Self-Supervised Learning of Phenotypic Representations from Cell Images with Weak Labels
arxiv(2022)
摘要
We propose WS-DINO as a novel framework to use weak label information in learning phenotypic representations from high-content fluorescent images of cells. Our model is based on a knowledge distillation approach with a vision transformer backbone (DINO), and we use this as a benchmark model for our study. Using WS-DINO, we fine-tuned with weak label information available in high-content microscopy screens (treatment and compound) and achieve state-of-the-art performance in not-same-compound mechanism of action prediction on the BBBC021 dataset (98%), and not-same-compound-and-batch performance (96%) using the compound as the weak label. Our method bypasses single cell cropping as a pre-processing step, and using self-attention maps we show that the model learns structurally meaningful phenotypic profiles.
更多查看译文
关键词
cell images
AI 理解论文
溯源树
样例
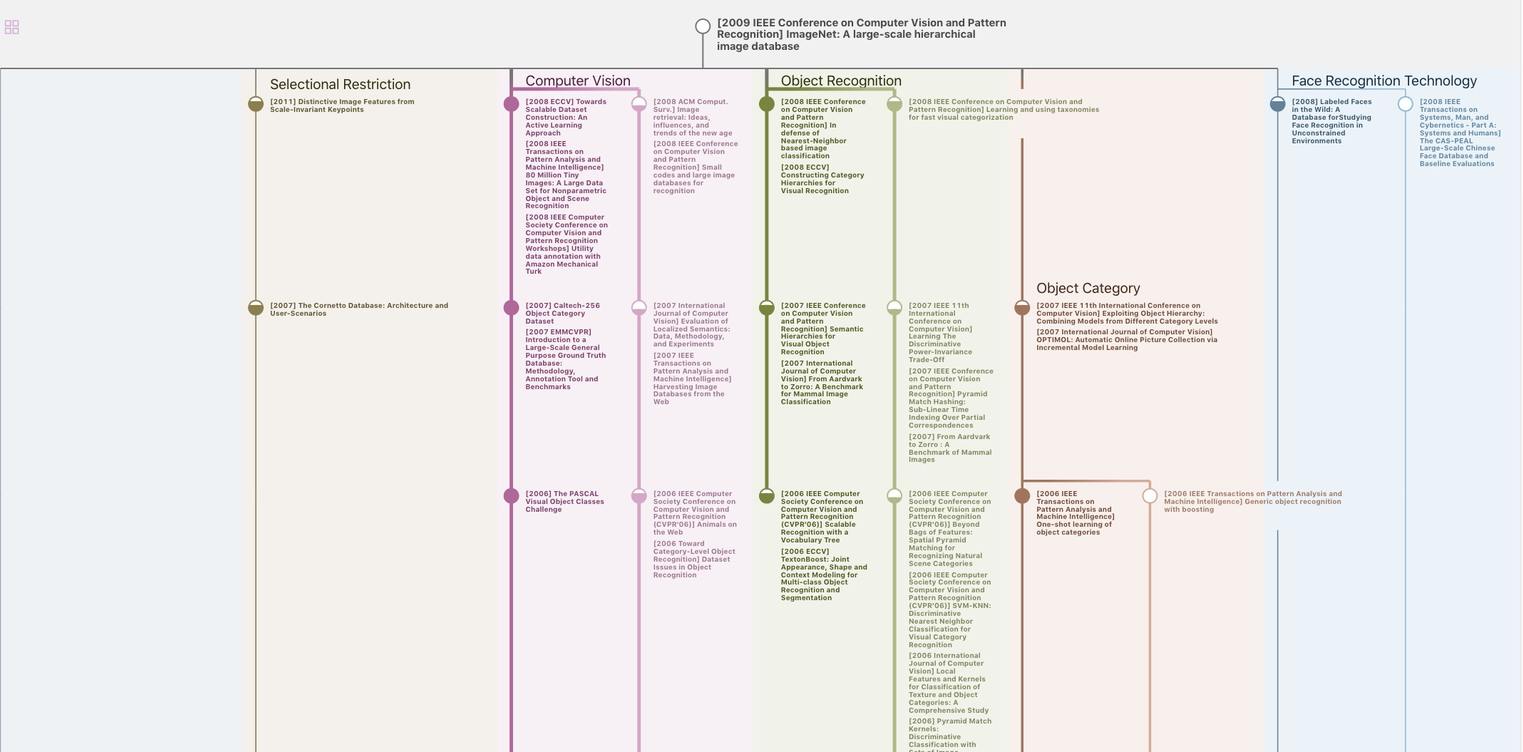
生成溯源树,研究论文发展脉络
Chat Paper
正在生成论文摘要