Protein engineering via Bayesian optimization -guided evolutionary algorithm and robotic experiments
BRIEFINGS IN BIOINFORMATICS(2023)
摘要
Directed protein evolution applies repeated rounds of genetic mutagenesis and phenotypic screening and is often limited by experimental throughput. Through in silico prioritization of mutant sequences, machine learning has been applied to reduce wet lab burden to a level practical for human researchers. On the other hand, robotics permits large batches and rapid iterations for protein engineering cycles, but such capacities have not been well exploited in existing machine learning-assisted directed evolution approaches. Here, we report a scalable and batched method, Bayesian Optimization -guided EVOlutionary (BO-EVO) algorithm, to guide multiple rounds of robotic experiments to explore protein fitness landscapes of combinatorial mutagenesis libraries. We first examined various design specifications based on an empirical landscape of protein G domain B1. Then, BO-EVO was successfully generalized to another empirical landscape of an Escherichia coli kinase PhoQ as well as simulated NK landscapes with up to moderate epistasis. This approach was then applied to guide robotic library creation and screening to engineer enzyme specificity of Rh1A, a key biosynthetic enzyme for rhamnolipid biosurfactants. A 4.8 -fold improvement in producing a target rhamnolipid congener was achieved after examining less than 1% of all possible mutants after four iterations. Overall, BO-EVO proves to be an efficient and general approach to guide combinatorial protein engineering without prior knowledge.
更多查看译文
关键词
adaptive experimental design,Bayesian optimization,evolutionary algorithm,machine learning,directed evolution
AI 理解论文
溯源树
样例
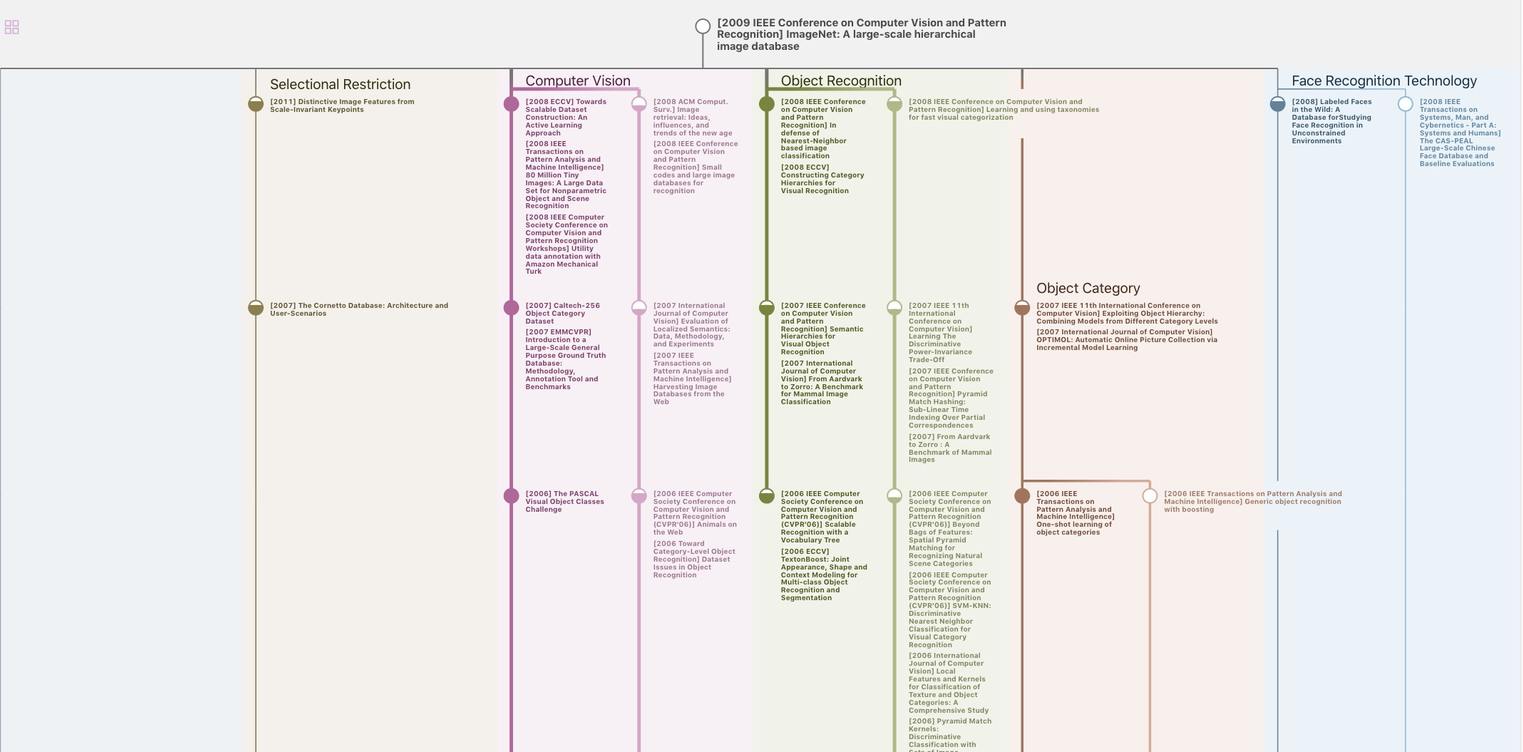
生成溯源树,研究论文发展脉络
Chat Paper
正在生成论文摘要