Transcriptomic forecasting with neural ODEs
biorxiv(2022)
摘要
Single-cell RNA-seq (scRNA-seq) technologies can uncover changes in the molecular states that underlie cellular phenotypes. However, to understand dynamic cellular processes, computational tools are needed to extract temporal information from the snapshots of cellular gene expression that scRNA-seq provides. To address this challenge, we have developed a neural ordinary differential equation based method, RNAForecaster, for predicting gene expression states in single cells for multiple future time steps. We demonstrate that RNAForecaster can accurately predict future expression states in simulated scRNA-seq data. We then show that using metabolic labeling scRNA-seq data from constitutively dividing cells, RNAForecaster accurately recapitulates many of the expected changes in gene expression during progression through the cell cycle over a three day period. Finally, we extend RNAForecaster to use unspliced and spliced counts from scRNA-seq to predict the impact of knock down experiments in pancreatic development. Thus, RNAForecaster enables estimation of future expression states in biological systems.
### Competing Interest Statement
Elana J. Fertig is on the Scientific Advisory Board of Resistance Bio / Viosera Therapeutics and a consultant for Mestag Therapeutics and Merck, and the other authors have no competing interests to declare in regards to this manuscript
更多查看译文
AI 理解论文
溯源树
样例
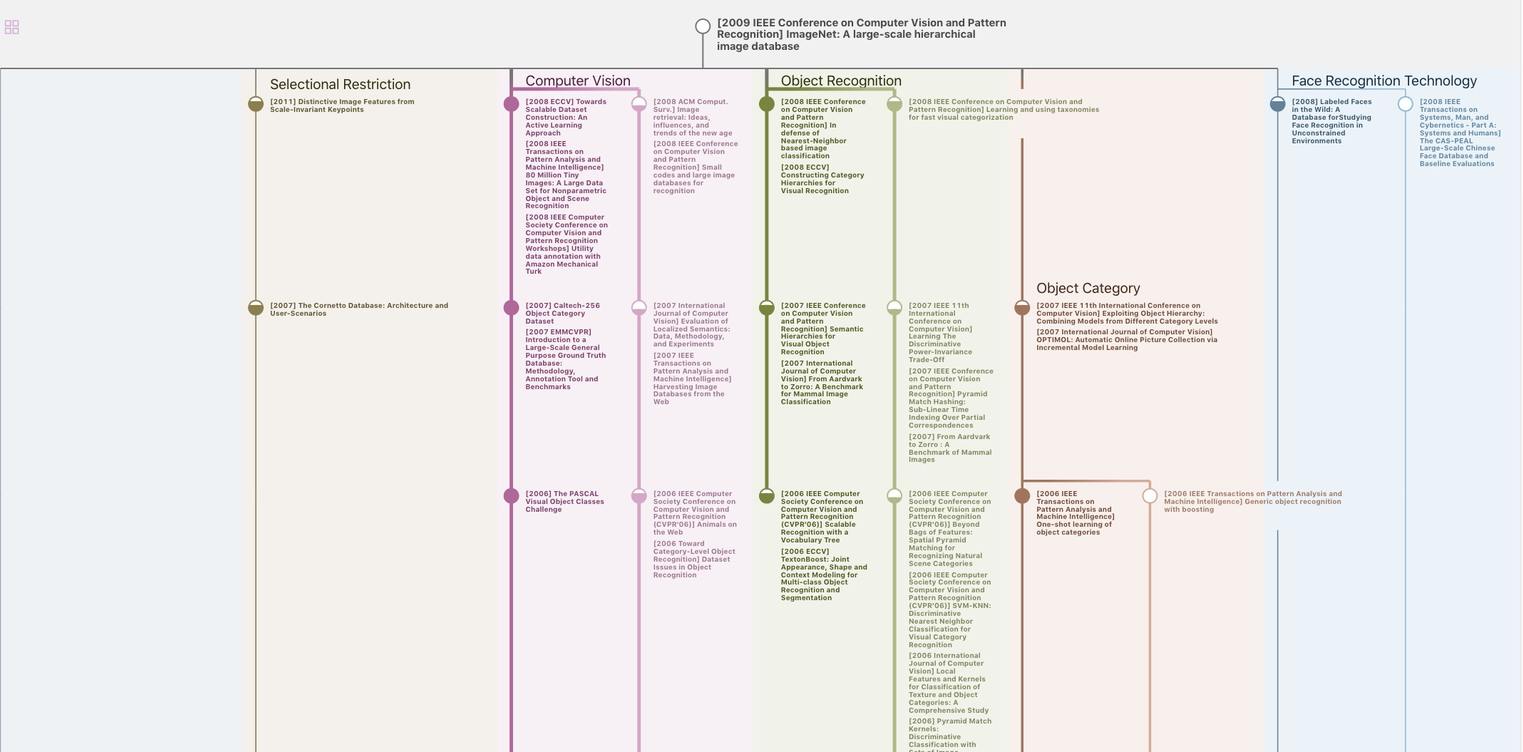
生成溯源树,研究论文发展脉络
Chat Paper
正在生成论文摘要