Boreal Forest Height Mapping using Sentinel-1 Time Series and improved LSTM model
biorxiv(2022)
摘要
Here, a novel semi-supervised Long Short-Term Memory (LSTM) model is developed and demonstrated for predicting forest tree height using time series of Sentinel-1 images. The model uses a Helix-Elapse (HE) projection approach to capture relationship between forest temporal patterns and Sentinel-1 time series, when the acquisition time intervals are irregular. A skip-link based LSTM block is introduced and a novel backbone network, Helix-LSTM, is proposed to retrieve temporal features at different receptive scales. Additionally, a novel semi-supervised strategy, Cross-Pseudo Regression, is employed to achieve better model performance. The developed model is compared versus basic LSTM model, attention-based bidirectional LSTM and several other established regression approaches used in forest variable mapping, demonstrating consistent improvement of forest height prediction accuracy. The study site is located in Central Finland and represents boreal forestland. At best, the achieved accuracy of forest height mapping was 28.3% rRMSE for pixel-level predictions, and 18.0% rRMSE on stand level. We expect that the developed model can also be used for modeling relationships between other forest variables and satellite image time series.
### Competing Interest Statement
The authors have declared no competing interest.
更多查看译文
关键词
lstm,forest,time series,mapping
AI 理解论文
溯源树
样例
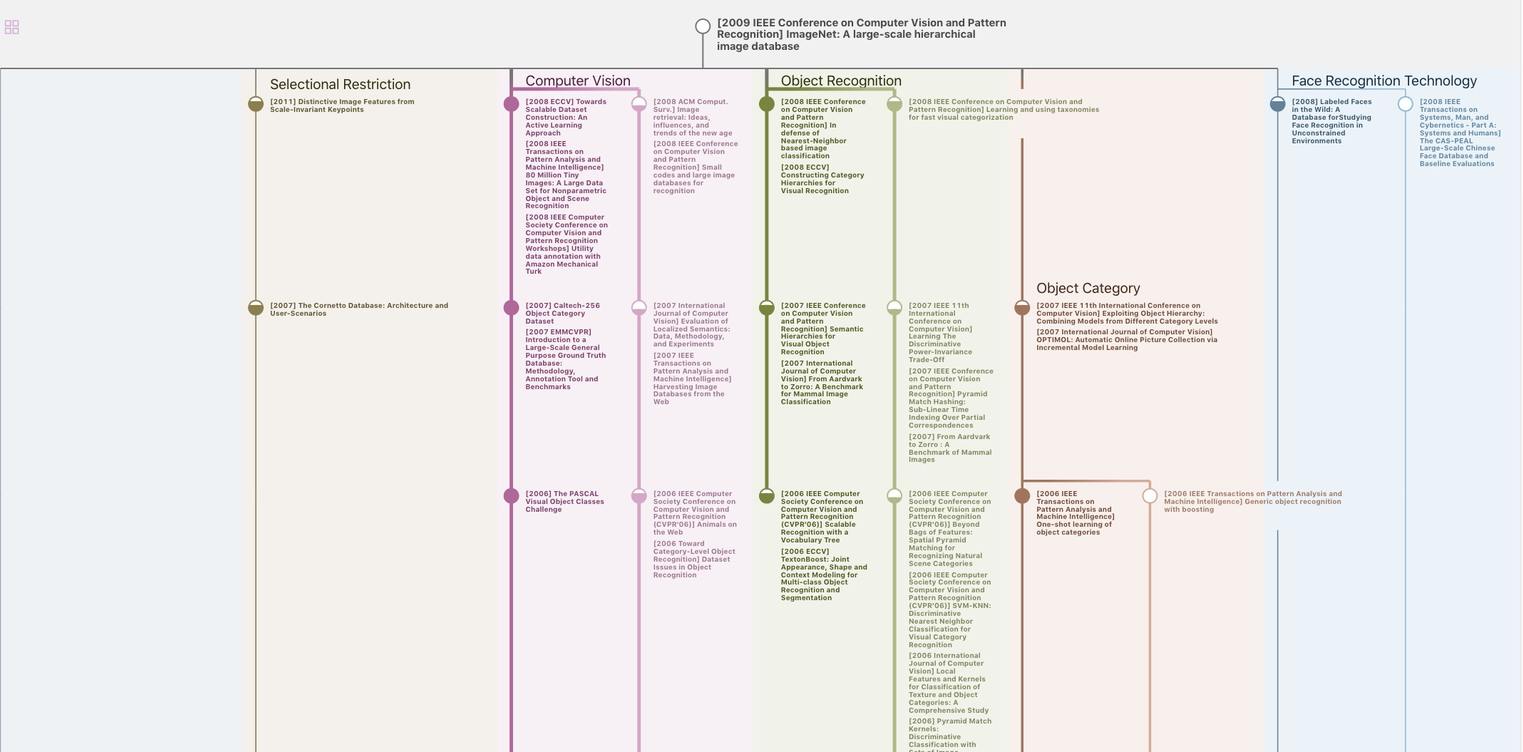
生成溯源树,研究论文发展脉络
Chat Paper
正在生成论文摘要