ECSAS: Exploring Critical Scenarios from Action Sequence in Autonomous Driving
2023 IEEE 32ND ASIAN TEST SYMPOSIUM, ATS(2023)
摘要
Rare critical scenarios are crucial to verify the performance of autonomous driving in different situations. Critical scenario generation requires the ability of sampling critical combinations from an infinite parameter space in the logical scenario. Existing solutions aim to explore the correlation of action parameters in the initial scenario rather than action sequences. How to model action sequences so that one can further consider the effects of different action parameters is the bottleneck of the problem. In this paper, we solve the problem by proposing the ECSAS framework. Specifically, we first propose a description language, BTScenario, allowing us to model action sequences of scenarios. We then use reinforcement learning to search for combinations of critical action parameters. Several optimizations are proposed to increase efficiency, including action mask and replay buffer. Experimental results show that our model with strong collision ability and effectively outperforms the existing methods on various nontrivial scenarios.
更多查看译文
关键词
Critical scenario generation,Reinforcement learning,Scenario description language and Autonomous driving
AI 理解论文
溯源树
样例
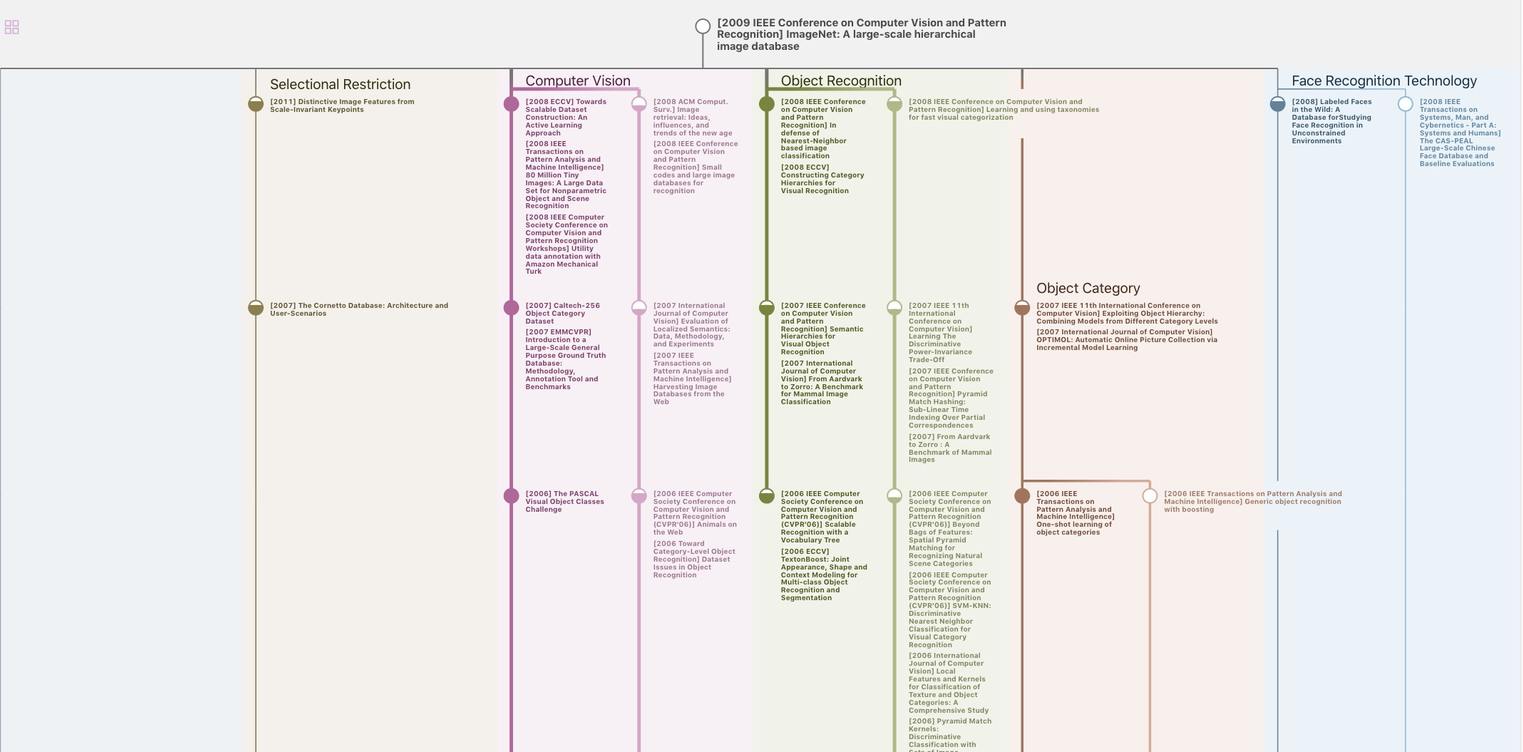
生成溯源树,研究论文发展脉络
Chat Paper
正在生成论文摘要