Searching for Energy-Efficient Hybrid Adder-Convolution Neural Networks.
IEEE Conference on Computer Vision and Pattern Recognition(2022)
摘要
As convolutional neural networks (CNNs) are more and more widely used in computer vision area, the energy consumption of CNNs has become the focus of researchers. For edge devices, both the battery life and the inference latency are critical and directly affect user experience. Recently, great progress has been made in the design of neural architectures and new operators. The emergence of neural architecture search technology has improved the performance of network step by step, and liberated the productivity of engineers to a certain extent. New operators, such as AdderNets, make it possible to further improve the energy efficiency of neural networks. In this paper, we explore the fusion of new adder operators and common convolution operators into state-of-the-art light-weight networks, GhostNet, to search for models with better energy efficiency and performance. Our proposed search equilibrium strategy ensures that the adder and convolution operators can be treated fairly in the search, and the resulting model achieves the same accuracy of 73.9% with GhostNet on the ImageNet dataset at an extremely low power consumption of 0.612 mJ. When keeping the same energy consumption, the accuracy reaches 74.3% which is 0.4% higher than original GhostNet.
更多查看译文
关键词
ImageNet dataset,GhostNet,inference latency,battery life,edge devices,search equilibrium strategy,light-weight networks,common convolution operators,adder operators,neural architecture search technology,energy consumption,computer vision area,CNN,energy-efficient hybrid adder-convolution neural networks
AI 理解论文
溯源树
样例
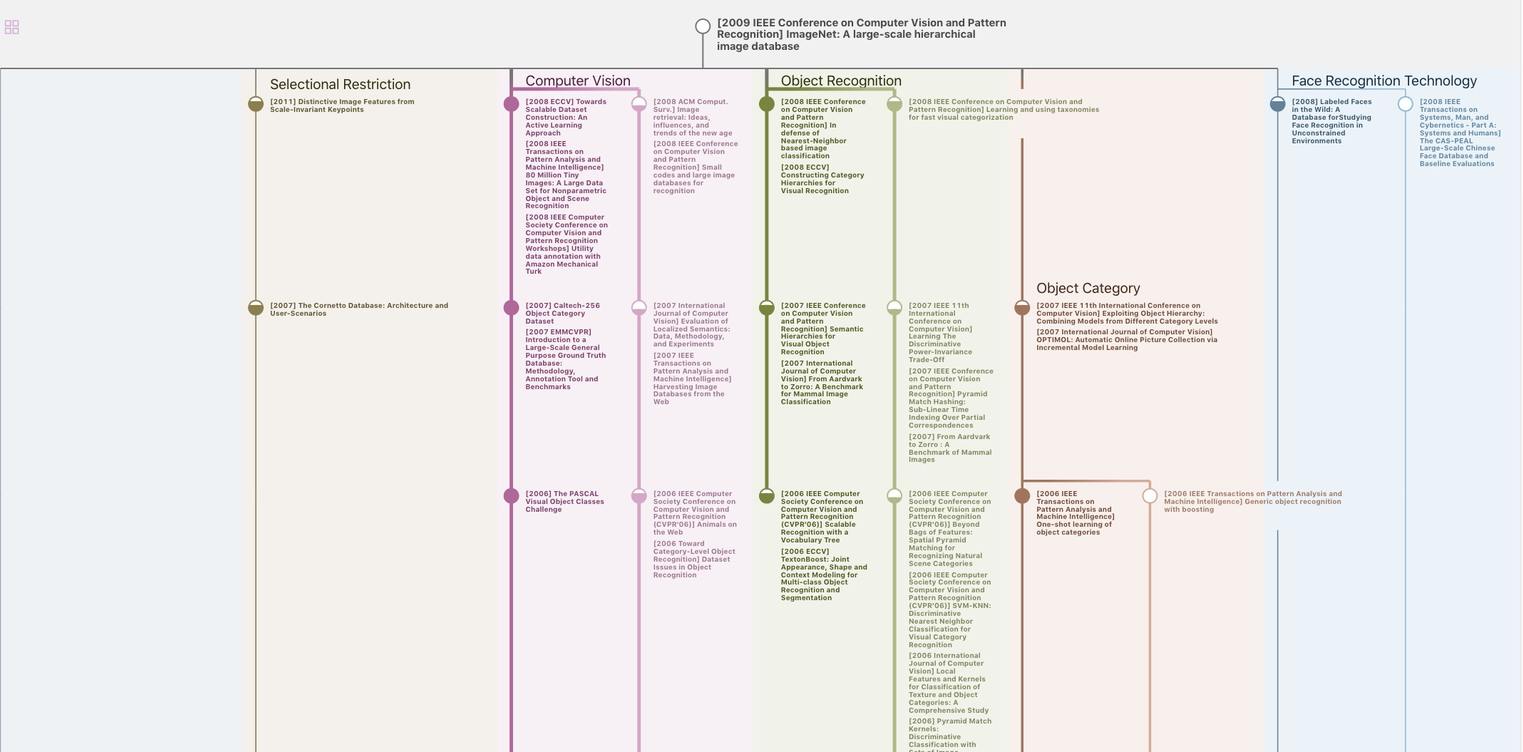
生成溯源树,研究论文发展脉络
Chat Paper
正在生成论文摘要