Machine Learning Electroencephalography Biomarkers Predictive of Epworth Sleepiness Scale
biorxiv(2022)
摘要
Excessive daytime sleepiness (EDS) causes difficulty in concentrating and continuous fatigue during the day. In a clinical setting, the assessment and diagnosis of EDS relies mostly on subjective questionnaires and verbal reports, which compromises the effectiveness of available therapies. In this study, we used a computational pipeline for the automated, rapid, high-throughput and objective analysis of retrospective encephalography (EEG) data to develop objective, surrogate biomarkers for EDS, thereby defining the quantitative EEG changes in individuals with High Epworth Sleepiness Scale (ESS) (n=31), compared to a group of individuals with Low ESS (n=41) at Cleveland Clinic. Signal processing of EEG showed significantly different EEG features in the Low ESS group compared to High ESS, including power enhancement in the alpha and beta bands, and attenuation in the delta and theta bands. Moreover, machine learning algorithms trained on the binary classification of High vs Low ESS reached >80% accuracy. These results demonstrate that EEG data contain information in the form of rhythmic activity that could be leveraged for the quantitative assessment of EDS using machine learning.
### Competing Interest Statement
The authors have declared no competing interest.
更多查看译文
关键词
biomarkers
AI 理解论文
溯源树
样例
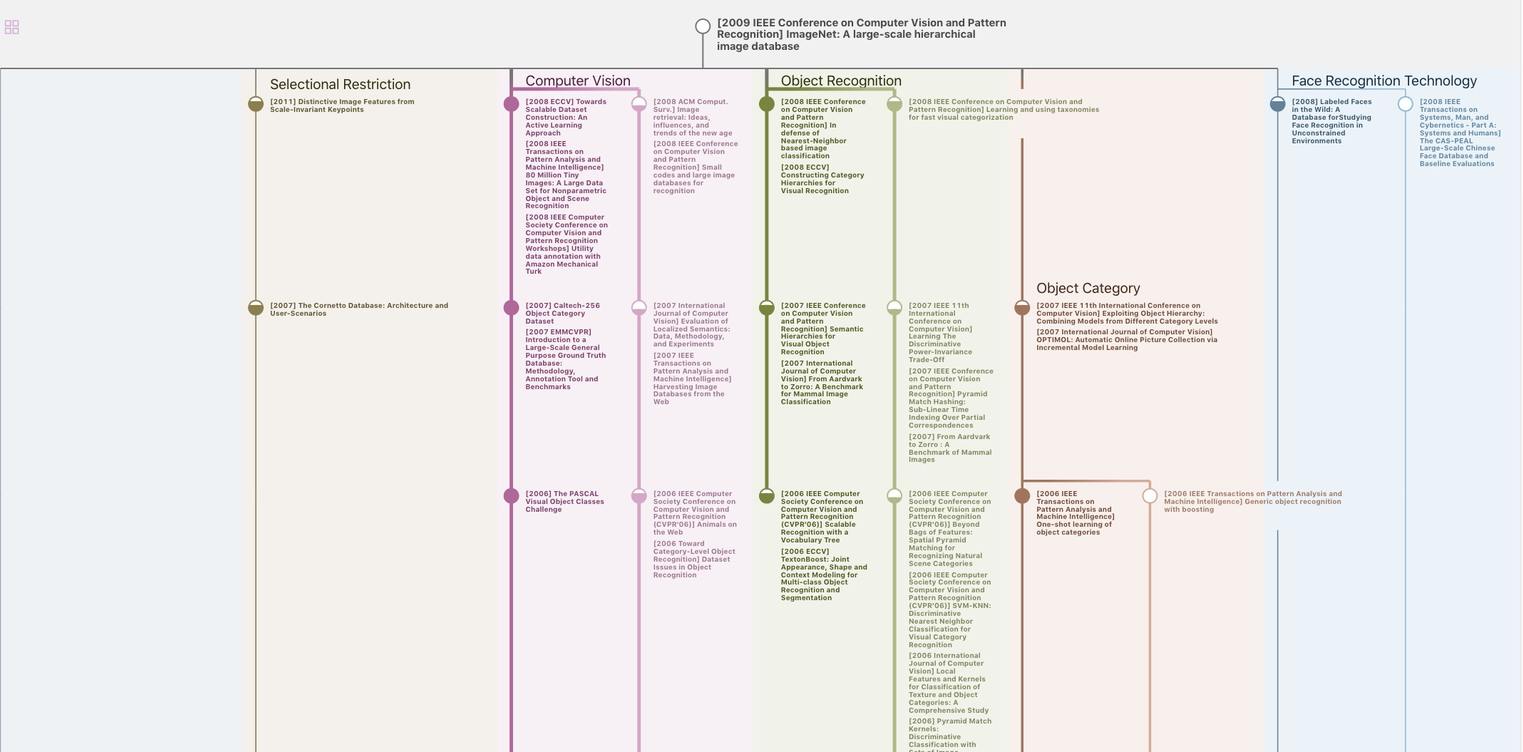
生成溯源树,研究论文发展脉络
Chat Paper
正在生成论文摘要