Deformable Registration of MRI to Intraoperative Cone-Beam CT of the Brain Using a Joint Synthesis and Registration Network
MEDICAL IMAGING 2022: IMAGE-GUIDED PROCEDURES, ROBOTIC INTERVENTIONS, AND MODELING(2022)
摘要
Purpose: Neuro-endoscopic surgery requires accurate targeting of deep-brain structures in the presence of deep-brain deformations ( up to 10 mm). We report a deep learning-based method to solve deformable MR-to-CBCT registration using a joint synthesis and registration (JSR) network. Method: The JSR network first encodes the MR and CBCT images into latent variables via MR and CBCT encoders, which are then decoded by two branches: image synthesis branches for MR-CT and CBCT-CT synthesis; and a registration branch for intra-modality registration in an intermediate (synthetic) CT domain. The two branches are jointly optimized, encouraging the encoders to extract features pertinent to both synthesis and registration. The algorithm was trained and tested on a dataset of 420 paired volumes presenting a wide range of simulated deformations. The JSR method was trained in a semi-supervised manner and evaluated in comparison to an alternative, state-of-the-art, inter-modality registration method (VoxelMorph). Results: The JSR method achieved Dice of 0.67 +/- 0.11, surface distance error (SD) of 0.47 +/- 0.26 mm, and target registration error (TRE) of 2.23 +/- 0.80 mm in a simulation study - each superior to the alternative methods considered in this work. Moreover, JSR maintained diffeomorphism and exhibited a fast runtime of 2.55 +/- 0.03 s. Conclusion: The JSR algorithm demonstrates accurate, near real-time deformable registration of preoperative MRI to intraoperative CBCT and is potentially suitable to intraoperative guidance of intracranial neurosurgery.
更多查看译文
关键词
Deformable Registration, Multimodal Registration, Deep Learning, Neurosurgery, Image Synthesis, Generative Adversarial Network
AI 理解论文
溯源树
样例
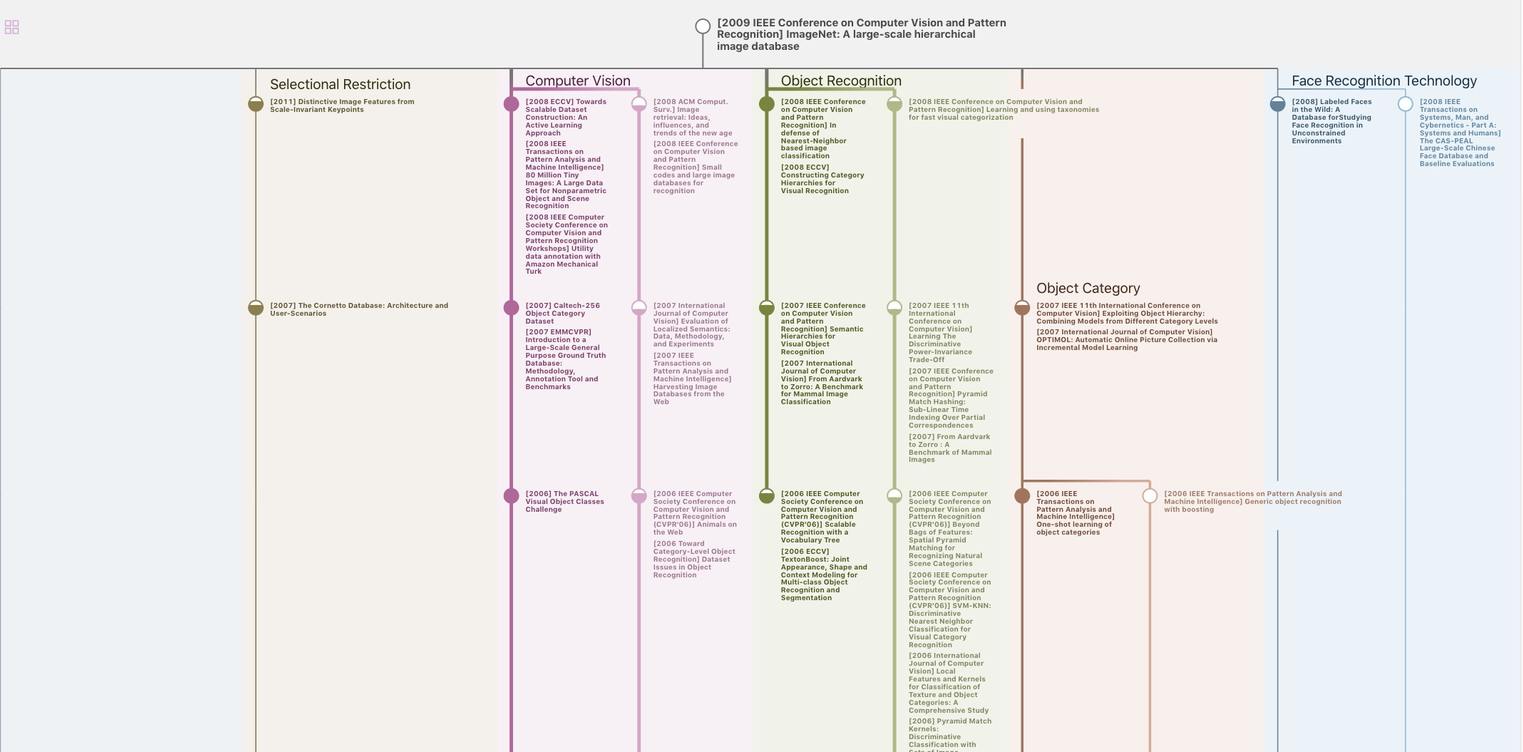
生成溯源树,研究论文发展脉络
Chat Paper
正在生成论文摘要