Real-Time Online Video Detection with Temporal Smoothing Transformers.
European Conference on Computer Vision(2022)
摘要
Streaming video recognition reasons about objects and their actions in every frame of a video. A good streaming recognition model captures both long-term dynamics and short-term changes of video. Unfortunately, in most existing methods, the computational complexity grows linearly or quadratically with the length of the considered dynamics. This issue is particularly pronounced in transformer-based architectures. To address this issue, we reformulate the cross-attention in a video transformer through the lens of kernel and apply two kinds of temporal smoothing kernel: A box kernel or a Laplace kernel. The resulting streaming attention reuses much of the computation from frame to frame, and only requires a constant time update each frame. Based on this idea, we build TeSTra, a Temporal Smoothing Transformer, that takes in arbitrarily long inputs with constant caching and computing overhead. Specifically, it runs $6\times$ faster than equivalent sliding-window based transformers with 2,048 frames in a streaming setting. Furthermore, thanks to the increased temporal span, TeSTra achieves state-of-the-art results on THUMOS'14 and EPIC-Kitchen-100, two standard online action detection and action anticipation datasets. A real-time version of TeSTra outperforms all but one prior approaches on the THUMOS'14 dataset.
更多查看译文
关键词
Online action detection,Action anticipation,Transformer,Temporal smoothing kernel
AI 理解论文
溯源树
样例
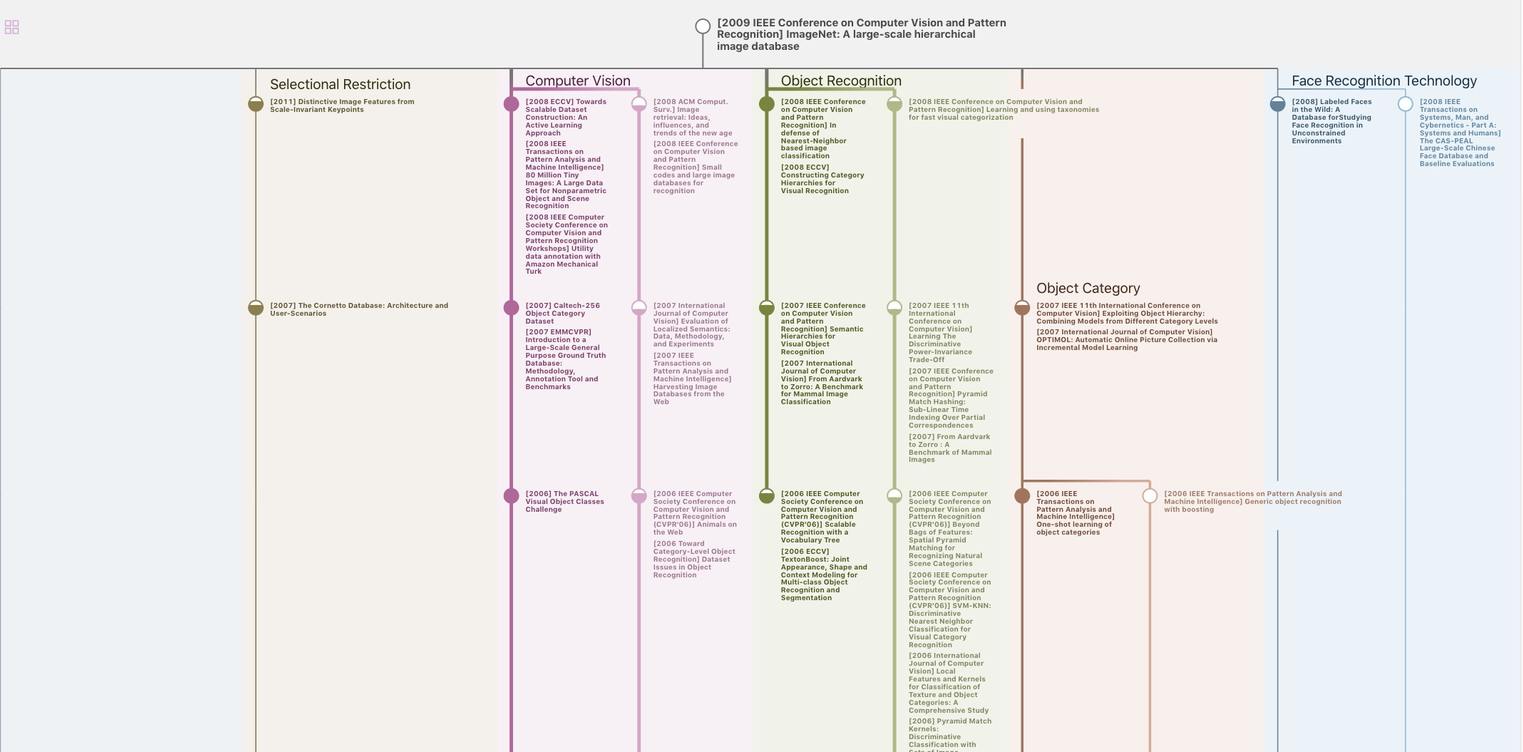
生成溯源树,研究论文发展脉络
Chat Paper
正在生成论文摘要