Efficient Image Super-Resolution with Collapsible Linear Blocks
2022 IEEE/CVF Conference on Computer Vision and Pattern Recognition Workshops (CVPRW)(2022)
摘要
In this paper, we propose a simple but effective architecture for fast and accurate single image super-resolution. Unlike other compact image super-resolution methods based on hand-crafted designs, we first apply coarse-grained pruning for network acceleration, and then introduce collapsible linear blocks to recover the representative ability of the pruned network. Specifically, each collapsible linear block has a multi-branch topology during training, and can be equivalently replaced with a single convolution in the inference stage. Such decoupling of the training-time and inference-time architecture is implemented via a structural re-parameterization technique, leading to improved representation without introducing extra computation costs. Additionally, we adopt a two-stage training mechanism with progressively larger patch sizes to facilitate the optimization procedure. We evaluate the proposed method on the NTIRE 2022 Efficient Image Super-Resolution Challenge and achieve a good trade-off between latency and accuracy. Particularly, under the condition of limited inference time (≤ 49.42ms) and parameter amount (≤ 0.894M), our solution obtains the best fidelity results in terms of PSNR, i.e., 29.05dB and 28.75dB on the DIV2K validation and test sets, respectively.
更多查看译文
关键词
collapsible linear block,fast image super-resolution,accurate single image super-resolution,hand-crafted designs,coarse-grained pruning,network acceleration,pruned network,inference stage,training-time,inference-time architecture,two-stage training mechanism,NTIRE 2022 Efficient Image Super-Resolution Challenge,inference time,structural reparameterization technique
AI 理解论文
溯源树
样例
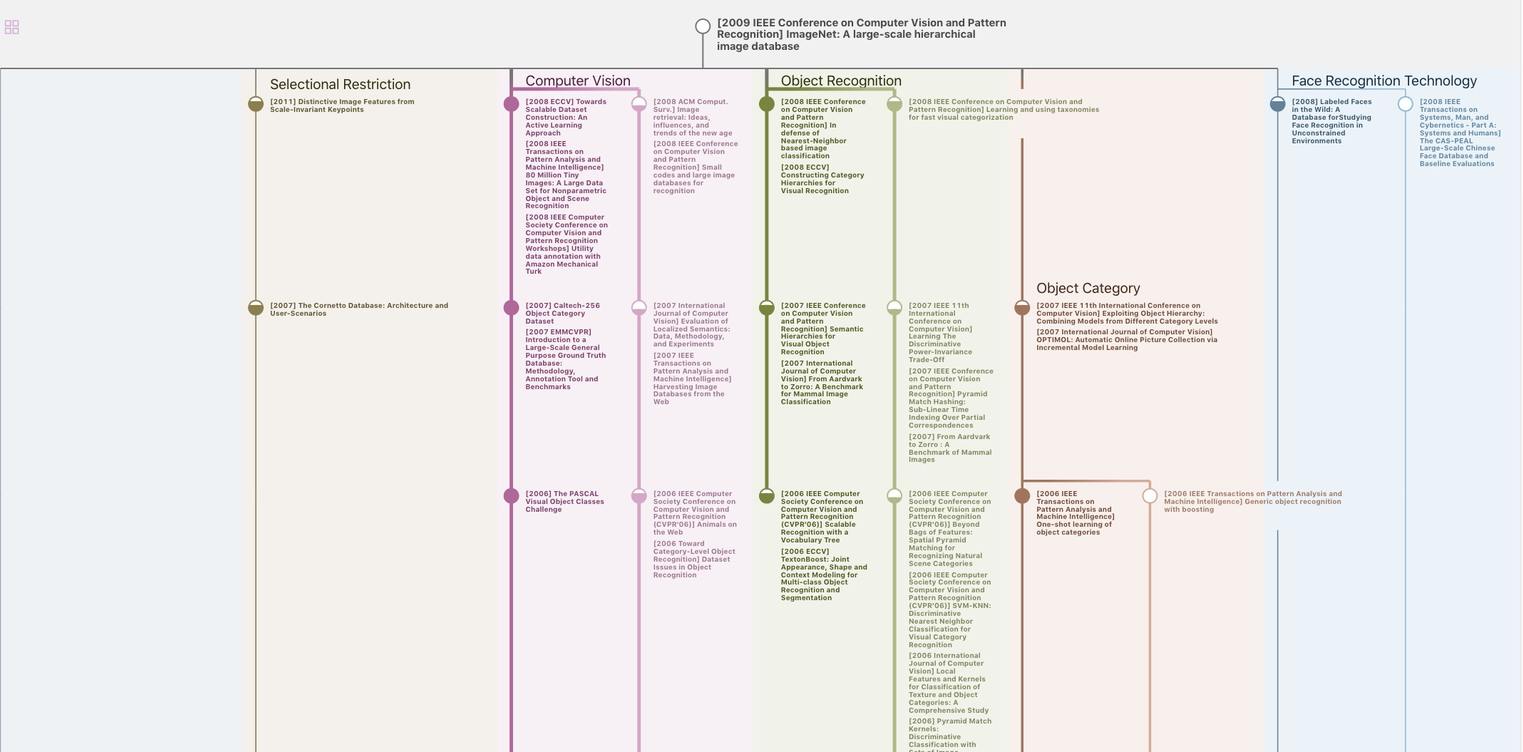
生成溯源树,研究论文发展脉络
Chat Paper
正在生成论文摘要