Data Fusion by Matrix Completion for Exposome Target Interaction Prediction
biorxiv(2022)
摘要
Background Exposure to toxic chemicals presents a huge health burden and disease risk. Key to understanding which chemicals cause specific toxic effects is determining the molecular target(s) of these chemicals. Given that over a thousand new chemicals are produced annually, it is infeasible to perform a comprehensive safety assessment for all novel chemicals due to limited resources. Thus, a robust computational method for discovering targets of environmental exposures, which can then be used to prioritize chemicals for further study, is a promising direction for public health research.
Objectives We implemented a novel matrix completion algorithm named coupled matrix-matrix completion (CMMC) for predicting exposome-target interactions.
Methods The low rank matrix completion problem can be described as predicting missing values of an incomplete un-observed/under-observed or partially observed matrix. Different from previous matrix completion algorithms based on matrix factorization, the CMMC algorithm incorporates two coupled matrices in form of auxiliary information to help better optimize the distance metric and maintains a fast runtime. The present data fusion model has the potential to automatically reveal shared and unshared components through modeling constraints. The two coupled matrices represent the relationships among the chemical exposures and gene targets, respectively, and help jointly analyze incomplete data sets. We collected and processed relevant data from the Comparative Toxicogenomics Database (CTD) as a benchmark dataset for implementing and testing our method and comparing it to alternative prediction methods.
Results Our method achieved an AUC of 0.89 on the benchmark dataset generated in this study. Compared to other state-of-the-art methods, CMMC achieved the best performance for predicting environmental chemical-gene target interactions. The case study shows that CMMC can be used to discover potential molecular targets of novel chemicals without any prior bioactivity knowledge.
Discussion Our CMMC approach is a powerful method for predicting the target genes of environmental exposures.
### Competing Interest Statement
The authors have declared no competing interest.
更多查看译文
AI 理解论文
溯源树
样例
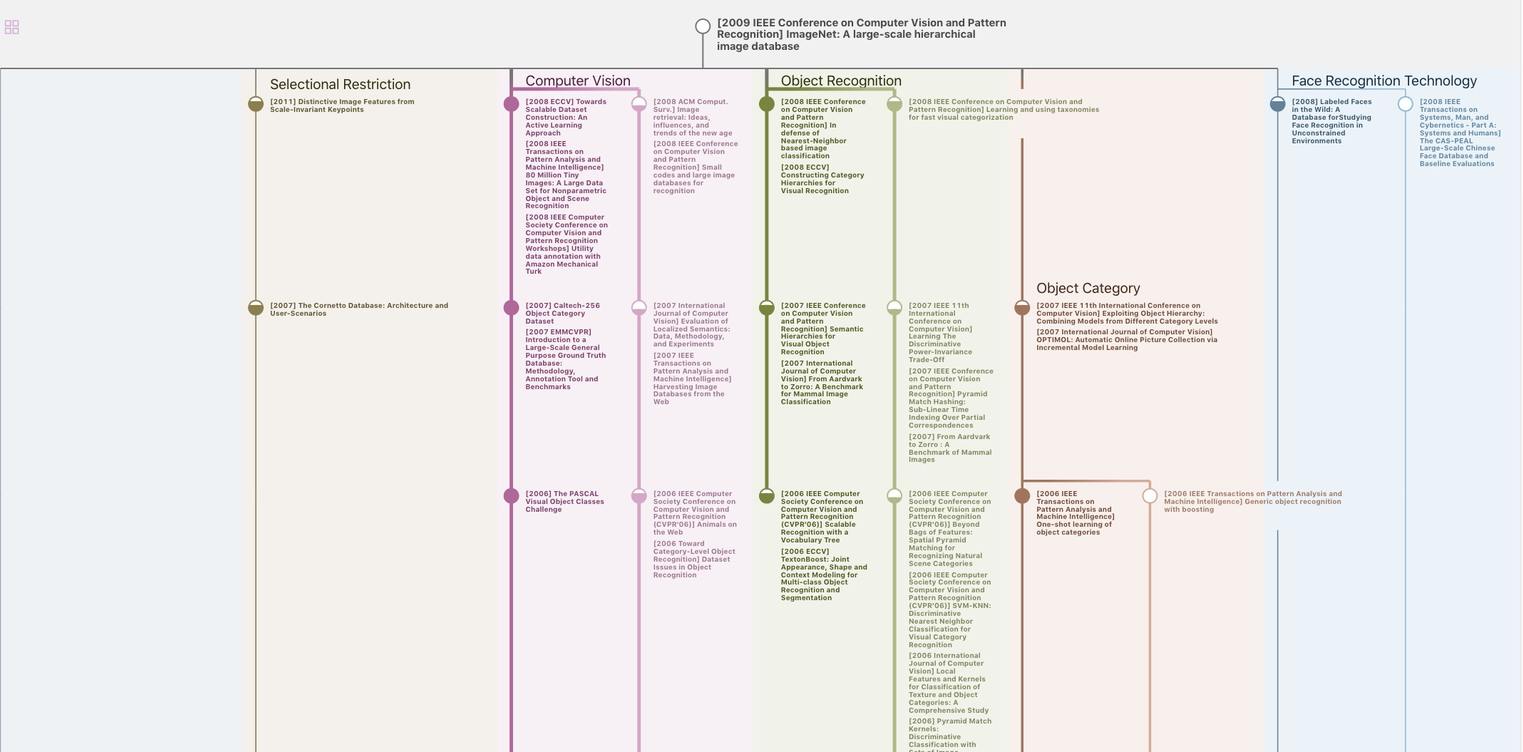
生成溯源树,研究论文发展脉络
Chat Paper
正在生成论文摘要