Adaptive Local-Component-aware Graph Convolutional Network for One-shot Skeleton-based Action Recognition
WACV(2023)
摘要
Skeleton-based action recognition receives increasing attention because skeleton sequences reduce training complexity by eliminating visual information irrelevant to actions. To further improve sample efficiency, meta-learning-based one-shot learning solutions were developed for skeleton-based action recognition. These methods predict by finding the nearest neighbors according to the similarity between instance-level global embedding. However, such measurement holds unstable representativity due to inadequate generalized learning on the averaged local invariant and noisy features, while intuitively, steady and fine-grained recognition relies on determining key local body movements. To address this limitation, we present the Adaptive Local-Component-aware Graph Convolutional Network, which replaces the comparison metric with a focused sum of similarity measurements on aligned local embedding of action-critical spatial/temporal segments. Comprehensive one-shot experiments on the public benchmark of NTURGB+D 120 indicate that our method provides a stronger representation than the global embedding and helps our model reach state-of-the-art.
更多查看译文
关键词
Algorithms: Video recognition and understanding (tracking,action recognition,etc.),Machine learning architectures,formulations,and algorithms (including transfer,low-shot,semi-,self-,and un-supervised learning)
AI 理解论文
溯源树
样例
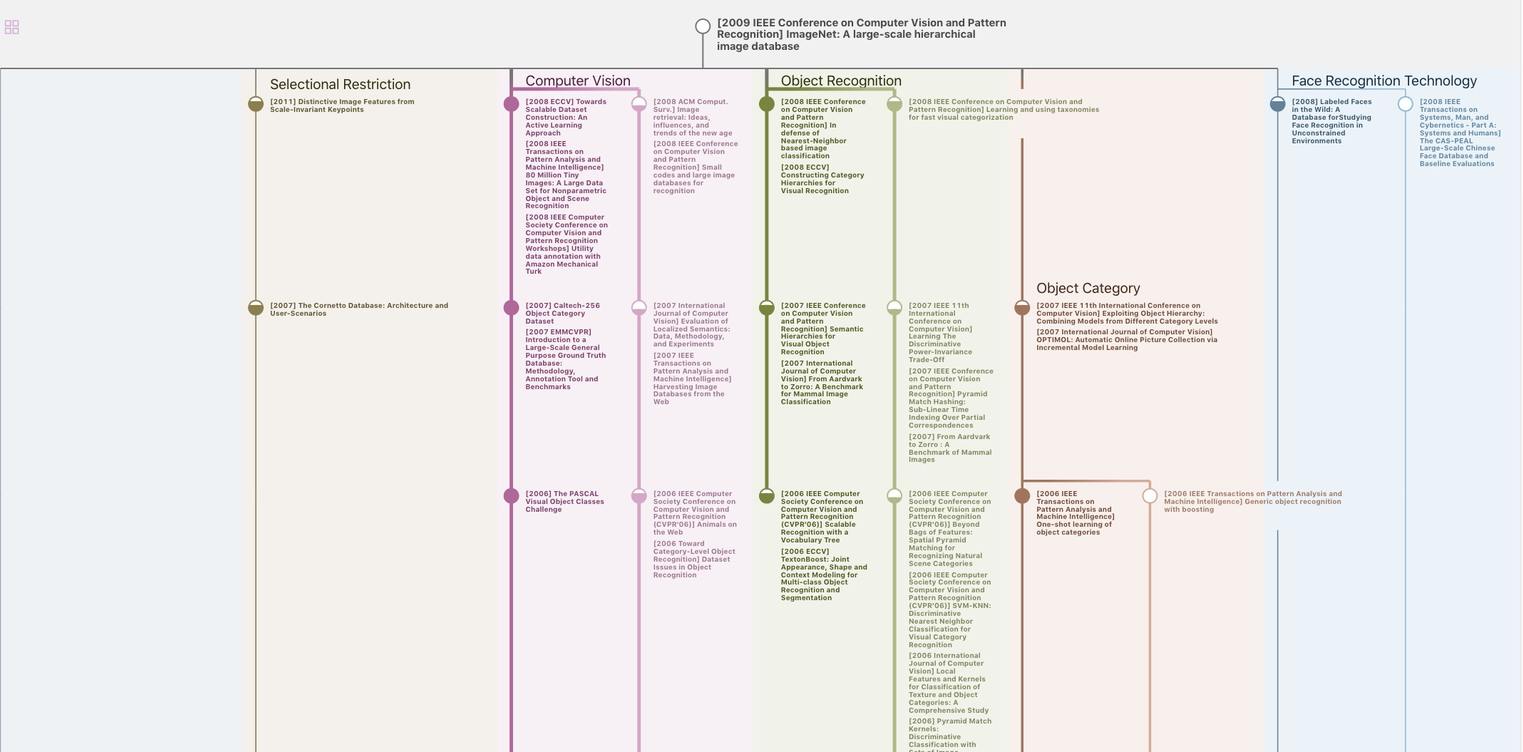
生成溯源树,研究论文发展脉络
Chat Paper
正在生成论文摘要