Data-Driven Models for Predicting Solar Radiation in Semi-Arid Regions
Computers, Materials & Continua(2023)
摘要
Solar energy represents one of the most important renewable energy sources contributing to the energy transition process. Considering that the observation of daily global solar radiation (GSR) is not affordable in some parts of the globe, there is an imperative need to develop alternative ways to predict it. Therefore, the main objective of this study is to evaluate the performance of different hybrid data-driven techniques in predicting daily GSR in semi-arid regions, such as the majority of Spanish territory. Here, four ensemble-based hybrid models were developed by hybridizing Addi-tive Regression (AR) with Random Forest (RF), Locally Weighted Linear Regression (LWLR), Random Subspace (RS), and M5P. The base algorithms of the developed models are scarcely applied in previous studies to predict solar radiation. The testing phase outcomes demonstrated that the AR-RF models outperform all other hybrid models. The provided models were validated by statistical metrics, such as the correlation coefficient (R) and root mean square error (RMSE). The results proved that Scenario #6, utilizing extraterrestrial solar radiation, relative humidity, wind speed, and mean, maximum, and minimum ambient air temperatures as the model inputs, leads to the most accurate predictions among all scenarios (R = 0.968-0.988 and RMSE = 1.274-1.403 MJ/m2middotd). Also, Scenario #3 stood in the next rank of accuracy for predicting the solar radiation in both validating stations. The AD-RF model was the best predictive, followed by AD-RS and AD-LWLR. Hence, this study recommends new effective methods to predict GSR in semi-arid regions.
更多查看译文
关键词
Solar radiation prediction,random forest,locally-weighted linear regression,additive regression
AI 理解论文
溯源树
样例
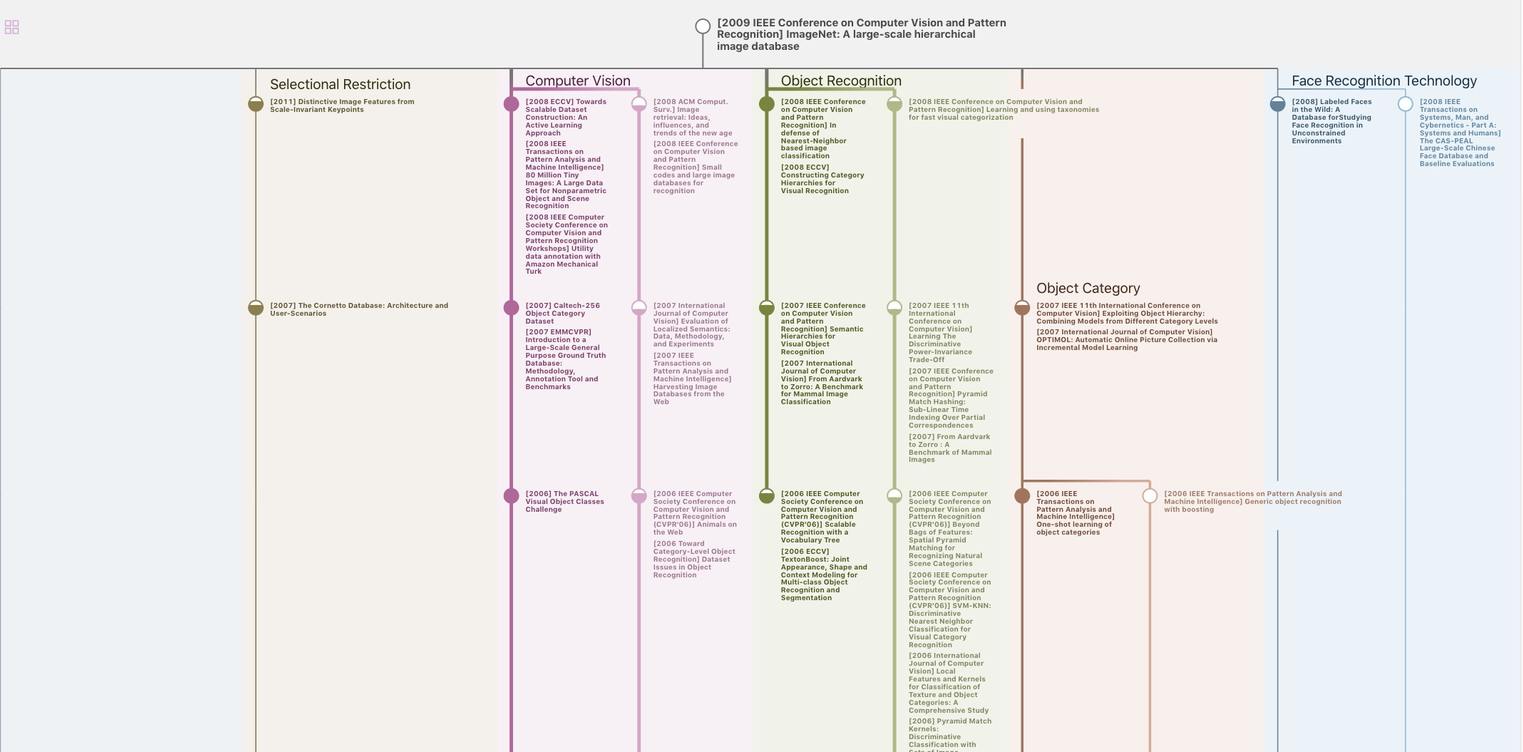
生成溯源树,研究论文发展脉络
Chat Paper
正在生成论文摘要