On the Relation between Sensitivity and Accuracy in In-Context Learning.
arxiv(2023)
摘要
In-context learning (ICL) suffers from oversensitivity to the prompt, making it unreliable in real-world scenarios. We study the sensitivity of ICL with respect to multiple perturbation types. First, we find that label bias obscures the true sensitivity, and therefore prior work may have significantly underestimated ICL sensitivity. Second, we observe a strong negative correlation between ICL sensitivity and accuracy: predictions sensitive to perturbations are less likely to be correct. Motivated by these findings, we propose \textsc{SenSel}, a few-shot selective prediction method that abstains from sensitive predictions. Experiments on ten classification datasets show that \textsc{SenSel} consistently outperforms two commonly used confidence-based and entropy-based baselines on abstention decisions.
更多查看译文
关键词
sensitivity,accuracy,learning,in-context
AI 理解论文
溯源树
样例
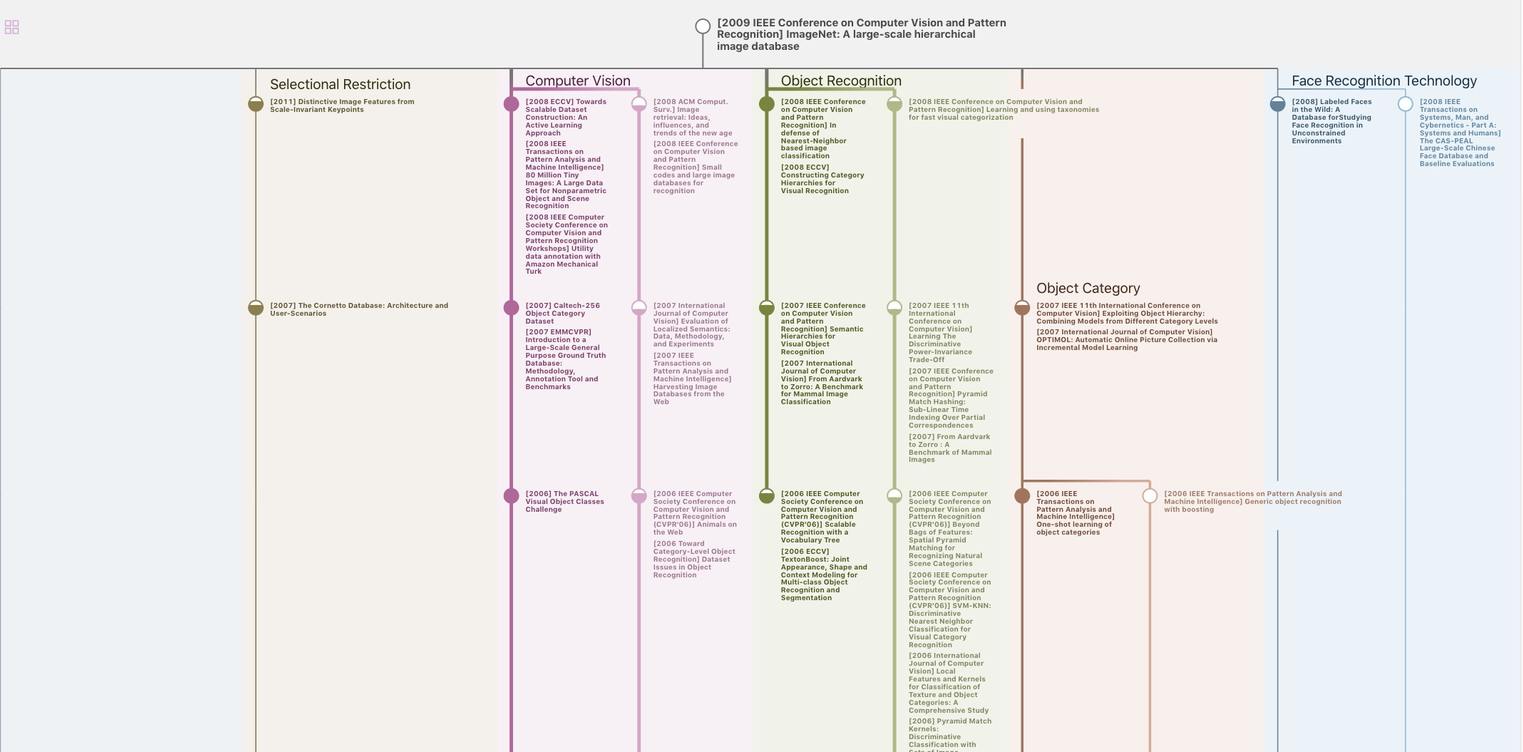
生成溯源树,研究论文发展脉络
Chat Paper
正在生成论文摘要