CoV-TI-Net: Transferred Initialization with Modified End Layer for COVID-19 Diagnosis.
SMC(2022)
摘要
This paper proposes transferred initialization with modified fully connected layers for COVID-19 diagnosis. Convolutional neural networks (CNN) achieved a remarkable result in image classification. However, training a high-performing model is a very complicated and time-consuming process because of the complexity of image recognition applications. On the other hand, transfer learning is a relatively new learning method that has been employed in many sectors to achieve good performance with fewer computations. In this research, the PyTorch pre-trained models (VGG19\_bn and WideResNet -101) are applied in the MNIST dataset for the first time as initialization and with modified fully connected layers. The employed PyTorch pre-trained models were previously trained in ImageNet. The proposed model is developed and verified in the Kaggle notebook, and it reached the outstanding accuracy of 99.77% without taking a huge computational time during the training process of the network. We also applied the same methodology to the SIIM-FISABIO-RSNA COVID-19 Detection dataset and achieved 80.01% accuracy. In contrast, the previous methods need a huge compactional time during the training process to reach a high-performing model. Codes are available at the following link: github.com/dipuk0506/SpinalNet
更多查看译文
关键词
Transfer learning,MNIST,COVID,VGG,Py-Torch,WideResNet,SpinalNet.
AI 理解论文
溯源树
样例
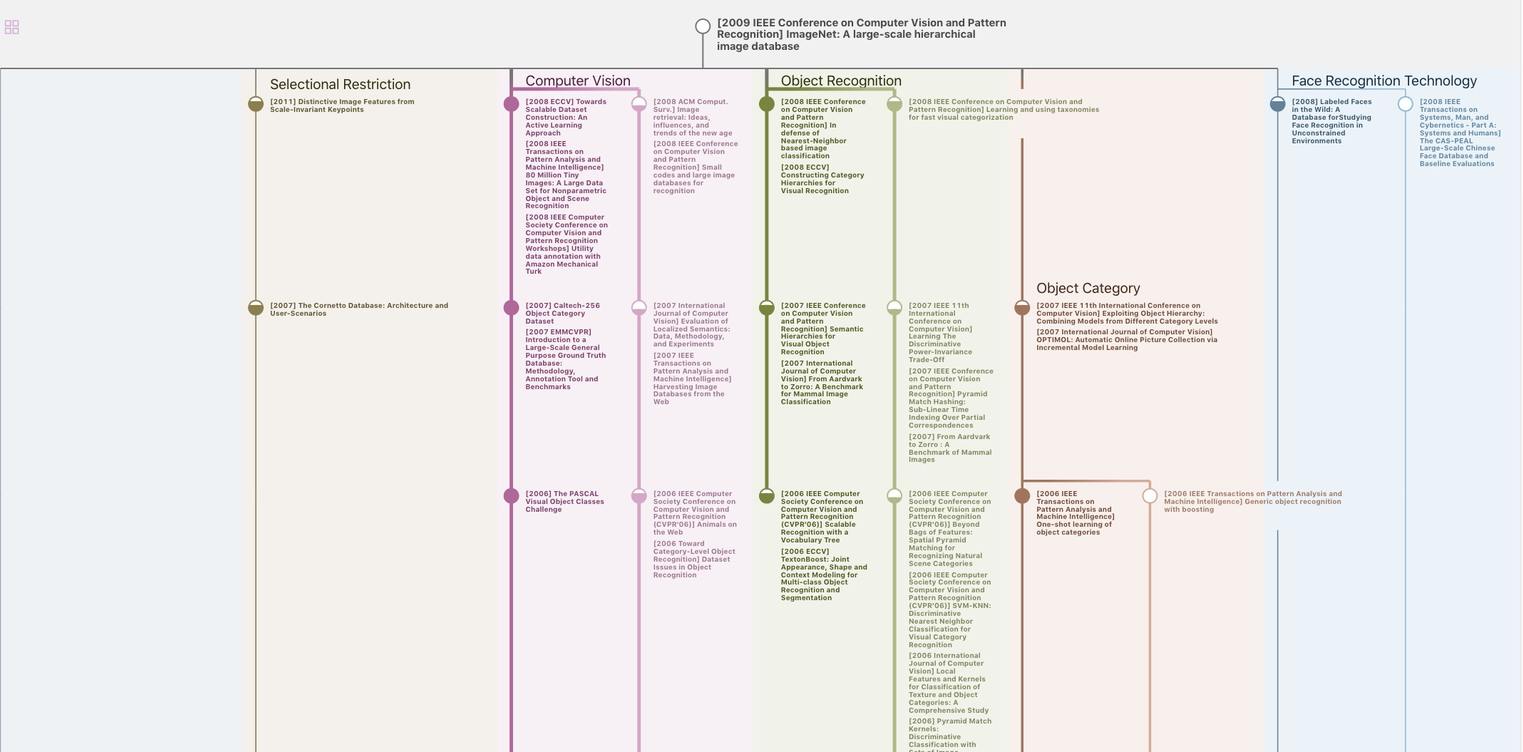
生成溯源树,研究论文发展脉络
Chat Paper
正在生成论文摘要