Deep learning image reconstruction to improve accuracy of iodine quantification and image quality in dual-energy CT of the abdomen: a phantom and clinical study
European radiology(2022)
摘要
Objectives To investigate the effect of deep learning image reconstruction (DLIR) on the accuracy of iodine quantification and image quality of dual-energy CT (DECT) compared to that of other reconstruction algorithms in a phantom experiment and an abdominal clinical study. Methods An elliptical phantom with five different iodine concentrations (1–12 mgI/mL) was imaged five times with fast-kilovoltage-switching DECT for three target volume CT dose indexes. All images were reconstructed using filtered back-projection, iterative reconstruction (two levels), and DLIR algorithms. Measured and nominal iodine concentrations were compared among the algorithms. Contrast-enhanced CT of the abdomen with the same scanner was acquired in clinical patients. In arterial and portal venous phase images, iodine concentration, image noise, and coefficients of variation for four locations were retrospectively compared among the algorithms. One-way repeated-measures analyses of variance were used to evaluate differences in the iodine concentrations, standard deviations, coefficients of variation, and percentages of error among the algorithms. Results In the phantom study, the measured iodine concentrations were equivalent among the algorithms: within ± 8% of the nominal values, with root-mean-square deviations of 0.08–0.36 mgI/mL, regardless of radiation dose. In the clinical study (50 patients; 35 men; mean age, 68 ± 11 years), iodine concentrations were equivalent among the algorithms for each location (all p > .99). Image noise and coefficients of variation were lower with DLIR than with the other algorithms (all p < .01). Conclusions The DLIR algorithm reduced image noise and variability of iodine concentration values compared with other reconstruction algorithms in the fast-kilovoltage-switching dual-energy CT. Key Points • In the phantom study, standard deviations and coefficients of variation in iodine quantification were lower on images with the deep learning image reconstruction algorithm than on those with other algorithms . • In the clinical study, iodine concentrations of measurement location in the upper abdomen were consistent across four reconstruction algorithms, while image noise and variability of iodine concentrations were lower on images with the deep learning image reconstruction algorithm .
更多查看译文
关键词
Multidetector computed tomography,Deep learning,Quantitative evaluation,Iodine
AI 理解论文
溯源树
样例
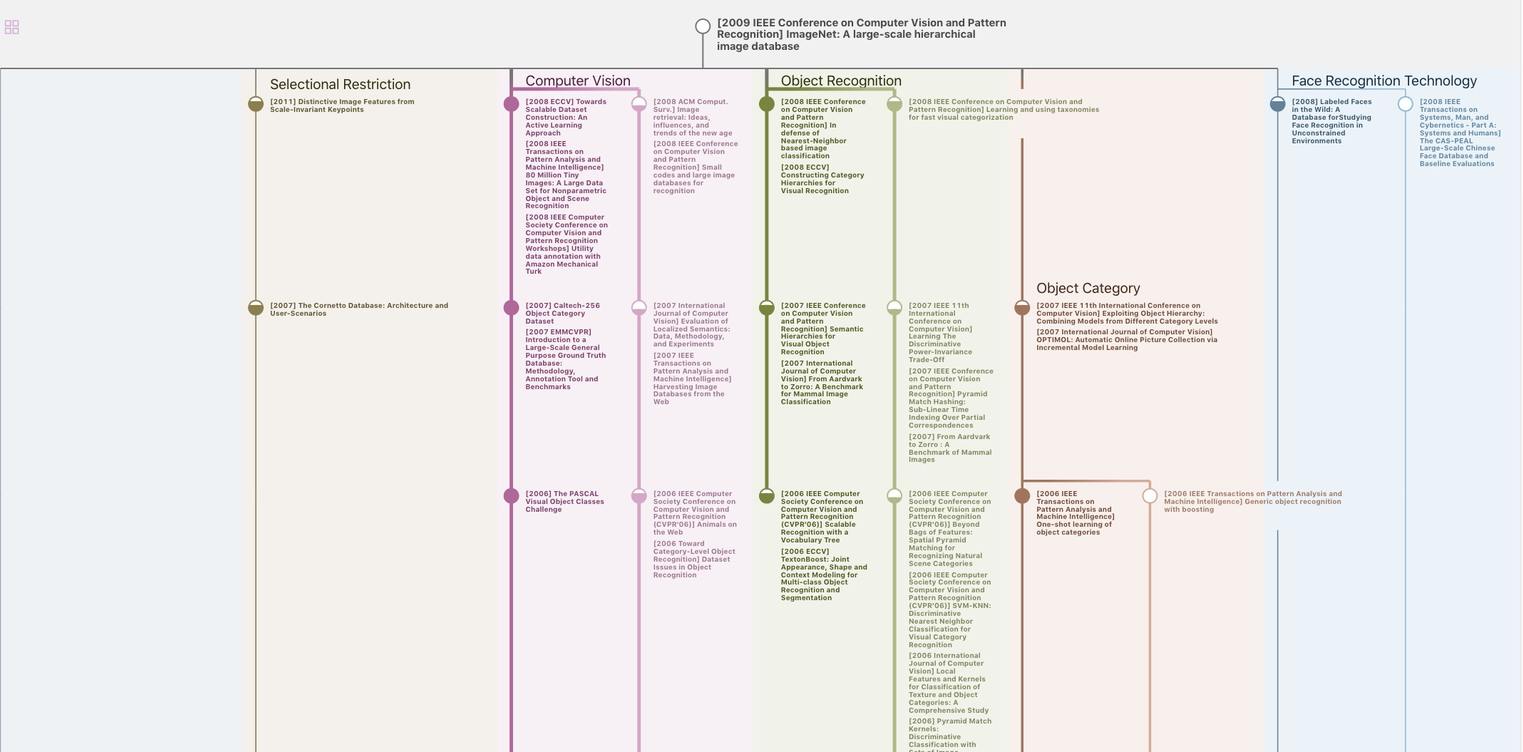
生成溯源树,研究论文发展脉络
Chat Paper
正在生成论文摘要