A novel deep learning-based fault diagnosis algorithm for preventing protection malfunction
International Journal of Electrical Power & Energy Systems(2023)
摘要
To prevent serious malfunctions and reduce the impact of faults during an emergency state of a power system, protection systems are required to have disturbance and fault state identification abilities. In this study, a novel fault diagnosis framework based on deep learning with anti-disturbance ability is proposed to identify the fault state and fault type information, even under the influence of system disturbance. The framework consists of two parts: unsupervised and supervised learning. Specifically, an unsupervised deep auto-encoder (DAE) is applied for offline feature selection and data cleaning. The DAE can extract key fault features and significantly improve the fault detection accuracy. Furthermore, two supervised convolutional neural networks are used to learn key fault feature extraction online from complex operation information in power systems and assess the fault situ-ation and type. Using case studies, the proposed method was implemented and compared with existing intelli-gent methods. The results indicate that the proposed framework has a better performance in terms of fault state identification and protection malfunction prevention.
更多查看译文
关键词
DL,Deep auto-encoder,Fault diagnosis,Unsupervised and supervised learning,Disturbance,Malfunction prevention
AI 理解论文
溯源树
样例
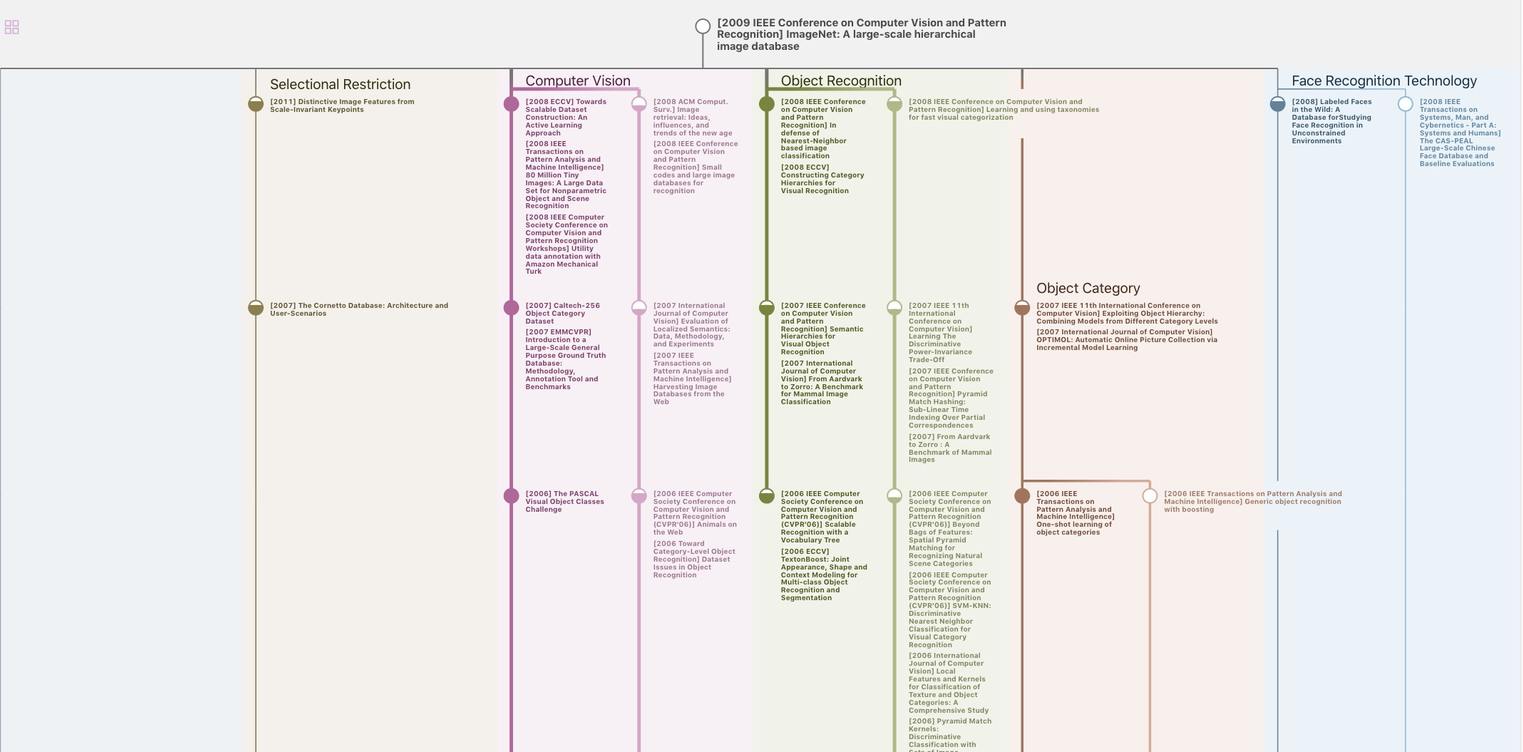
生成溯源树,研究论文发展脉络
Chat Paper
正在生成论文摘要