REDIRECTION: Generating drug repurposing hypotheses using link prediction with DISNET data
2022 IEEE 35th International Symposium on Computer-Based Medical Systems (CBMS)(2022)
摘要
In recent years and due to COVID-19 pandemic, drug repurposing or repositioning has been placed in the spotlight. Giving new therapeutic uses to already existing drugs, this discipline allows to streamline the drug discovery process, reducing the costs and risks inherent to de novo development. Computational approaches have gained momentum, and emerging techniques from the machine learning domain have proved themselves as highly exploitable means for repurposing prediction. Against this backdrop, one can find that biomedical data can be represented in terms of graphs, which allow depicting in a very expressive manner the underlying structure of the information. Combining these graph data structures with deep learning models enhances the prediction of new links, such as potential disease-drug connections. In this paper, we present a new model named REDIRECTION, which aims to predict new disease-drug links in the context of drug repurposing. It has been trained with a part of the DISNET biomedical graph, formed by diseases, symptoms, drugs, and their relationships. The reserved testing graph for the evaluation has yielded to an AUROC of 0.93 and an AUPRC of 0.90. We have performed a secondary validation of REDIRECTION using RepoDB data as the testing set, which has led to an AUROC of 0.87 and a AUPRC of 0.83. In the light of these results, we believe that REDIRECTION can be a meaningful and promising tool to generate drug repurposing hypotheses.
更多查看译文
关键词
Drug repurposing,Drug repositioning,Graph deep learning,Graph Neural Networks,DISNET knowledge base
AI 理解论文
溯源树
样例
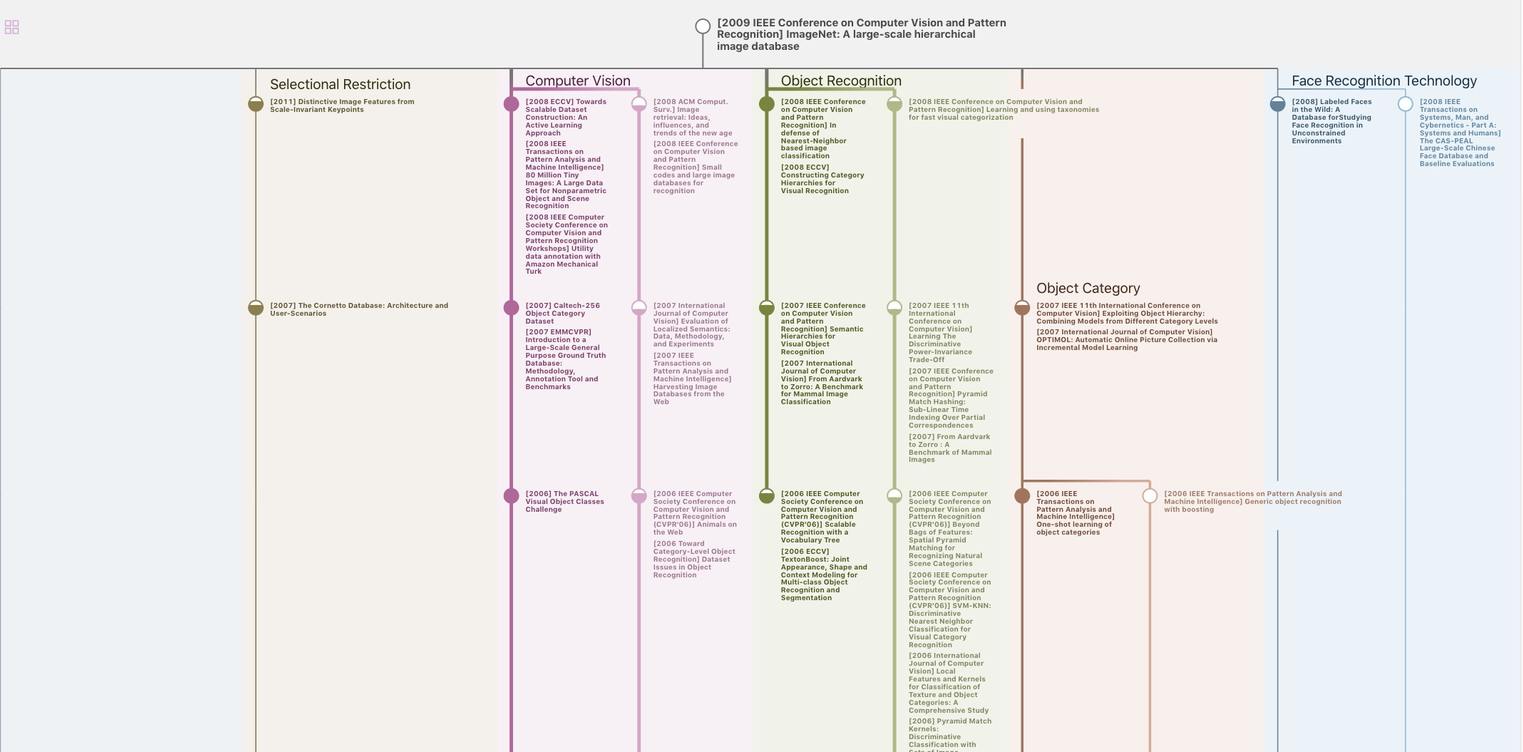
生成溯源树,研究论文发展脉络
Chat Paper
正在生成论文摘要