How Does It Feel? Self-Supervised Costmap Learning for Off-Road Vehicle Traversability
2023 IEEE INTERNATIONAL CONFERENCE ON ROBOTICS AND AUTOMATION, ICRA(2023)
摘要
Estimating terrain traversability in off-road environments requires reasoning about complex interaction dynamics between the robot and these terrains. However, it is challenging to create informative labels to learn a model in a supervised manner for these interactions. We propose a method that learns to predict traversability costmaps by combining exteroceptive environmental information with proprioceptive terrain interaction feedback in a self-supervised manner. Additionally, we propose a novel way of incorporating robot velocity into the costmap prediction pipeline. We validate our method in multiple short and large-scale navigation tasks on challenging off-road terrains using two different large, all-terrain robots. Our short-scale navigation results show that using our learned costmaps leads to overall smoother navigation, and provides the robot with a more fine-grained understanding of the robot-terrain interactions. Our large-scale navigation trials show that we can reduce the number of interventions by up to 57% compared to an occupancy-based navigation baseline in challenging off-road courses ranging from 400 m to 3150 m. Appendix and full experiment videos can be found in our website: https://mateoguaman.github.io/hdif.
更多查看译文
AI 理解论文
溯源树
样例
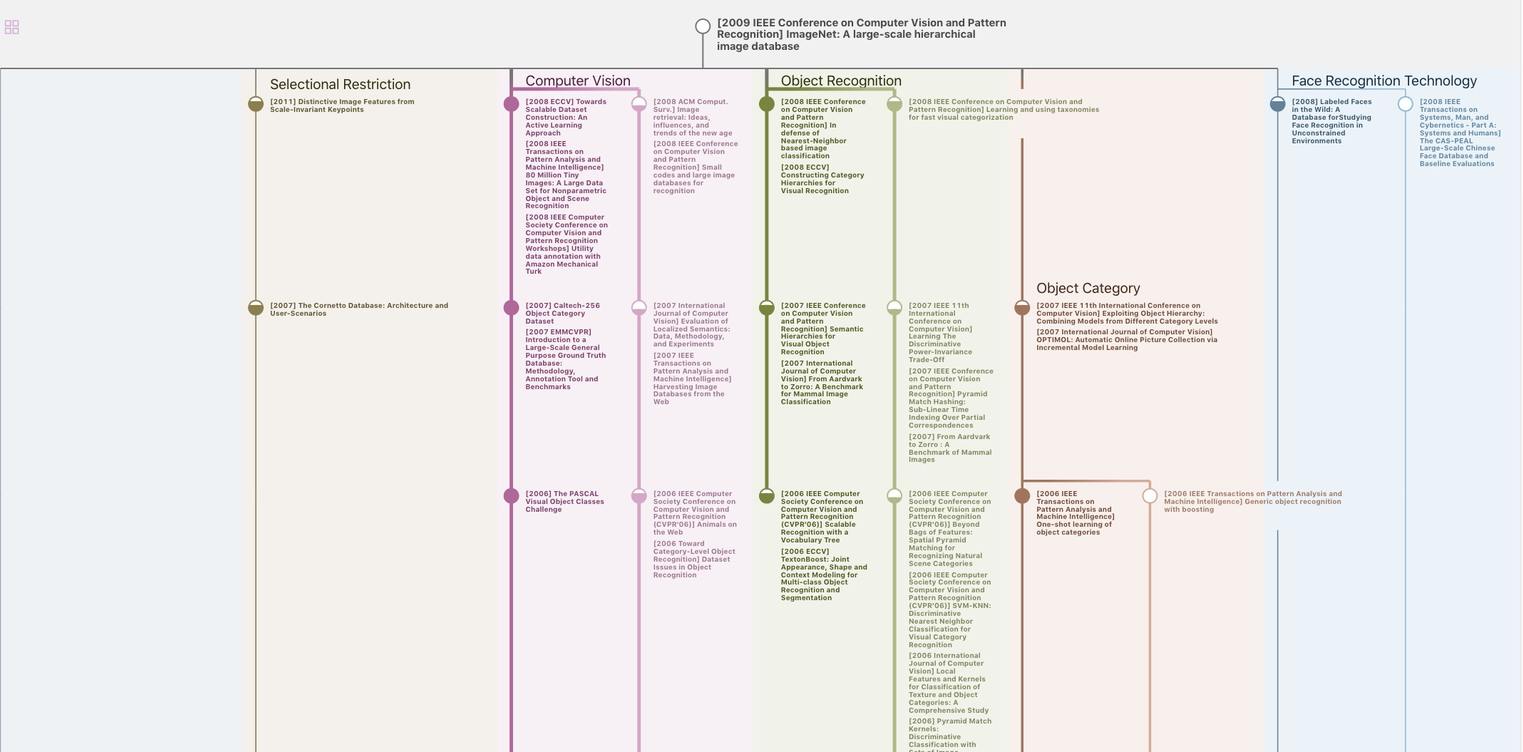
生成溯源树,研究论文发展脉络
Chat Paper
正在生成论文摘要