Case Studies for Computing Density of Reachable States for Safe Autonomous Motion Planning
NASA Formal Methods (NFM)(2022)
摘要
Density of the reachable states can help understand the risk of safety-critical systems, especially in situations when worst-case reachability is too conservative. Recent work provides a data-driven approach to compute the density distribution of autonomous systems' forward reachable states online. In this paper, we study the use of such approach in combination with model predictive control for verifiable safe path planning under uncertainties. We first use the learned density distribution to compute the risk of collision online. If such risk exceeds the acceptable threshold, our method will plan for a new path around the previous trajectory, with the risk of collision below the threshold. Our method is well-suited to handle systems with uncertainties and complicated dynamics as our data-driven approach does not need an analytical form of the systems' dynamics and can estimate forward state density with an arbitrary initial distribution of uncertainties. We design two challenging scenarios (autonomous driving and hovercraft control) for safe motion planning in environments with obstacles under system uncertainties. We first show that our density estimation approach can reach a similar accuracy as the Monte-Carlo-based method while using only 0.01X training samples. By leveraging the estimated risk, our algorithm achieves the highest success rate in goal reaching when enforcing the safety rate above 0.99.
更多查看译文
关键词
Reachability analysis,State density estimation,Online planning,Liouville Theorem,Neural network
AI 理解论文
溯源树
样例
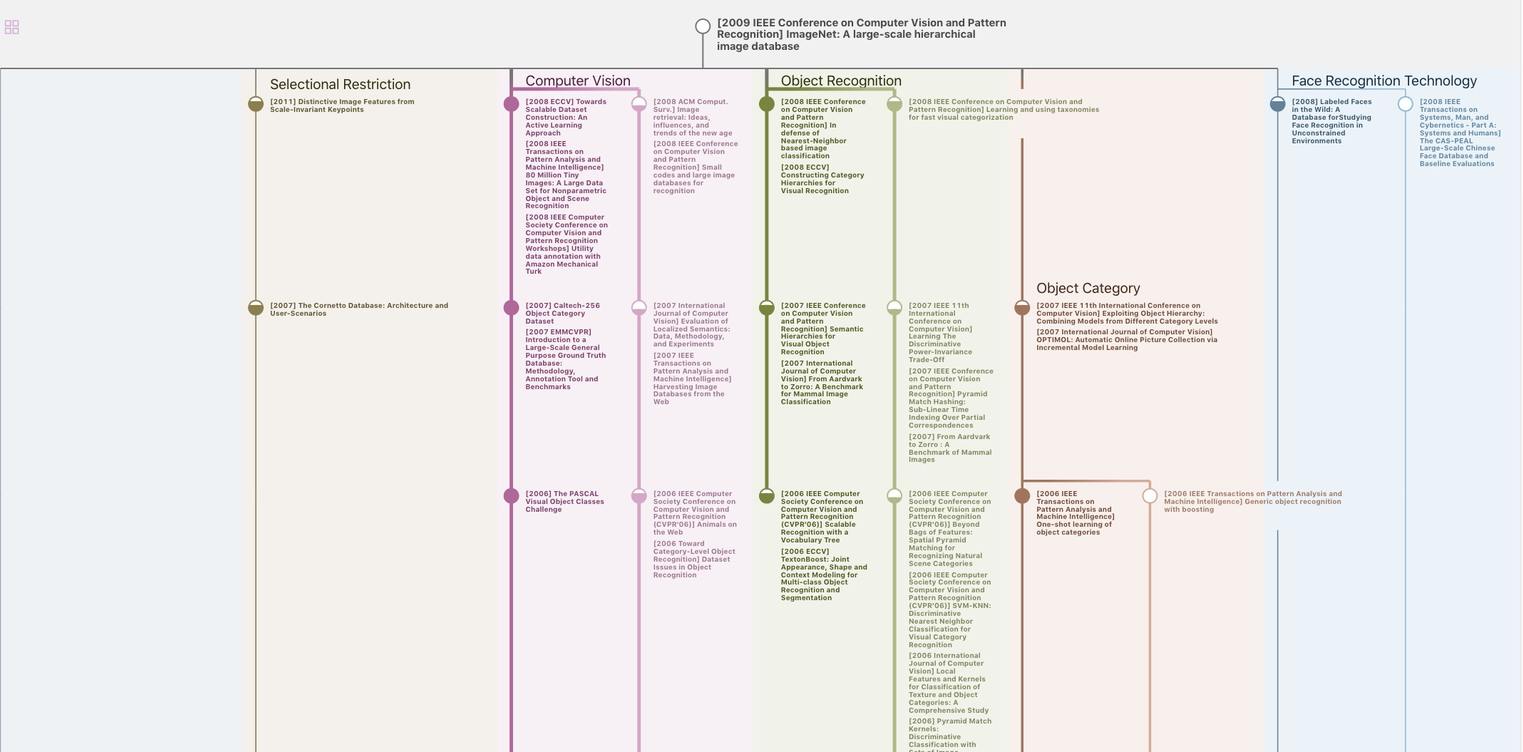
生成溯源树,研究论文发展脉络
Chat Paper
正在生成论文摘要