Automated myocardial segmentation of extra-cellular volume mapping cardiac magnetic resonance images using fully convolutional neural networks
MEDICAL IMAGING 2022: BIOMEDICAL APPLICATIONS IN MOLECULAR, STRUCTURAL, AND FUNCTIONAL IMAGING(2022)
摘要
Extra-cellular volume (ECV) mapping cardiac magnetic resonance (CMR) imaging allows for the characterization of expanded myocardial extracellular space, a common feature of myocardial fibrosis (MF). Quantification of MF is feasible using ECV mapping techniques; however, prior manual delineation of the endocardial and epicardial borders is required. In this study, we propose a method for automated myocardial delineation of ECV maps using convolutional neural networks (CNNs). We compare two methods based on the standard U-Net and the U-Net++ architectures using a five-fold cross validation on basal, mid, and apical short-axis ECV maps of the left ventricle (LV) in 73 patients with ischemic (n=38) or dilated (n=35) cardiomyopathies. The standard U-Net and U-Net++ -based architectures yielded DSC metrics of 87.61% and 87.89%, respectively, against manual contours derived by an expert. Precision and recall were reported >85% and relative error <12% for both CNNs. The U-Net++ architecture outperformed the standard U-Net on the order of 1-2% for all metrics. An inter-operator variability analysis was performed on a subset of myocardial contours derived by three operators. The inter-operator analysis demonstrated significant differences in the distribution of myocardial ECV values among three operators as per the Kruskal-Wallis H-test (average pair-wise P-value = 0.040), but operator differences failed to show significance against U-Net++ or standard U-Net (average pair-wise P-value 0.055 and 0.060, respectively). Correlation of global ECV improved for operators against U-Net++ (rho=0.88) and against standard U-Net (rho=0.877) compared to correlation of global ECV values between all operators (rho=0.828).
更多查看译文
关键词
ECV mapping, U-Net, U-Net plus, segmentation, CMR, convolutional neural networks
AI 理解论文
溯源树
样例
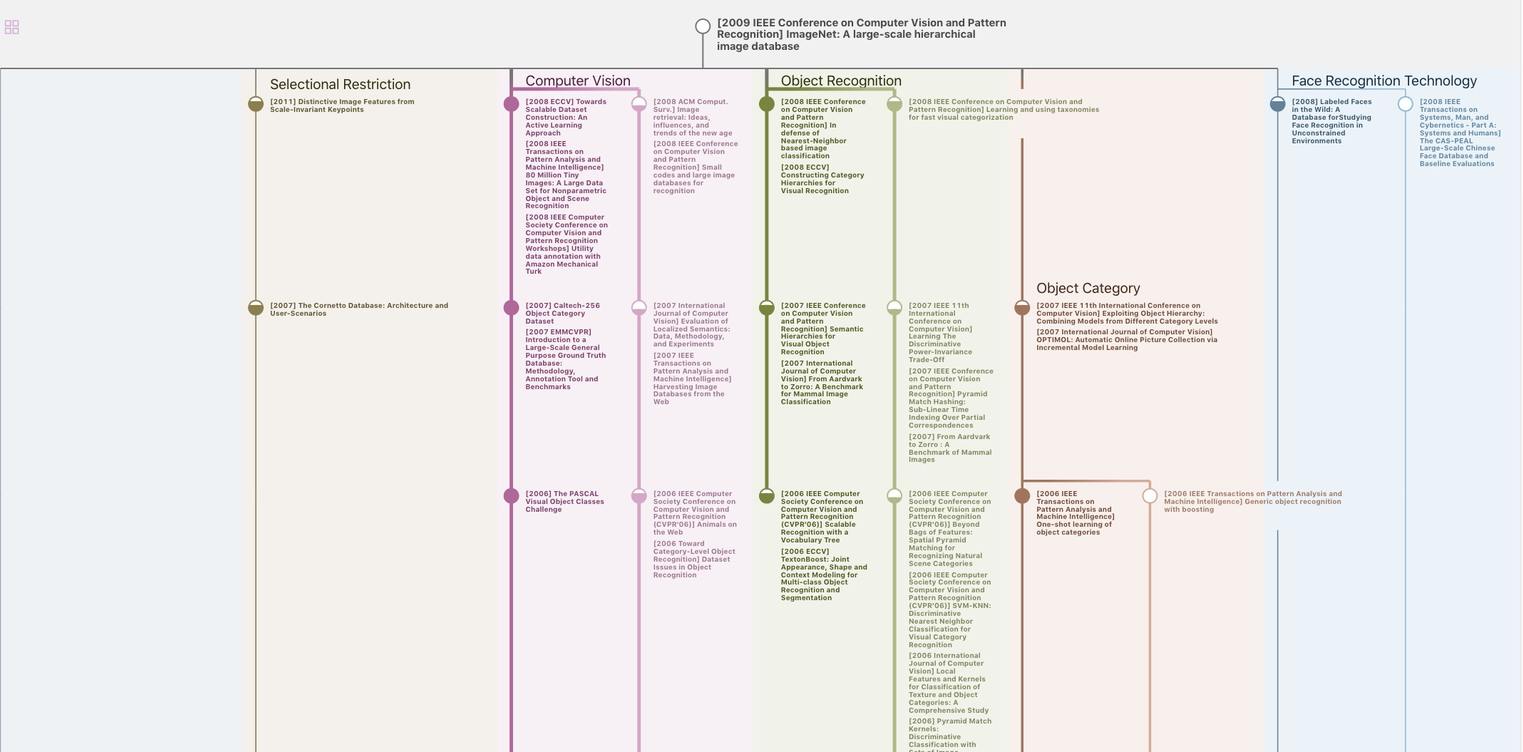
生成溯源树,研究论文发展脉络
Chat Paper
正在生成论文摘要