Can Segmentation Models Be Trained with Fully Synthetically Generated Data?
SIMULATION AND SYNTHESIS IN MEDICAL IMAGING, SASHIMI 2022(2022)
摘要
In order to achieve good performance and generalisability, medical image segmentation models should be trained on sizeable datasets with sufficient variability. Due to ethics and governance restrictions, and the costs associated with labelling data, scientific development is often stifled, with models trained and tested on limited data. Data augmentation is often used to artificially increase the variability in the data distribution and improve model generalisability. Recent works have explored deep generative models for image synthesis, as such an approach would enable the generation of an effectively infinite amount of varied data, addressing the generalisability and data access problems. However, many proposed solutions limit the user's control over what is generated. In this work, we propose brainSPADE, a model which combines a synthetic diffusion-based label generator with a semantic image generator. Our model can produce fully synthetic brain labels on-demand, with or without pathology of interest, and then generate a corresponding MRI image of an arbitrary guided style. Experiments show that brainSPADE synthetic data can be used to train segmentation models with performance comparable to that of models trained on real data.
更多查看译文
关键词
segmentation models,fully synthetically,data
AI 理解论文
溯源树
样例
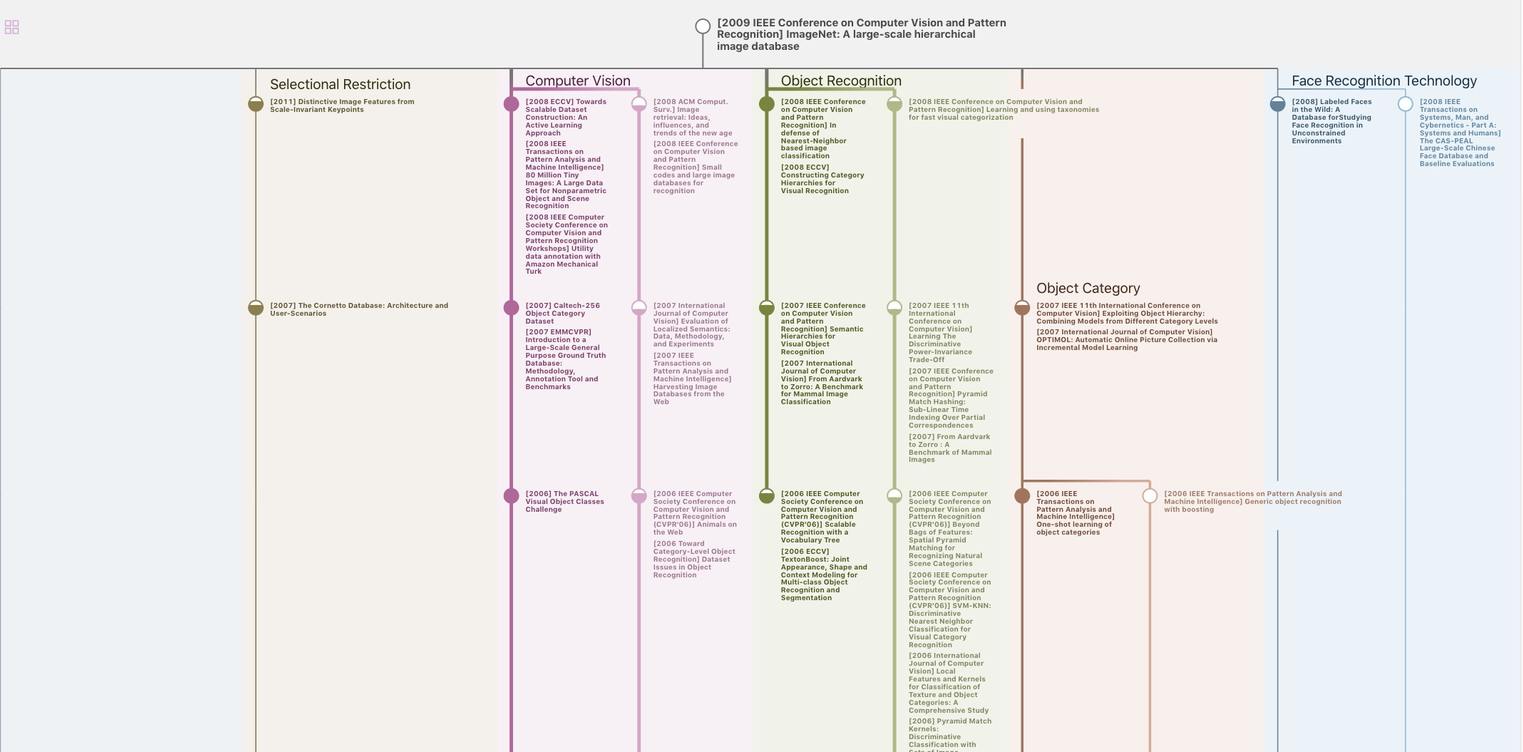
生成溯源树,研究论文发展脉络
Chat Paper
正在生成论文摘要