Efficient anomaly identification in temporal and non-temporal industrial data using tree based approaches
Applied Intelligence(2022)
摘要
Anomalies indicate impending failures in expensive industrial devices. Manufacturers of such devices or Plant Managers depend heavily on anomaly detection algorithms to perform monitoring and predictive maintenance activities. Since false alarms directly impact any industrial manufacturer’s revenue, it is crucial to reduce the number of false alarms generated by anomaly detection algorithms. Here in this paper, we have proposed multiple solutions to address this ongoing problem in the industry. The proposed unsupervised solution, Multi-Generations Tree (MGTree) algorithm, not only reduced the false positive alarms but is also equally effective on small and large datasets. MGTree has been applied to multiple industrial datasets such as Yahoo, AWS, GE, and machine sensors for evaluation purposes. Our empirical evaluation shows that MGTree performs favorably to Isolation Forest (iForest), One Class Support Vector Machine (OCSVM), and Elliptic Envelope in terms of correctness of the identification (True-Positive and False-Positive) of the anomalies. We have also proposed a time series prediction algorithm Weighted Time-Window Moving Estimation (WTM), which does not rely on the dataset’s stationary characteristics and is evaluated on multiple time-series datasets. The hybrid combination of WTM and MGTree, Uni-variate Multi-Generations Tree (UVMGTree) worked very well in anomaly identification of the time series datasets and outperformed OCSVM, iForest, SARIMA, and Elliptic Envelope. Our approach can have a profound impact on the predictive maintenance and health monitoring of the industrial systems across the domains where the operations team can save significant time and effort in handling false alarms. .
更多查看译文
关键词
Anomaly, Clustering, Time-series, Binary tree, Multi-generations
AI 理解论文
溯源树
样例
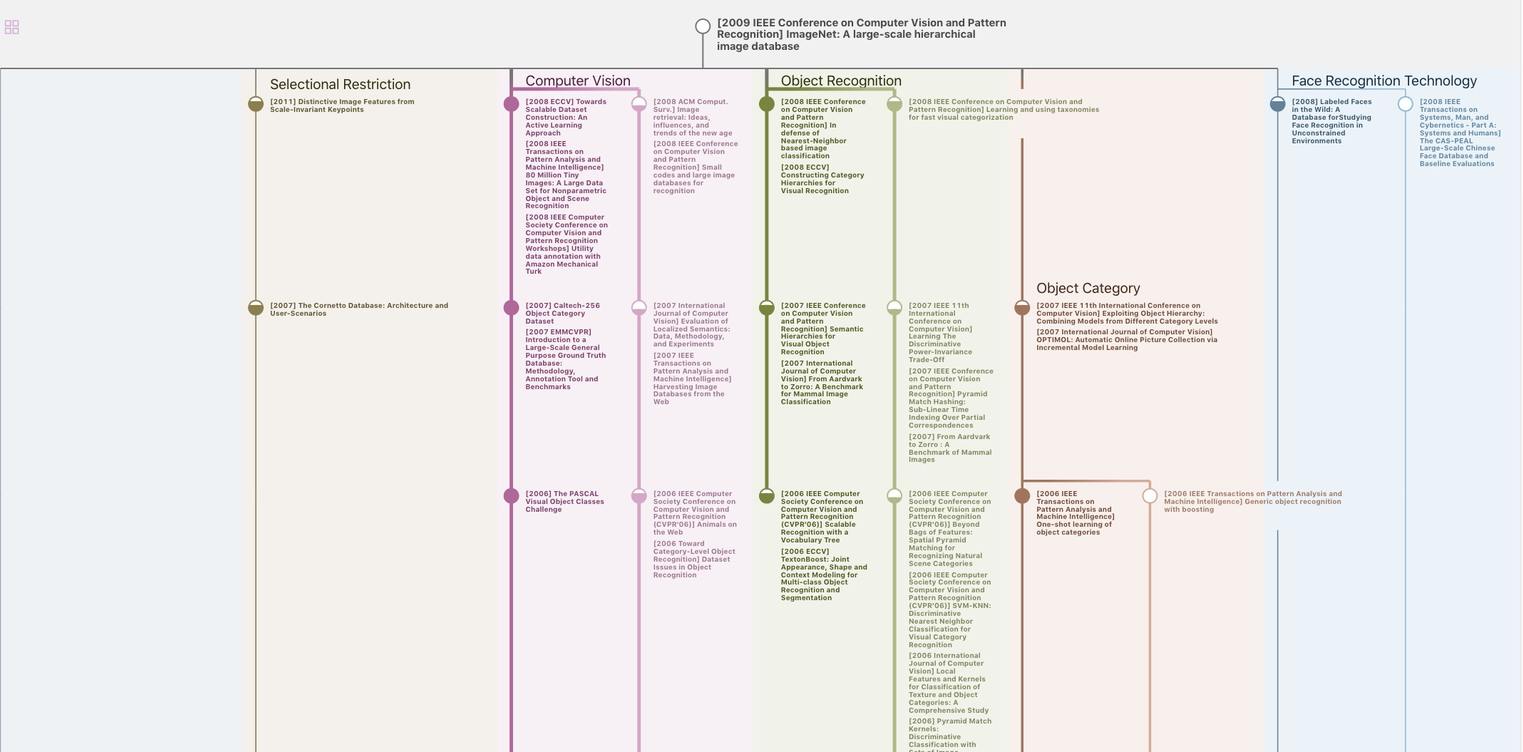
生成溯源树,研究论文发展脉络
Chat Paper
正在生成论文摘要