Noise2Quality: Non-Reference, Pixel-Wise Assessment of Low Dose CT Image Quality
MEDICAL IMAGING 2022: IMAGE PERCEPTION, OBSERVER PERFORMANCE, AND TECHNOLOGY ASSESSMENT(2022)
摘要
CT image quality is reliant on radiation dose, as low dose CT (LDCT) scans contain increased noise in images. This compromises the diagnostic performance on such scans. Therefore, it is desirable to perform Image Quality Assessment (IQA) prior to diagnostic use of CT scans. Often, image quality is assessed with full-reference methods, where a LDCT is algorithmically compared against its full dose counterpart. However due to health concerns, acquiring full dose CT scans is challenging and not desirable. As an alternative, non-reference IQA (NR-IQA) can be performed. Moreover, IQA at the pixel level is important, as most IQA methods only provide a global assessment, which means localized regions of interest cannot be specifically assessed. A solution for localized-IQA is to produce visually-interpretable quality maps. Deep learning methods could be employed by leveraging computer vision techniques, such as Self-Supervised learning (SSL). In this work, we propose Noise2Quality (N2Q)-a novel self-supervised, non-reference, pixel-wise image quality assessment model to predict IQA maps from LDCTs. Self-supervised dose level prediction as an auxiliary task further improves the model performance. Our experimental evaluation both qualitatively and quantitatively demonstrates the effectiveness of the model in accurately predicting IQA maps over various baselines.
更多查看译文
关键词
Computed Tomography,Self-Supervised Learning,Image Quality Assessment,Low-Dose CT
AI 理解论文
溯源树
样例
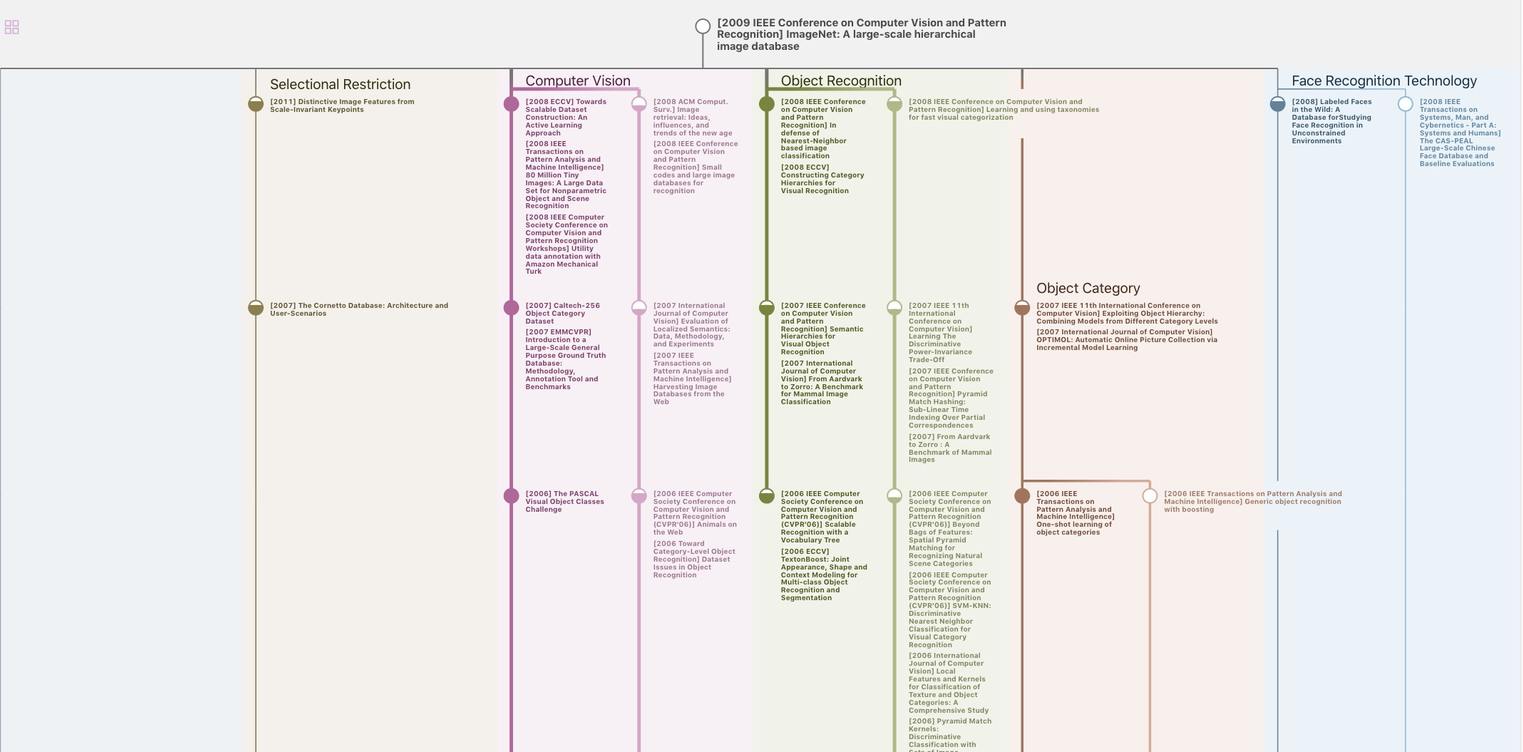
生成溯源树,研究论文发展脉络
Chat Paper
正在生成论文摘要