Self-supervised learning for fair recommender systems
Applied Soft Computing(2022)
摘要
Data-driven recommender algorithms are widely used in many systems, such as e-commerce recommender systems and movie recommendation systems. However, these systems could be affected by data bias, which leads to unfair recommendations for different groups of users. To address this problem, we propose a group rank fair recommender (GRFRec) method to mitigate the unfairness of recommender algorithms. We design a self-supervised learning framework to enhance user representation from both global and local views for fair results. In addition, adversarial learning is introduced to eliminate group-specific information and results in an unbiased user-item representation space, which avoids some groups suffering from unfair treatment in recommender results. Experimental results on three real-world datasets demonstrate that GRFRec can not only significantly improve fairness but also attain better results on the recommendation accuracy.
更多查看译文
关键词
Fairness representation,Recommender systems,Self-supervised learning
AI 理解论文
溯源树
样例
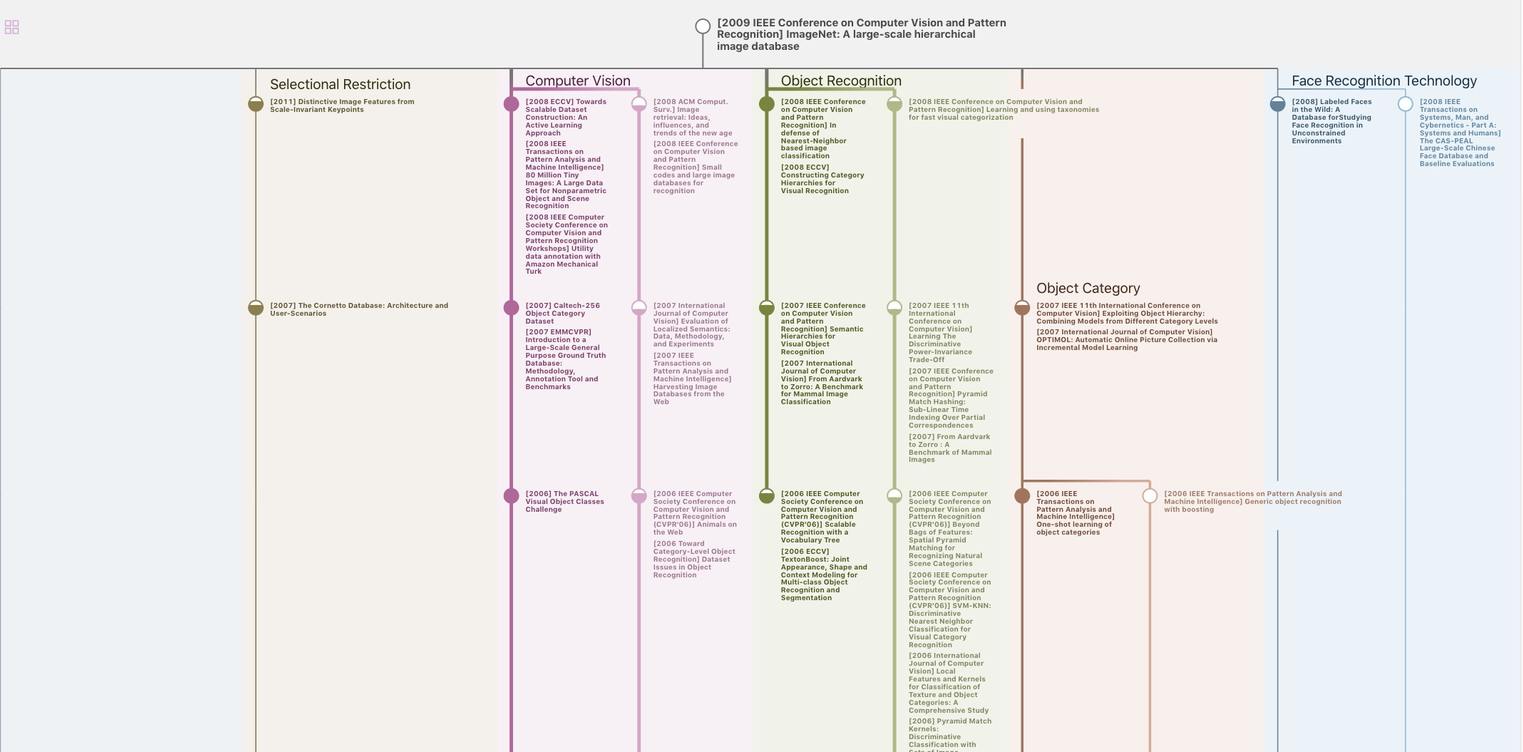
生成溯源树,研究论文发展脉络
Chat Paper
正在生成论文摘要