Multistate modeling and structure selection for multitype recurrent events and terminal event data.
Biometrical journal. Biometrische Zeitschrift(2023)
摘要
In cardiovascular disease studies, a large number of risk factors are measured but it often remains unknown whether all of them are relevant variables and whether the impact of these variables is changing with time or remains constant. In addition, more than one kind of cardiovascular disease events can be observed in the same patient and events of different types are possibly correlated. It is expected that different kinds of events are associated with different covariates and the forms of covariate effects also vary between event types. To tackle these problems, we proposed a multistate modeling framework for the joint analysis of multitype recurrent events and terminal event. Model structure selection is performed to identify covariates with time-varying coefficients, time-independent coefficients, and null effects. This helps in understanding the disease process as it can detect relevant covariates and identify the temporal dynamics of the covariate effects. It also provides a more parsimonious model to achieve better risk prediction. The performance of the proposed model and selection method is evaluated in numerical studies and illustrated on a real dataset from the Atherosclerosis Risk in Communities study.
更多查看译文
关键词
adaptive group lasso,multistate model,polynomial splines,probability prediction,time-varying coefficients
AI 理解论文
溯源树
样例
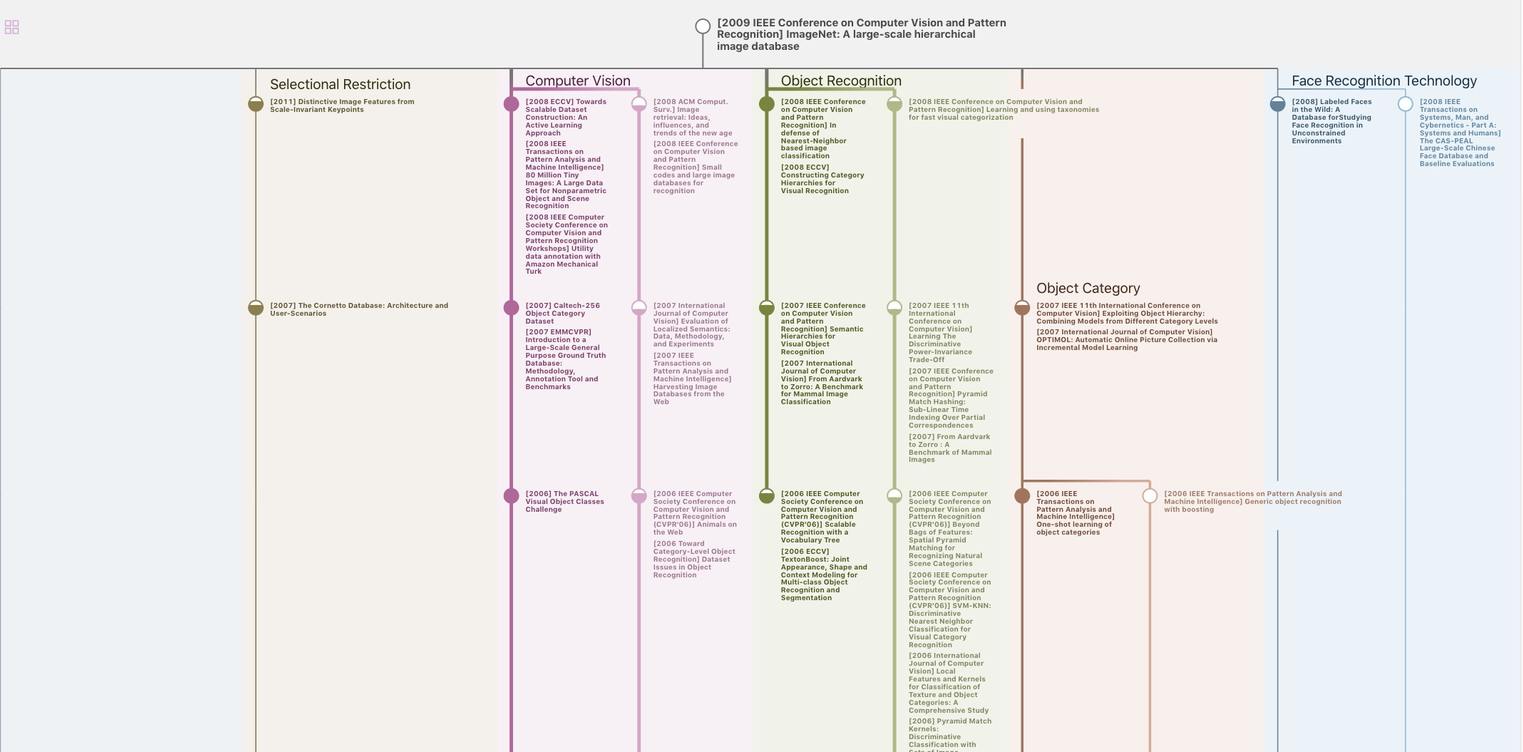
生成溯源树,研究论文发展脉络
Chat Paper
正在生成论文摘要