Detecting anomalous content in dairy with whole metagenome sequencing
biorxiv(2022)
摘要
The increasing knowledge of microbial ecology in food products relating to quality and safety as well as the established usefulness of machine learning algorithms for anomaly detection in multiple scenarios suggests that the application of microbiome data in food production systems for anomaly detection is an important next step toward bettering our food supply chain. To that end, the objective of the research presented here was to assess the feasibility of using whole metagenome shotgun sequencing data as input into anomaly detection algorithms using fluid milk as a model system. Contrastive PCA, cluster-based methods, and explainable AI were evaluated as potential methods for detection of two anomalous sample classes using longitudinal metagenomic profiling of fluid milk compared to baseline samples collected under comparable circumstances. Traditional methods (alpha and beta diversity, clustering-based contrastive PCA, MDS, and dendrograms) failed to differentiate anomalous sample classes; however, explainable AI was able to classify anomalous vs. baseline samples and indicate microbial drivers in association with antibiotic use e.g., Morganella and Enterobacter and microbial signatures related to milk collected from an alternative farm e.g., Coxiella. This work also characterizes the microbiome of fluid milk in greater depth than previously published studies. Our results indicate the application of artificial intelligence continues to hold promise in the realm of microbiome data analysis and could present further opportunity for downstream analytic automation to aid in food safety and quality.
IMPORTANCE We evaluated the feasibility of using untargeted metagenomic se-quencing of raw milk for detecting anomalous food ingredient content with artificial intelligence methods. The approach could potentially be applied in the food industry for safety and quality control.
更多查看译文
关键词
whole metagenome,dairy,anomalous content
AI 理解论文
溯源树
样例
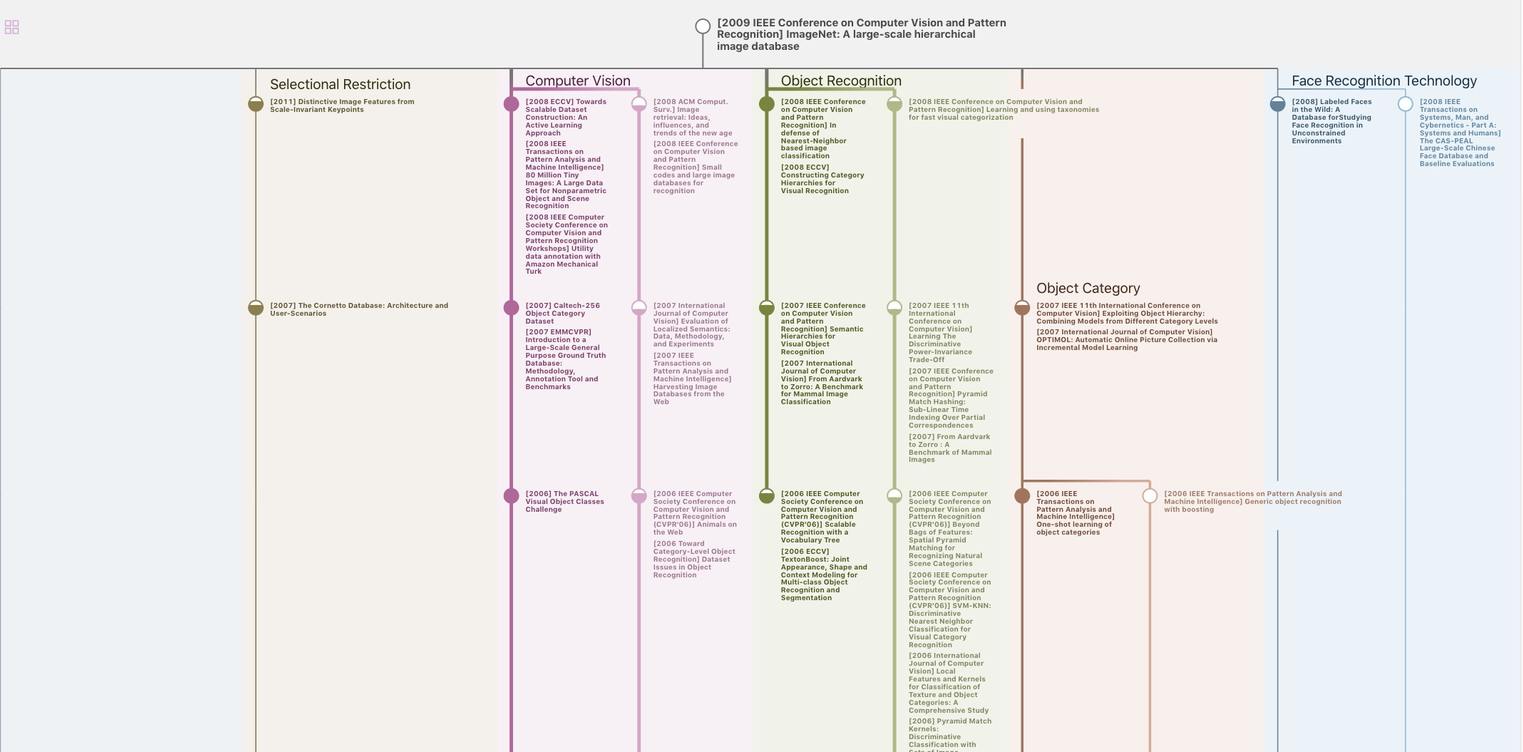
生成溯源树,研究论文发展脉络
Chat Paper
正在生成论文摘要