Sat U-Net: A Fusion Based Method for Forensic Splicing Localization in Satellite Images
MULTIMODAL IMAGE EXPLOITATION AND LEARNING 2022(2022)
摘要
Satellite images are widely available to the public. These satellite images are used in various fields including natural disaster analysis, meteorology and agriculture. As with any type of images, satellite images can be altered using image manipulation tools. A common manipulation is splicing, i.e., pasting on top of an image a region coming from a different source image. Most manipulation detection methods designed for images captured by "consumer cameras" tend to fail when used with satellite images. In this paper we propose a machine learning approach, Sat U-Net, to fuse the results of two exiting forensic splicing localization methods to increase their overall accuracy and robustness. Sat U-Net is a U-Net based architecture exploiting several Transformers to enhance the performance. Sat U-Net fuses the outputs of two unsupervised splicing detection methods, Gated PixelCNN Ensemble and Vision Transformer, to produce a heatmap highlighting the manipulated image region. We show that our fusion approach trained on images from one satellite can be lightly retrained on few images from another satellite to detect spliced regions. We compare our approach to well-known splicing detection methods (i.e., Noiseprint) and segmentation techniques (i.e., U-Net and Nested Attention U-Net). We conducted our experiments on two large datasets: one dataset contains images from Sentinel 2 satellites and the other one contains images from Worldview 3 satellite. Our experiments show that our proposed fusion method performs well when compared to other techniques in localizing spliced areas using Jaccard Index and Dice Score as metrics on both datasets.
更多查看译文
关键词
Forensic, Satellite Images, Fusion, Deep Learning
AI 理解论文
溯源树
样例
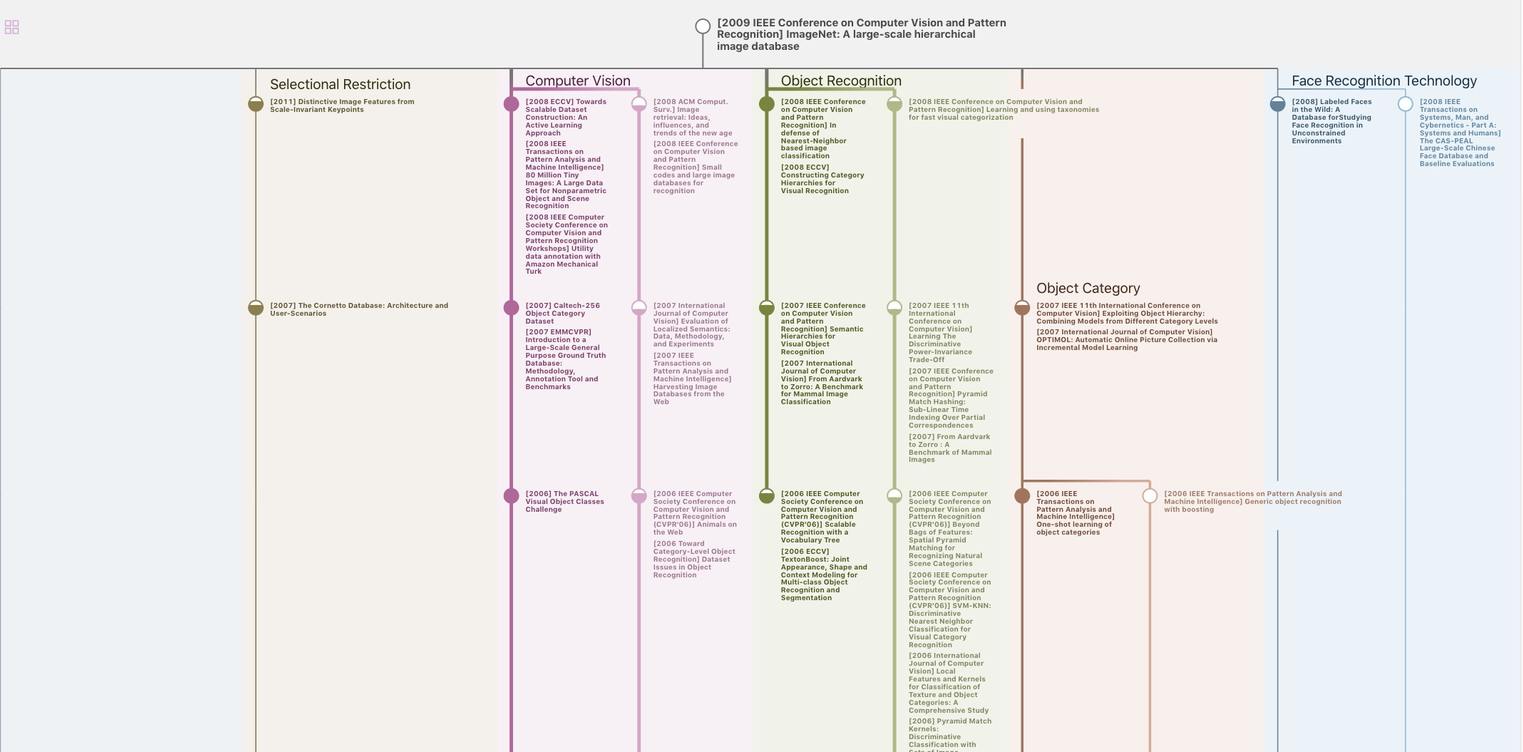
生成溯源树,研究论文发展脉络
Chat Paper
正在生成论文摘要