Deep Variation Prior: Joint Image Denoising and Noise Variance Estimation Without Clean Data
IEEE TRANSACTIONS ON IMAGE PROCESSING(2024)
摘要
With recent deep learning based approaches showing promising results in removing noise from images, the best denoising performance has been reported in a supervised learning setup that requires a large set of paired noisy images and ground truth data for training. The strong data requirement can be mitigated by unsupervised learning techniques, however, accurate modelling of images or noise variances is still crucial for high-quality solutions. The learning problem is ill-posed for unknown noise distributions. This paper investigates the tasks of image denoising and noise variance estimation in a single, joint learning framework. To address the ill-posedness of the problem, we present deep variation prior (DVP), which states that the variation of a properly learnt denoiser with respect to the change of noise satisfies some smoothness properties, as a key criterion for good denoisers. Building upon DVP and under the assumption that the noise is zero mean and pixel-wise independent conditioned on the image, an unsupervised deep learning framework, that simultaneously learns a denoiser and estimates noise variances, is developed. Our method does not require any clean training images or an external step of noise estimation, and instead, approximates the minimum mean squared error denoisers using only a set of noisy images. With the two underlying tasks being considered in a single framework, we allow them to be optimised for each other. The experimental results show a denoising quality comparable to that of supervised learning and accurate noise variance estimates.
更多查看译文
关键词
Image denoising,unsupervised learning,noise level estimation,deep learning without ground truth
AI 理解论文
溯源树
样例
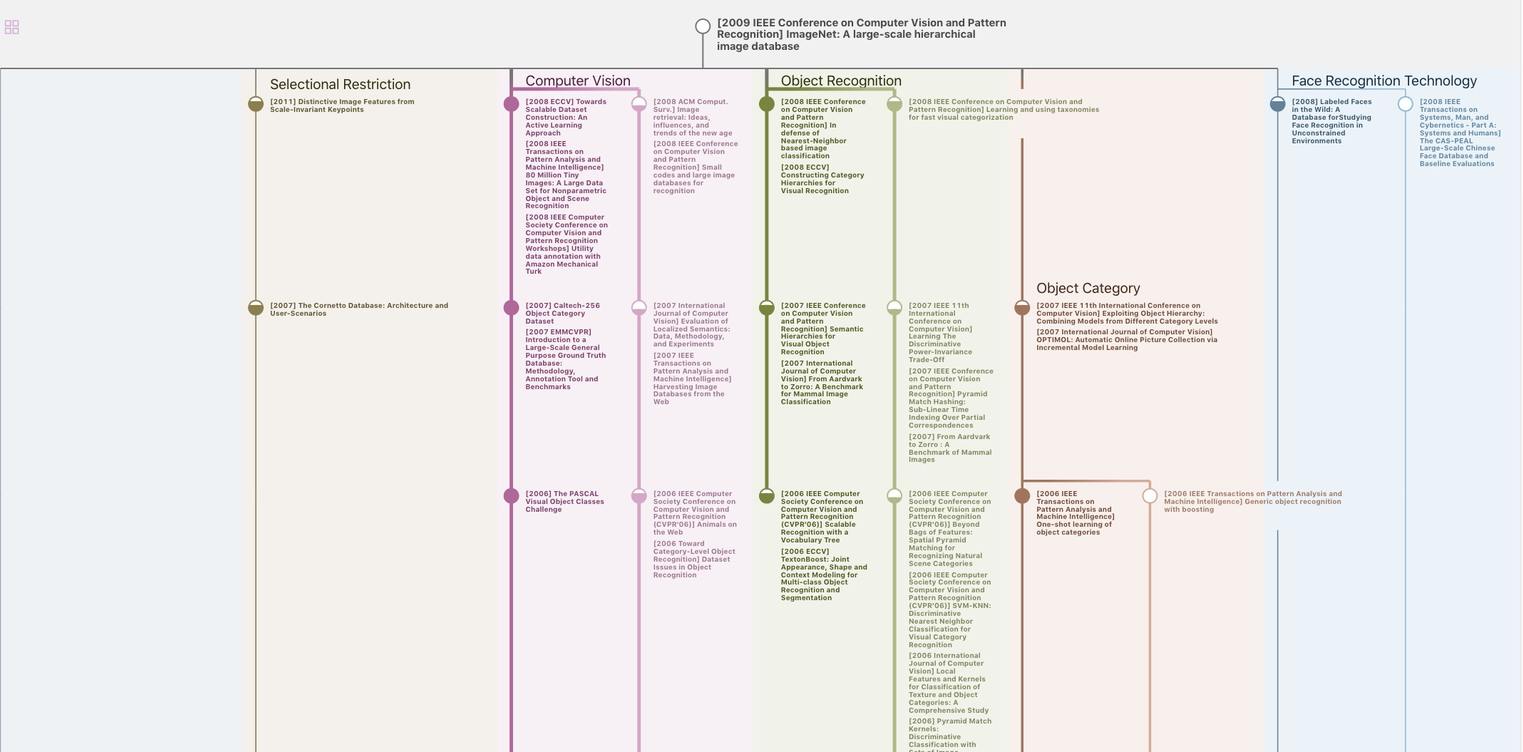
生成溯源树,研究论文发展脉络
Chat Paper
正在生成论文摘要