Communication-Efficient Federated Learning for Multi-Institutional Medical Image Classification
MEDICAL IMAGING 2022: IMAGING INFORMATICS FOR HEALTHCARE, RESEARCH, AND APPLICATIONS(2022)
摘要
Federated learning (FL) has emerged with increasing popularity in the medical image analysis field. In collaborative model training, it provides a privacy-preserving scheme by keeping data localized. In FL frameworks, instead of collecting data from clients, the server learns a global model by aggregating local training models from clients and broadcasts the updated model. However, in the situation where data is not identically and independently distributed (non-i.i.d), the model aggregation requires frequent message passing, which may face the communication bottleneck. In this paper, we propose a communication-efficient FL framework based on the adaptive server-client model transmission. The local model in the client will only be uploaded to the server under the conditions of (1) a probability threshold and (2) an informative model updating threshold. Our framework also tackles the data heterogeneity in federated networks by involving a proximal term. We evaluate our approach on a simulated multi-site medical image dataset for diabetic retinopathy (DR) rating. We demonstrate that our framework not only maintains the accuracy on non-i.i.d dataset but also provides a significant reduction in communication cost compared to other FL algorithms.
更多查看译文
关键词
Federated Learning, Medical Image Classification, Communication Efficiency
AI 理解论文
溯源树
样例
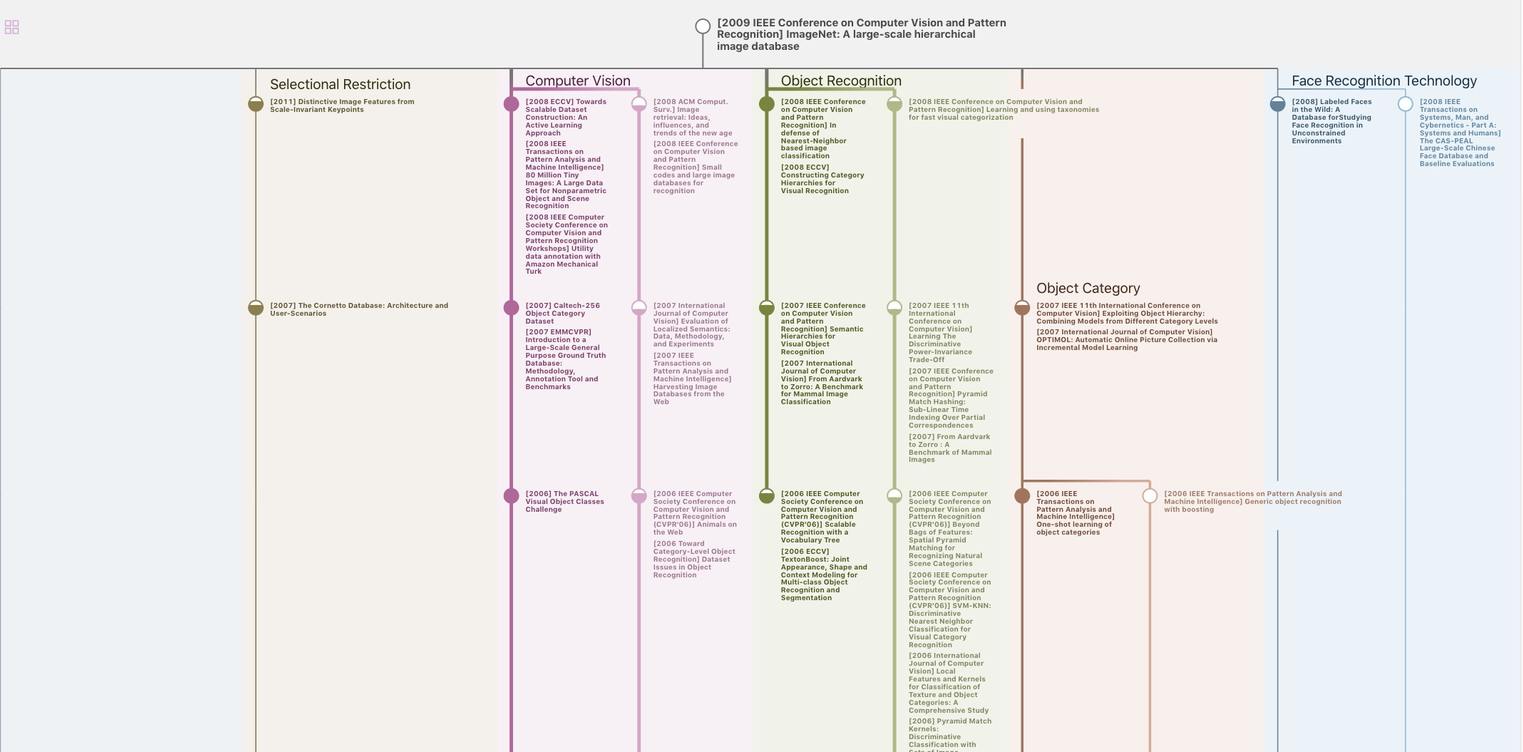
生成溯源树,研究论文发展脉络
Chat Paper
正在生成论文摘要