Transport-Based Anatomical-Functional Metric Learning for Liver Tumor Recognition Using Dual-View Dynamic CEUS Imaging
IEEE Transactions on Biomedical Engineering(2023)
摘要
Hepatocellular carcinoma (HCC) and intrahepatic cholangiocarcinoma (ICC) are the most common malignancy arising from primary liver cancer (PLC). Liver ultrasound (US) has been the main approach for the early screening and differential diagnosis. Since ultrasonic findings of PLC are closely associated with tumor growth pattern, histological morphology, tumor staging, and other factors, ultrasonic imaging findings overlap partially. Besides, qualitative assessment is highly dependent on expertise. To improve the diagnostic objectiveness, we propose a novel transport-based anatomical-functional metric learning (T-AFML) method to
quantify imaging similarity
of both the gray-scale US and dynamic contrast-enhanced US view. Considering that the hemodynamic changes vary with individuals, we introduce a temporally regularized optimal transport to align the local enhancement patterns automatically. To sufficiently exploit ultrasonic findings similarity from different modalities, a selector-based metric integration mechanism is adopted to adaptively select a dominant modality accounting for the similarity measure. In this retrospective study, we collected a total of 174 liver cancer patients consists of 105 HCC and 69 ICC, and our method achieves the superior diagnostic accuracy and sensitivity of 88.41% and 86.16%, respectively, demonstrating its efficacy in quantifying multi-modal ultrasonic findings similarity for PLC diagnosis.
更多查看译文
关键词
Imaging similarity measure,optimal transport,primary liver cancer,temporal alignment
AI 理解论文
溯源树
样例
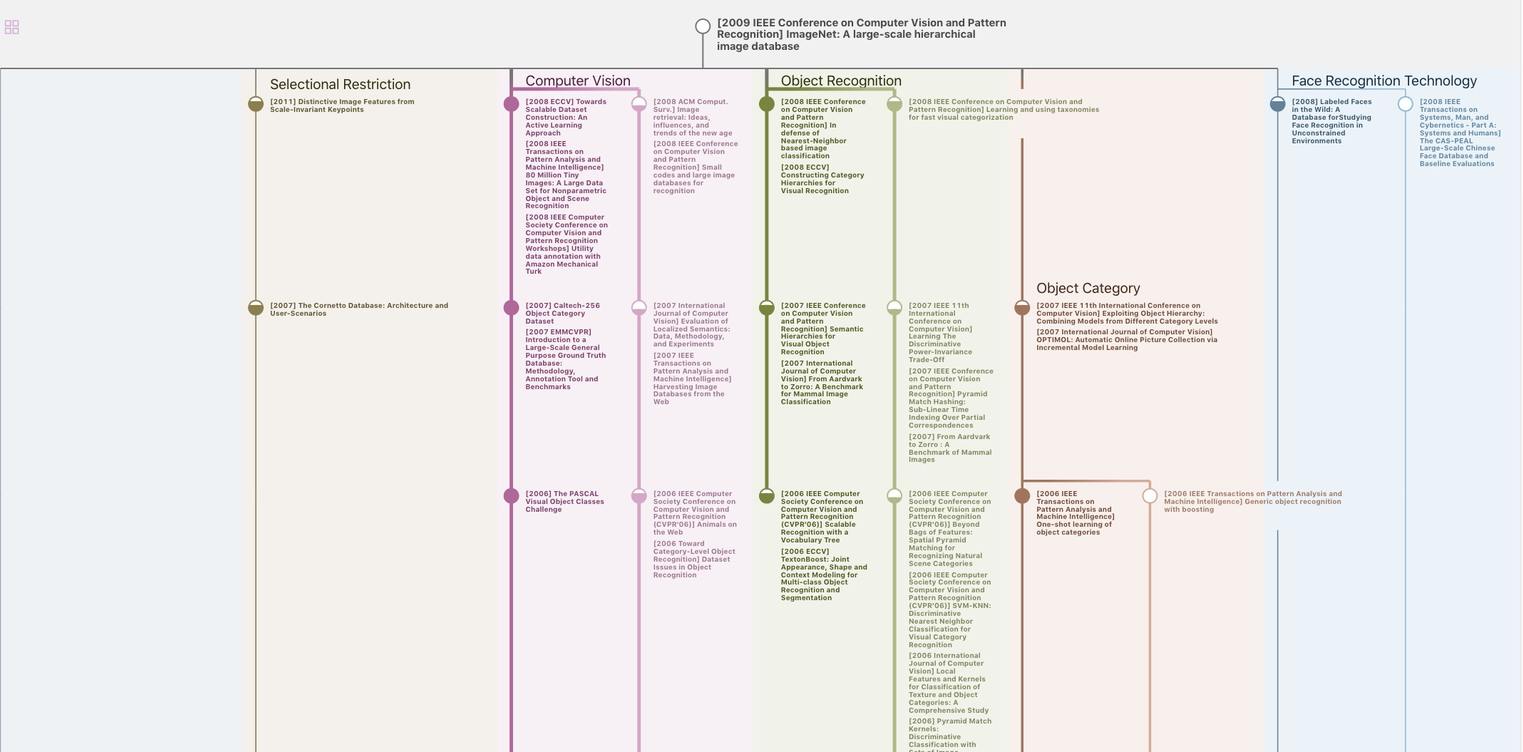
生成溯源树,研究论文发展脉络
Chat Paper
正在生成论文摘要