A meta-learning configuration framework for graph-based similarity search indexes.
Inf. Syst.(2023)
摘要
Similarity searches retrieve elements in a dataset with similar characteristics to the input query element. Recent works show that graph-based methods have outperformed others in the literature, such as tree-based and hash-based methods. However, graphs are highly parameter-sensitive for indexing and searching, which usually demands extra time for finding a suitable trade-off for specific user requirements. Current approaches to select parameters rely on observing published experimental results or Grid Search procedures. While the former has no guarantees that good settings for a dataset will also perform well on a different one, the latter is computationally expensive and limited to a small range of values. In this work, we propose a meta-learning-based recommender framework capable of providing a suitable graph configuration according to the characteristics of the input dataset. We present two instantiations of the framework: a global instantiation that uses the whole meta -database to train meta-models and a dataset-similarity-based instantiation that relies on clustering to generate meta-models tailored to datasets with similar characteristics. We also developed generic and tuned versions of the instantiations. The generic versions can satisfy user requirements in orders of magnitude faster than the traditional Grid Search. The tuned versions provide more accurate predictions at a higher cost. Our results show that the tuned methods outperform the Grid Search for most cases, providing recommendations close to the optimal one and being a suitable alternative, particularly for more challenging datasets.(c) 2022 Elsevier Ltd. All rights reserved.
更多查看译文
关键词
Similarity searching,Graph -based indexes,Parameter recommending,Meta -learning
AI 理解论文
溯源树
样例
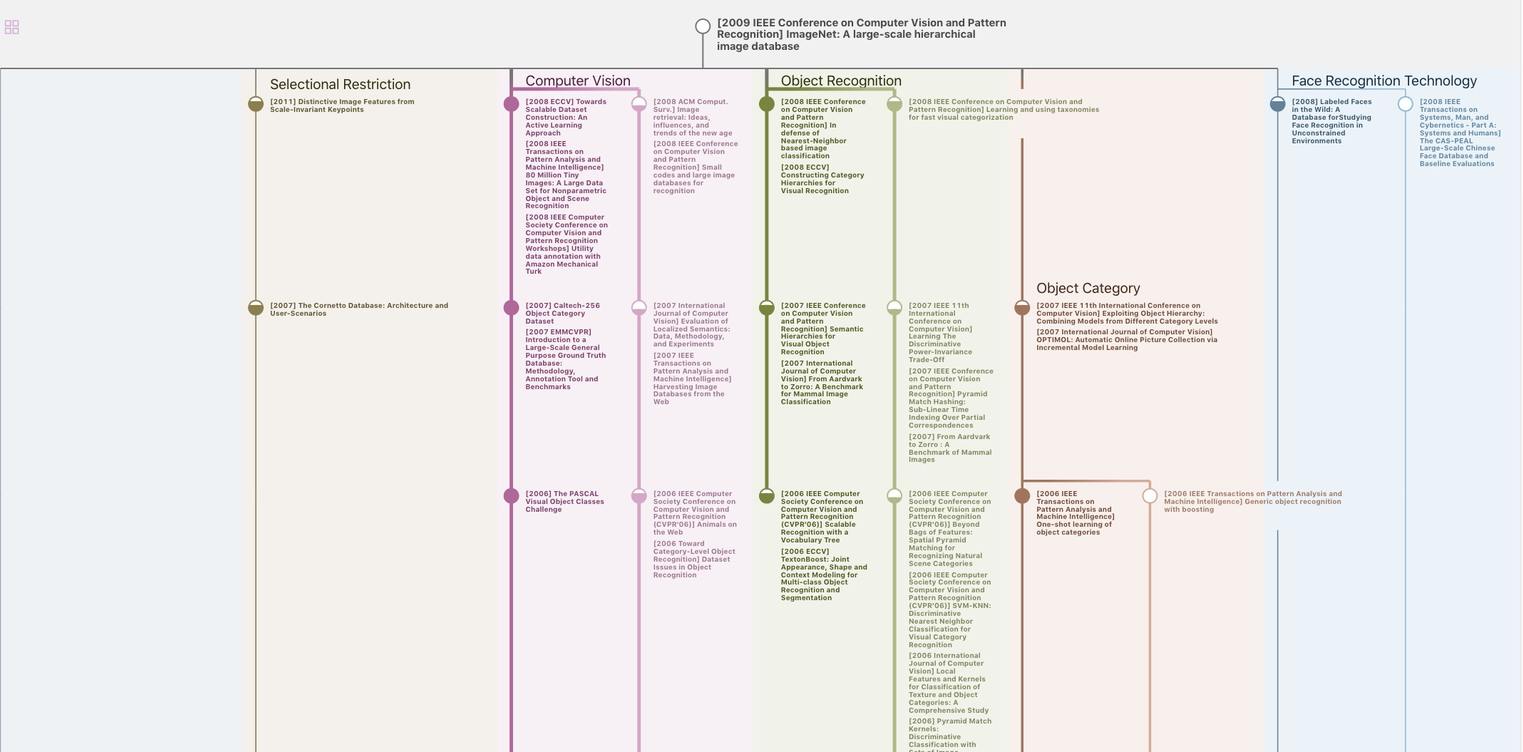
生成溯源树,研究论文发展脉络
Chat Paper
正在生成论文摘要