WiFi Fingerprinting Based Room Level Classification: Combining Short Term Fourier Transform and Imbalanced Learning Method
SIGNAL PROCESSING, SENSOR/INFORMATION FUSION, AND TARGET RECOGNITION XXXI(2022)
摘要
Mobile devices have distinct RF fingerprints, which are reflected by changes in the frequency of transmitted signals. The Short-Time Fourier Transform (STFT) is a suitable technique for evaluating this frequency content and thus identifying them. In this paper, we take advantage of STFT processing and perform room-level location classification. The raw in-phase and quadrature (IQ) signals and channel state information (CSI) frames have been collected using seven different cell phones. The data collection process has been performed in eight different locations on the same floor of our engineering building, which contains indoor hallways and rooms of different sizes. Three software-defined radios (SDRs) are placed in three different locations to receive signals simultaneously but separately. The IQ and CSI frames have been concatenated together for training a neural network. A Multi-Layer Perception (MLP) network has been used to train the concatenated signals as input and their corresponding locations as labels. A challenging aspect is that our dataset does not contain the same number of samples per location. Moreover, several locations have insufficient training data due to signal attenuation. An imbalanced learning method has been implemented in this dataset to overcome this limitation and improve the classification accuracy. The classification strategy involves binary classification like individual location vs. other. Using this approach, we obtain a mean accuracy of around 95%.
更多查看译文
关键词
STFT, WiFi fingerprinting, SDR, MLP, Imbalanced Learning
AI 理解论文
溯源树
样例
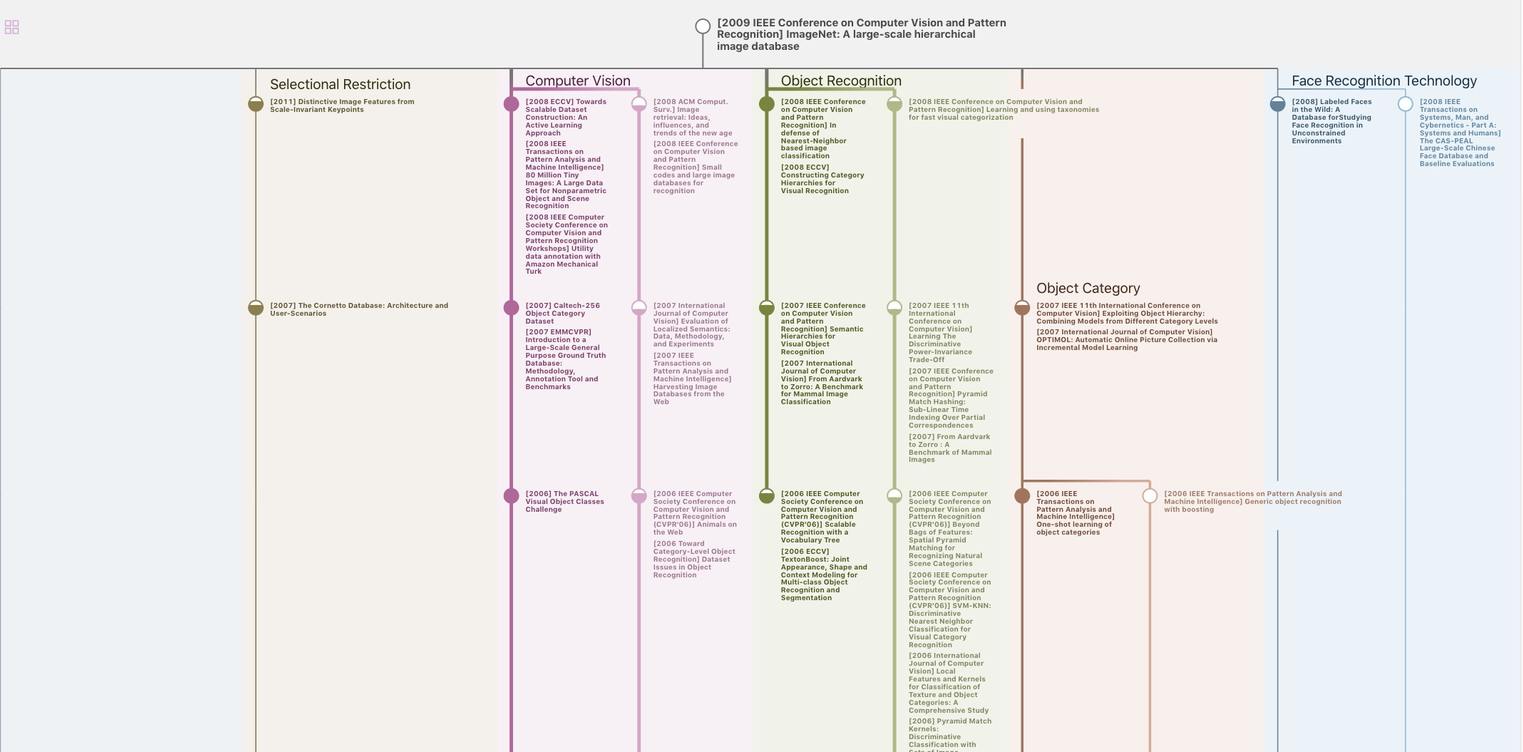
生成溯源树,研究论文发展脉络
Chat Paper
正在生成论文摘要