Neural Koopman Control Barrier Functions for Safety-Critical Control of Unknown Nonlinear Systems
2023 AMERICAN CONTROL CONFERENCE, ACC(2023)
摘要
We consider the problem of synthesis of safe controllers for nonlinear systems with unknown dynamics using Control Barrier Functions (CBF). We utilize Koopman operator theory (KOT) to associate the (unknown) nonlinear system with a higher dimensional bilinear system and propose a datadriven learning framework that uses a learner and a falsifier to simultaneously learn the Koopman operator based bilinear system and a corresponding CBF. We prove that the learned CBF for the latter bilinear system is also a CBF for the unknown nonlinear system by characterizing the l(2)-norm error bound between these two systems. We show that this error can be partially tuned by using the Lipschitz constant of the Koopman based observables. The CBF is then used to formulate a quadratic program to compute inputs that guarantee safety of the unknown nonlinear system. Numerical simulations are presented to validate our approach.
更多查看译文
关键词
Computational methods,Modeling,Robotics
AI 理解论文
溯源树
样例
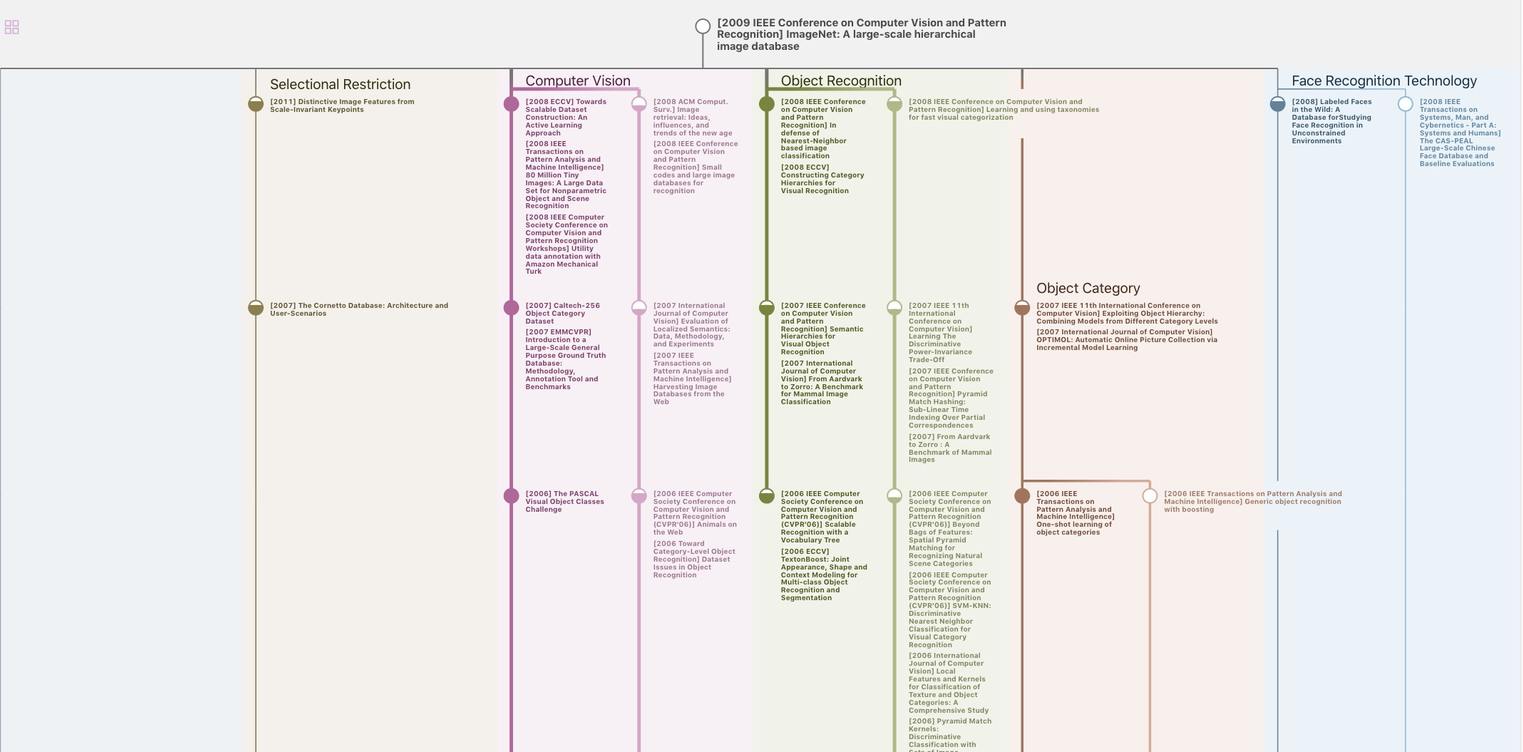
生成溯源树,研究论文发展脉络
Chat Paper
正在生成论文摘要