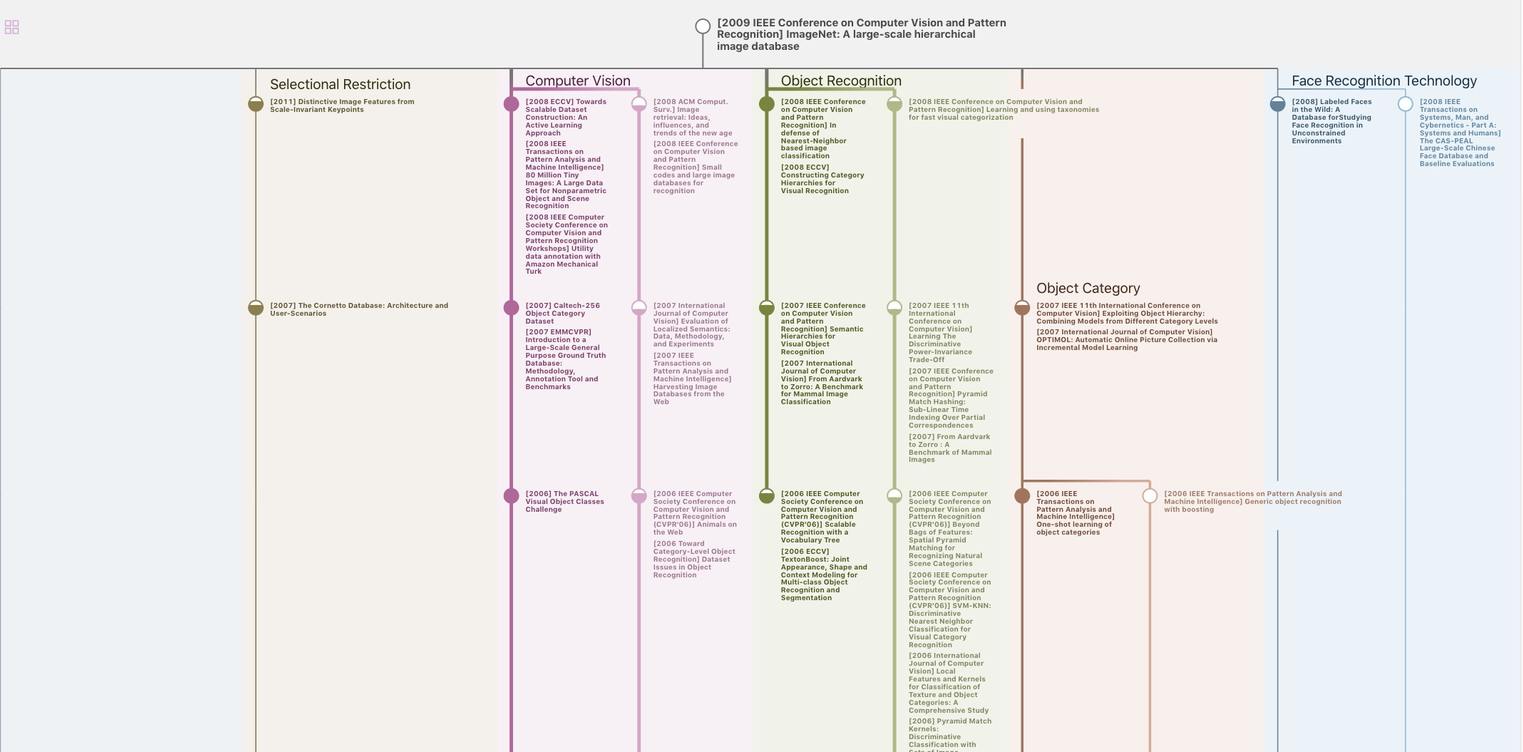
生成溯源树,研究论文发展脉络
Chat Paper
正在生成论文摘要