Learning to Utilize Curiosity: A New Approach of Automatic Curriculum Learning for Deep RL
MATHEMATICS(2022)
摘要
In recent years, reinforcement learning algorithms based on automatic curriculum learning have been increasingly applied to multi-agent system problems. However, in the sparse reward environment, the reinforcement learning agents get almost no feedback from the environment during the whole training process, which leads to a decrease in the convergence speed and learning efficiency of the curriculum reinforcement learning algorithm. Based on the automatic curriculum learning algorithm, this paper proposes a curriculum reinforcement learning method based on the curiosity model (CMCL). The method divides the curriculum sorting criteria into temporal-difference error and curiosity reward, uses the K-fold cross validation method to evaluate the difficulty priority of task samples, uses the Intrinsic Curiosity Module (ICM) to evaluate the curiosity priority of the task samples, and uses the curriculum factor to adjust the learning probability of the task samples. This study compares the CMCL algorithm with other baseline algorithms in cooperative-competitive environments, and the experimental simulation results show that the CMCL method can improve the training performance and robustness of multi-agent deep reinforcement learning algorithms.
更多查看译文
关键词
deep reinforcement learning, automatic curriculum learning, curiosity, sparse reward
AI 理解论文
溯源树
样例
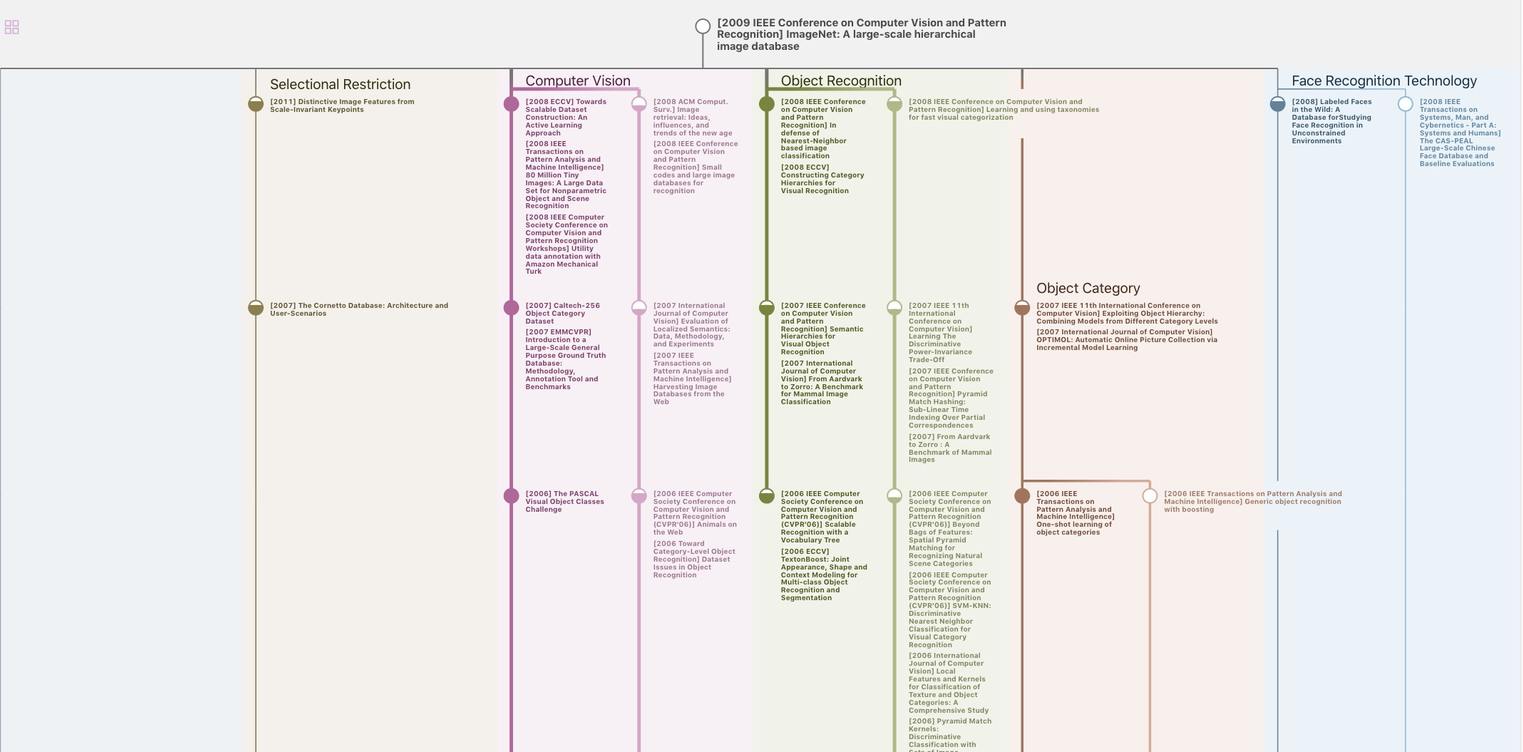
生成溯源树,研究论文发展脉络
Chat Paper
正在生成论文摘要