Cost-Effectiveness and Value-of-Information Analysis Using Machine Learning-Based Metamodeling: A Case of Hepatitis C Treatment
Medical decision making : an international journal of the Society for Medical Decision Making(2023)
摘要
Background Metamodels can address some of the limitations of complex simulation models by formulating a mathematical relationship between input parameters and simulation model outcomes. Our objective was to develop and compare the performance of a machine learning (ML)-based metamodel against a conventional metamodeling approach in replicating the findings of a complex simulation model. Methods We constructed 3 ML-based metamodels using random forest, support vector regression, and artificial neural networks and a linear regression-based metamodel from a previously validated microsimulation model of the natural history hepatitis C virus (HCV) consisting of 40 input parameters. Outcomes of interest included societal costs and quality-adjusted life-years (QALYs), the incremental cost-effectiveness (ICER) of HCV treatment versus no treatment, cost-effectiveness analysis curve (CEAC), and expected value of perfect information (EVPI). We evaluated metamodel performance using root mean squared error (RMSE) and Pearson's R-2 on the normalized data. Results The R-2 values for the linear regression metamodel for QALYs without treatment, QALYs with treatment, societal cost without treatment, societal cost with treatment, and ICER were 0.92, 0.98, 0.85, 0.92, and 0.60, respectively. The corresponding R-2 values for our ML-based metamodels were 0.96, 0.97, 0.90, 0.95, and 0.49 for support vector regression; 0.99, 0.83, 0.99, 0.99, and 0.82 for artificial neural network; and 0.99, 0.99, 0.99, 0.99, and 0.98 for random forest. Similar trends were observed for RMSE. The CEAC and EVPI curves produced by the random forest metamodel matched the results of the simulation output more closely than the linear regression metamodel. Conclusions ML-based metamodels generally outperformed traditional linear regression metamodels at replicating results from complex simulation models, with random forest metamodels performing best.
更多查看译文
关键词
cost-effectiveness,machine learning,metamodels,microsimulation,sensitivity analysis,value of information analysis
AI 理解论文
溯源树
样例
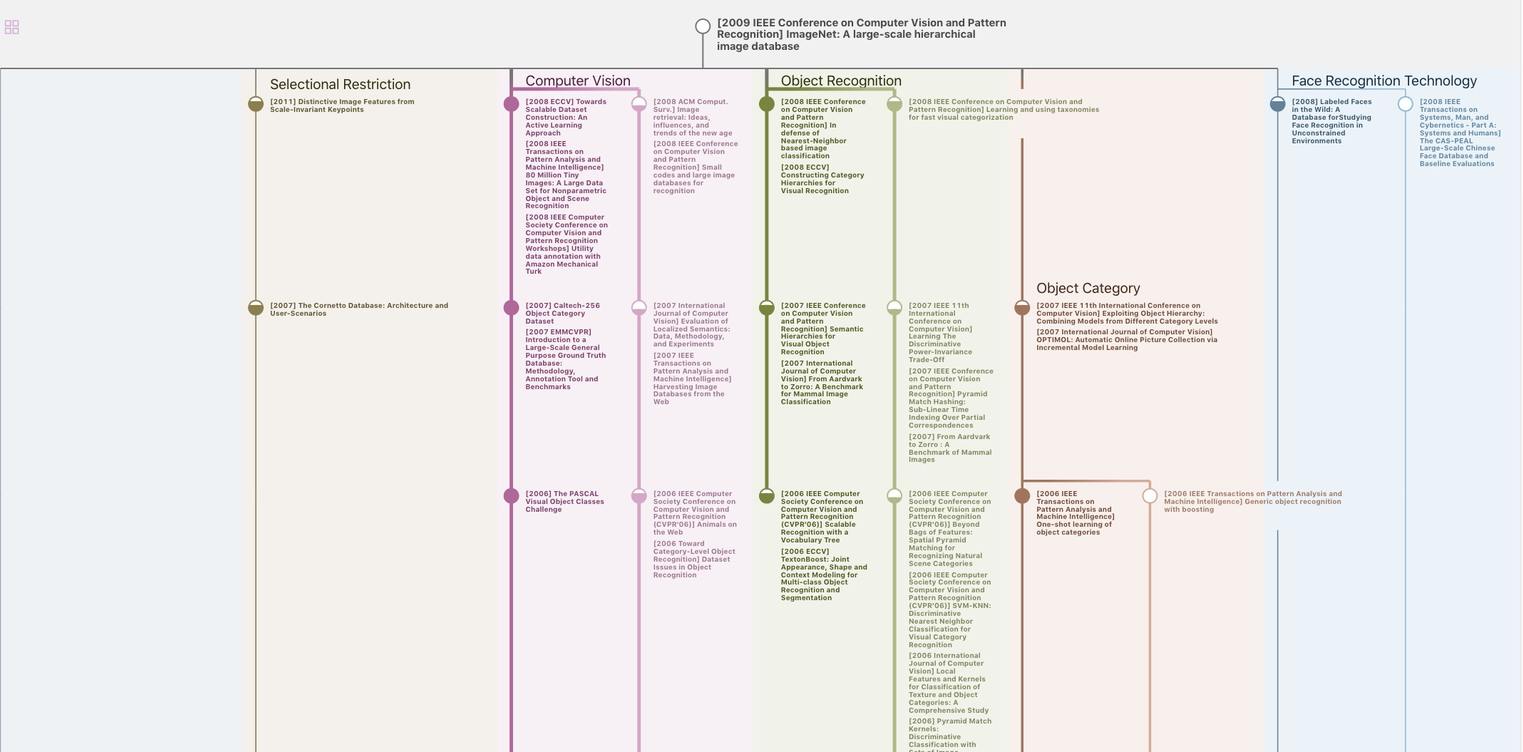
生成溯源树,研究论文发展脉络
Chat Paper
正在生成论文摘要