Attention Beats Concatenation for Conditioning Neural Fields
arxiv(2022)
摘要
Neural fields model signals by mapping coordinate inputs to sampled values. They are becoming an increasingly important backbone architecture across many fields from vision and graphics to biology and astronomy. In this paper, we explore the differences between common conditioning mechanisms within these networks, an essential ingredient in shifting neural fields from memorization of signals to generalization, where the set of signals lying on a manifold is modelled jointly. In particular, we are interested in the scaling behaviour of these mechanisms to increasingly high-dimensional conditioning variables. As we show in our experiments, high-dimensional conditioning is key to modelling complex data distributions, thus it is important to determine what architecture choices best enable this when working on such problems. To this end, we run experiments modelling 2D, 3D, and 4D signals with neural fields, employing concatenation, hyper-network, and attention-based conditioning strategies -- a necessary but laborious effort that has not been performed in the literature. We find that attention-based conditioning outperforms other approaches in a variety of settings.
更多查看译文
关键词
attention beats concatenation,conditioning neural fields
AI 理解论文
溯源树
样例
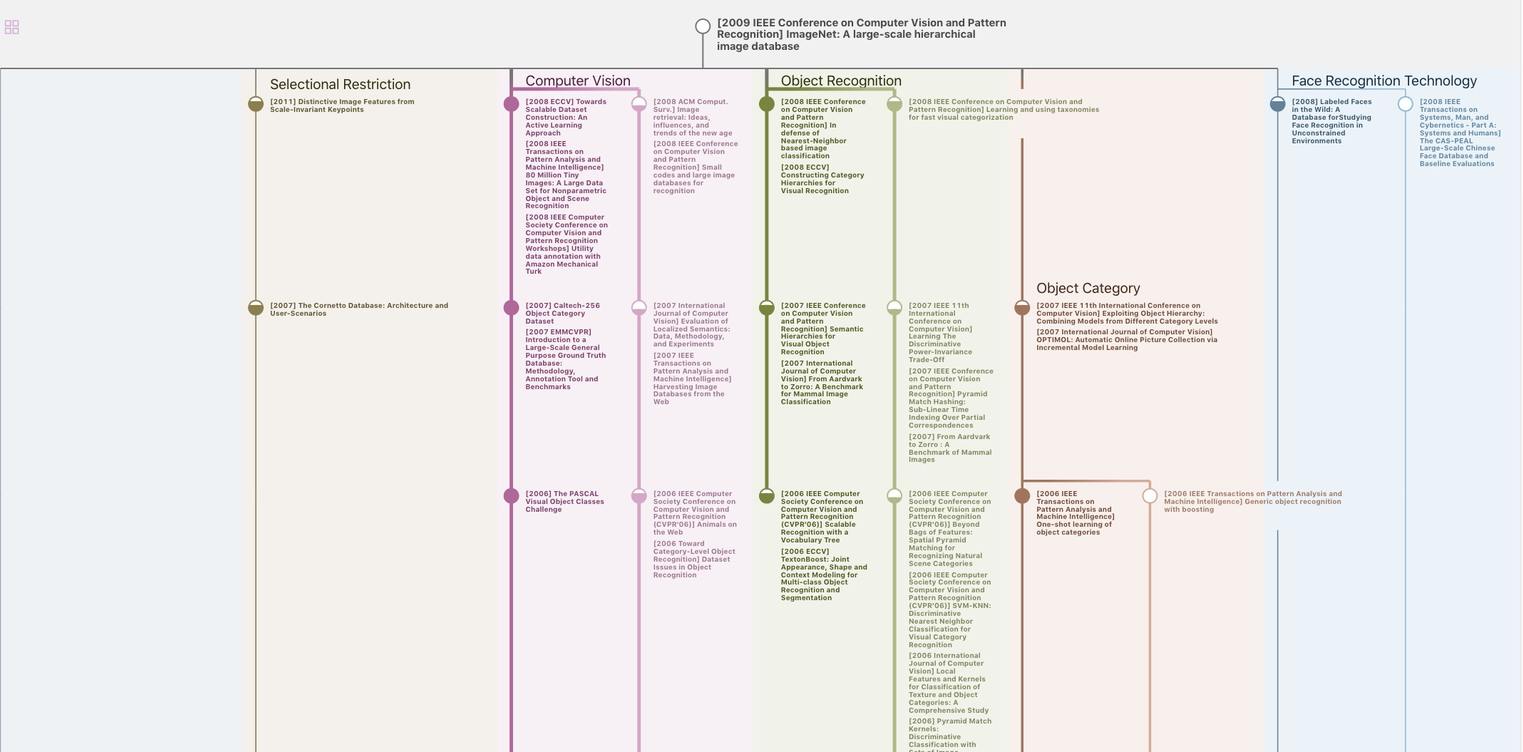
生成溯源树,研究论文发展脉络
Chat Paper
正在生成论文摘要