Beyond Voxel Prediction Uncertainty: Identifying Brain Lesions You Can Trust
INTERPRETABILITY OF MACHINE INTELLIGENCE IN MEDICAL IMAGE COMPUTING, IMIMIC 2022(2022)
摘要
Deep neural networks have become the gold-standard approach for the automated segmentation of 3D medical images. Their full acceptance by clinicians remains however hampered by the lack of intelligible uncertainty assessment of the provided results. Most approaches to quantify their uncertainty, such as the popular Monte Carlo dropout, restrict to some measure of uncertainty in prediction at the voxel level. In addition not to be clearly related to genuine medical uncertainty, this is not clinically satisfying as most objects of interest (e.g. brain lesions) are made of groups of voxels whose overall relevance may not simply reduce to the sum or mean of their individual uncertainties. In this work, we propose to go beyond voxel-wise assessment using an innovative Graph Neural Network approach, trained from the outputs of a Monte Carlo dropout model. This network allows the fusion of three estimators of voxel uncertainty: entropy, variance, and model's confidence; and can be applied to any lesion, regardless of its shape or size. We demonstrate the superiority of our approach for uncertainty estimate on a task of Multiple Sclerosis lesions segmentation.
更多查看译文
关键词
MS lesion, Detection, Deep learning, Interpretabilty, Prediction
AI 理解论文
溯源树
样例
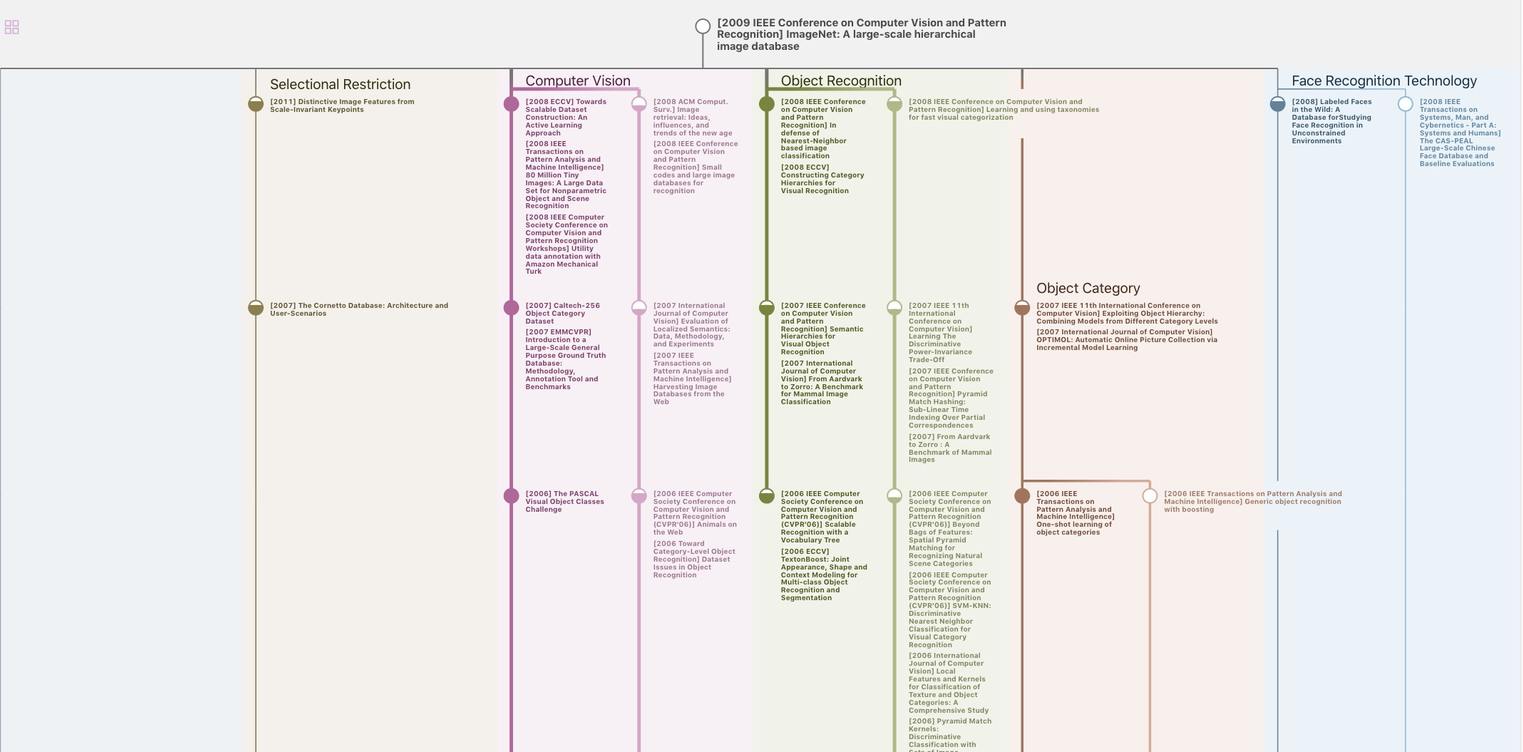
生成溯源树,研究论文发展脉络
Chat Paper
正在生成论文摘要