Deep Label Prior: Pre-Training-Free Salient Object Detection Network Based on Label Learning
IEEE TRANSACTIONS ON MULTIMEDIA(2023)
摘要
Due to the excellent semantics extraction capabilities, deep learning methods have significantly progressed in salient object detection (SOD). However, these methods often require time-consuming pre-training and large training datasets with ground truth. To address these issues, by referring to the framework known as "deep image prior (DIP)," we propose a SOD method called deep label prior network (DLPNet), which consists of A-stream and B-stream. The A-stream includes two cascaded UNets and a simple CNNs module to extract the initial saliency map, while the B-stream contains only two cascaded UNets, which refines the extracted initial saliency map. Unlike most of the current deep learning methods, DLPNet views the SOD task as a conditional image generation problem, relying on only the internal prior of the input itself to generate the saliency map. Hence, our DLPNet does not require pre-training or large annotated / unannotated datasets. Furthermore, we propose a morphology operation scheme, which creates rich pseudo-labels for facilitating the updating of network weights. Extensive experiments demonstrate that our method outperforms state-of-the-art unsupervised techniques and is even comparable to state-of-the-art supervised and weakly supervised methods on different evaluation metrics.
更多查看译文
关键词
Salient object detection,deep label prior,morphology operation,deep image prior
AI 理解论文
溯源树
样例
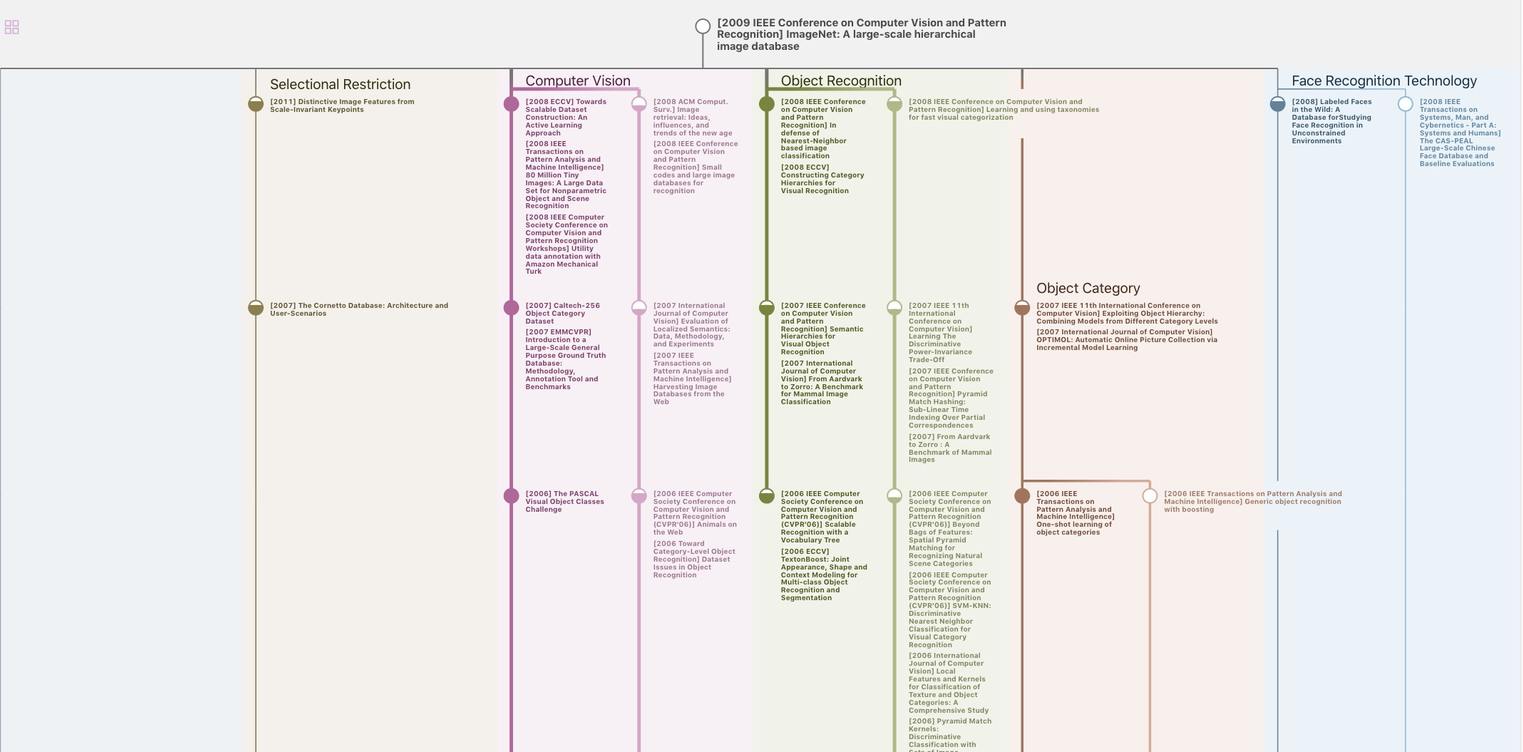
生成溯源树,研究论文发展脉络
Chat Paper
正在生成论文摘要